Survival Risk Prediction Of Esophageal Cancer Based On Self-Organizing Maps Clustering And Support Vector Machine Ensembles
IEEE ACCESS(2020)
摘要
This article provides a method based on self-organizing maps (SOM) neural network clustering and support vector machine (SVM) ensembles to predict the survival risk levels of esophageal cancer. Nine blood indexes related to patient survival are found by using SOM clustering method. Two critical thresholds for survival are found by plotting the receiver operating characteristic (ROC) curve twice, and the lifetime is divided into three risk levels. Using the SVM method, patients' risk levels are predicted and assessed. Four kernel functions of SVM are compared, and the prediction effect of RBF kernel function is better than other kernel functions. The parameters of SVM are optimized by using genetic algorithm (GA), particle swarm algorithm (PSO) and artificial bee colony (ABC) algorithm. Experimental results show that the prediction accuracies are improved by using optimization algorithms. After comparison, ABC-SVM has better prediction results than GA-SVM and PSO-SVM with a high prediction rate and fast running time.
更多查看译文
关键词
Artificial bee colony, genetic algorithm, particle swarm optimization, self-organizing maps, support vector machine
AI 理解论文
溯源树
样例
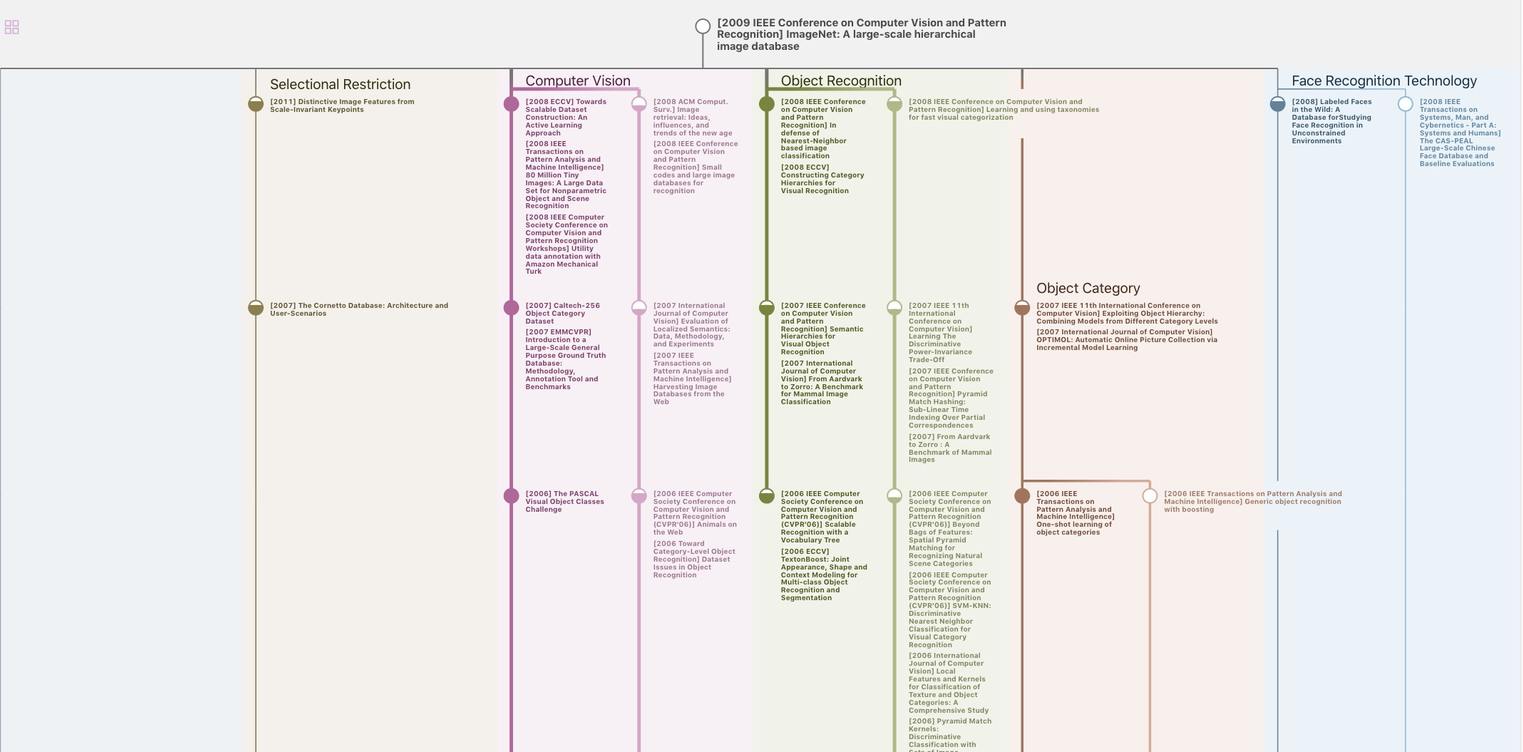
生成溯源树,研究论文发展脉络
Chat Paper
正在生成论文摘要