Measurement error in small area estimation: Functional versus structural versus naive models
SURVEY METHODOLOGY(2019)
摘要
Small area estimation using area-level models can sometimes benefit from covariates that are observed subject to random errors, such as covariates that are themselves estimates drawn from another survey. Given estimates of the variances of these measurement (sampling) errors for each small area, one can account for the uncertainty in such covariates using measurement error models (e.g., Ybarra and Lohr, 2008). Two types of area-level measurement error models have been examined in the small area estimation literature. The functional measurement error model assumes that the underlying true values of the covariates with measurement error are fixed but unknown quantities. The structural measurement error model assumes that these true values follow a model, leading to a multivariate model for the covariates observed with error and the original dependent variable. We compare and contrast these two models with the alternative of simply ignoring measurement error when it is present (naive model), exploring the consequences for prediction mean squared errors of use of an incorrect model under different underlying assumptions about the true model. Comparisons done using analytic formulas for the mean squared errors assuming model parameters are known yield some surprising results. We also illustrate results with a model fitted to data from the U.S. Census Bureau's Small Area Income and Poverty Estimates (SAIPE) Program.
更多查看译文
关键词
Sample survey,Area level model,Covariate,Prediction
AI 理解论文
溯源树
样例
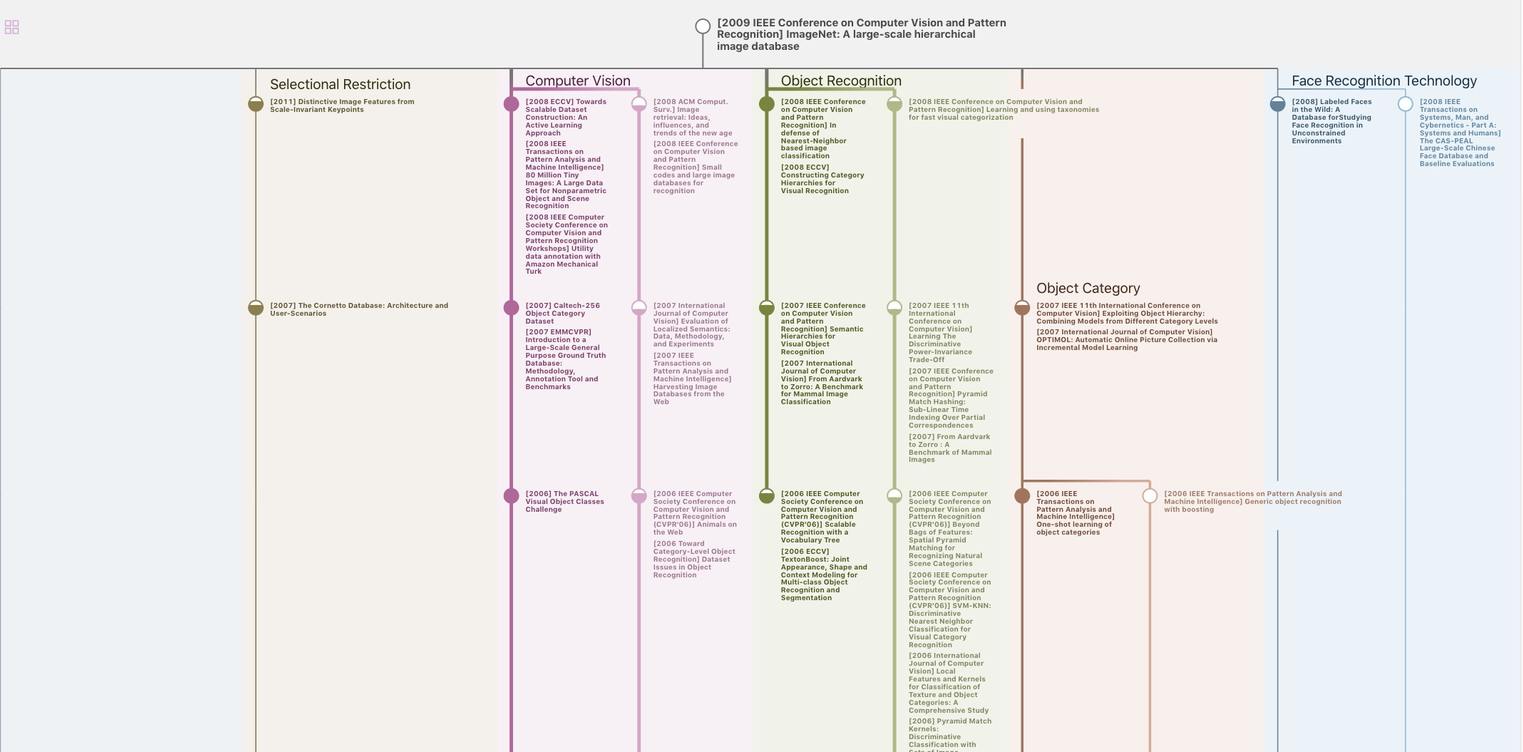
生成溯源树,研究论文发展脉络
Chat Paper
正在生成论文摘要