Contextual Colorization and Denoising for Low-Light Ultra High Resolution Sequences
ICIP(2021)
摘要
Low-light image sequences generally suffer from spatiotemporal incoherent noise, flicker and blurring of moving objects. These artefacts significantly reduce visual quality and, in most cases, post-processing is needed in order to generate acceptable quality. Most state-of-the-art enhancement methods based on machine learning require ground truth data but this is not usually available for naturally captured low light sequences. We tackle these problems with an unpaired learning method that offers simultaneous colorization and denoising. Our approach is an adaptation of the CycleGAN structure. To overcome the excessive memory limitations associated with ultra high resolution content, we propose a multiscale patch-based framework, capturing both local and contextual features. Additionally, an adaptive temporal smoothing technique is employed to remove flickering artefacts. Experimental results show that our method outperforms existing approaches in terms of subjective quality and that it is robust to variations in brightness levels and noise.
更多查看译文
关键词
colorization,denoising,GAN
AI 理解论文
溯源树
样例
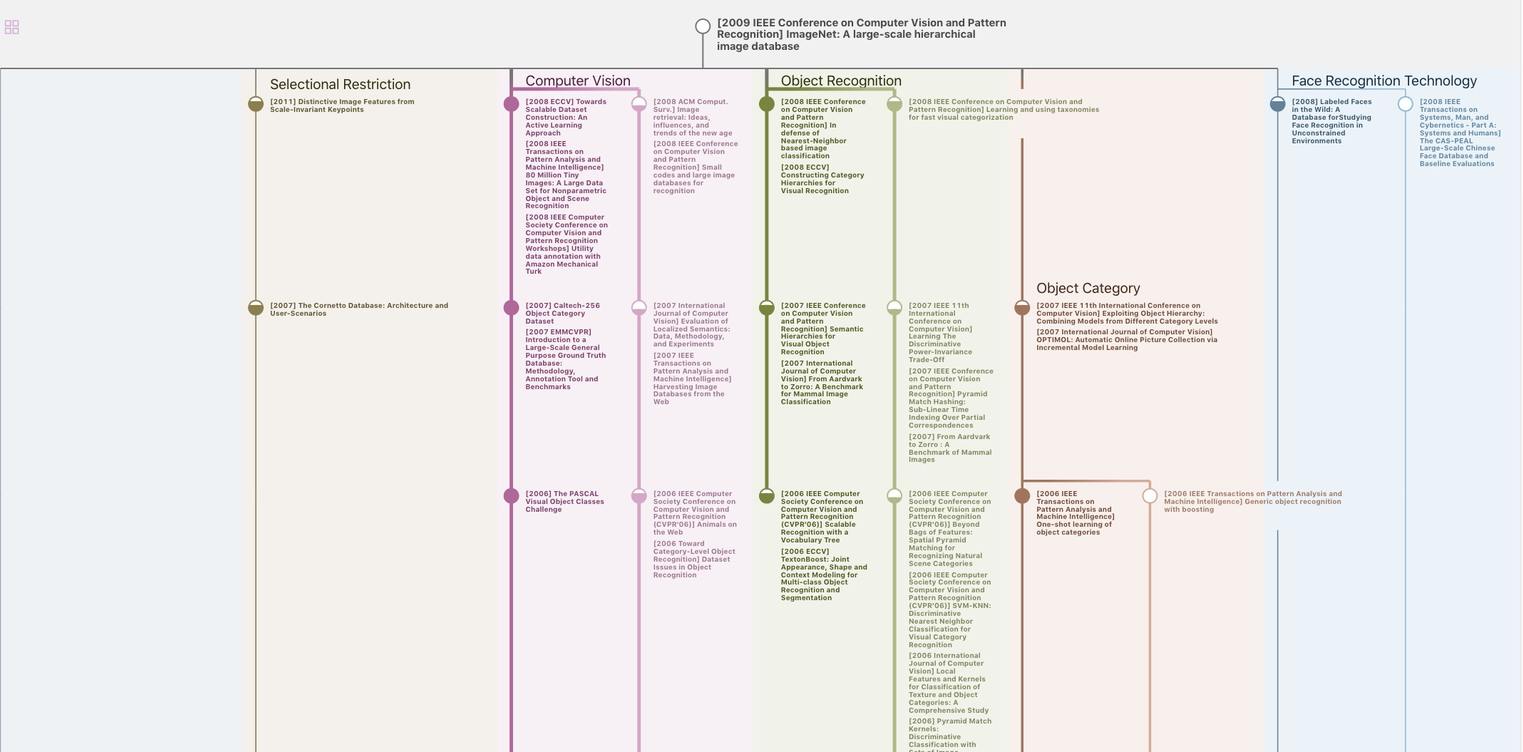
生成溯源树,研究论文发展脉络
Chat Paper
正在生成论文摘要