Genetic Programming Model Regularization
Studies in Computational Intelligence(2016)
摘要
We propose a tool for controlling the complexity of Genetic Programming models. The tool is supported by the theory of Vapnik-Chervonekis dimension (VCD) and is combined with a novel representation of models named straight line program. Experimental results, implemented on conventional algebraic structures (such as polynomials), show that the empirical risk, penalized by suitable upper bounds for the Vapnik-Chervonenkis dimension, gives a generalization error smaller than the use of statistical conventional techniques such as Bayesian or Akaike information criteria.
更多查看译文
关键词
Genetic Programming,Straight Line Program,Pfaffian Operator,Symbolic Regression
AI 理解论文
溯源树
样例
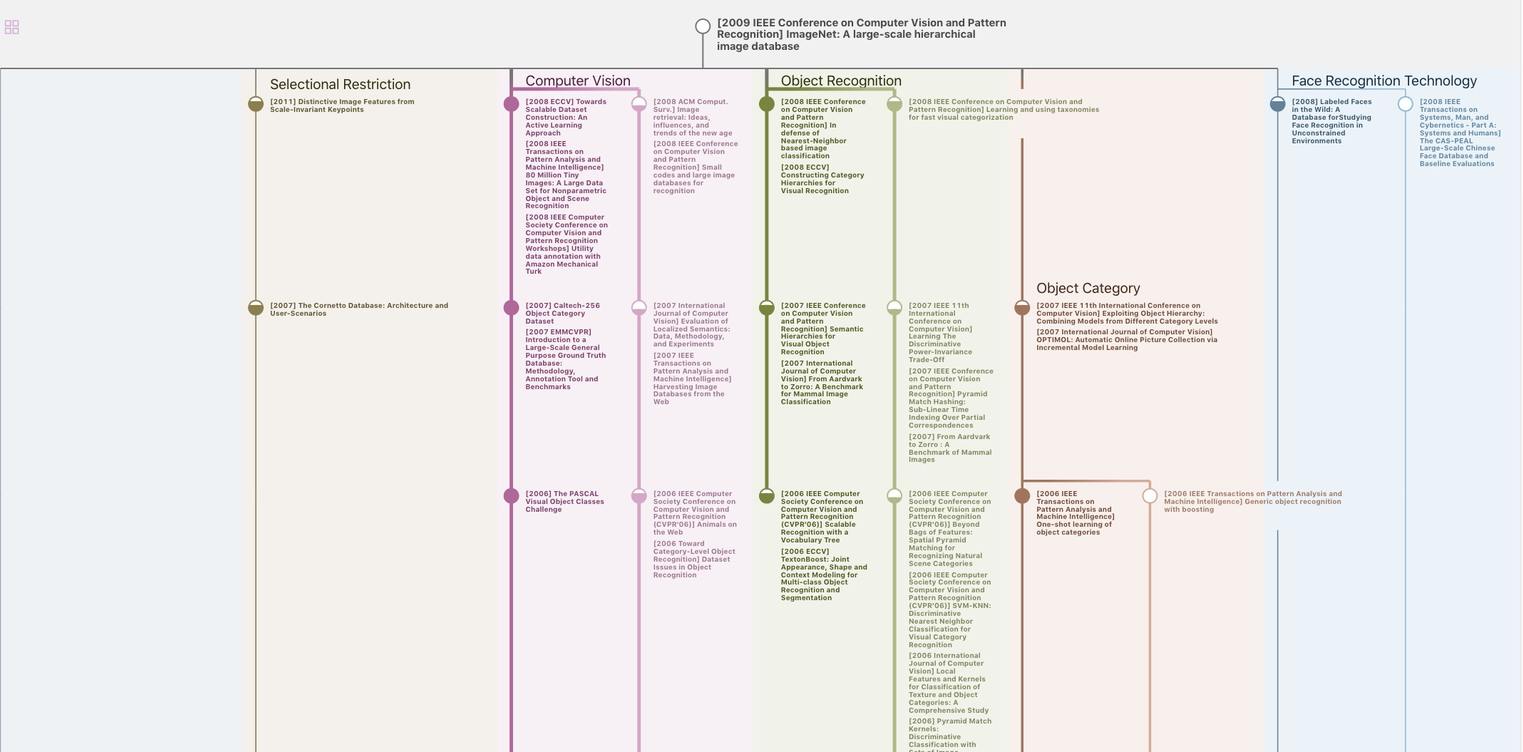
生成溯源树,研究论文发展脉络
Chat Paper
正在生成论文摘要