Efficient Parameter-Free Adaptive Multi-Modal Hashing
IEEE SIGNAL PROCESSING LETTERS(2020)
摘要
Unsupervised multi-modal hashing has recently attracted broad attention in research area of large-scale multimedia retrieval for its low storage cost, high retrieval speed, and independence on semantic labels. However, the model learning process of existing methods still suffer from the problem of low efficiency: 1) Many existing methods measure the contributions of different modalities using fixed modality weights. In order to avoid over-fitting, they need an inefficient hyper-parameter adjustment process. 2) Most existing methods adopt inefficient optimization strategies to learn hash codes. In this letter, we propose an unsupervised Efficient Parameter-free Adaptive Multi-modal Hashing (EPAMH) model to adaptively capture the modality variations and preserve the discriminative semantics of multi-modal features into the binary hash codes. Moreover, we directly learn the binary codes with simple and efficient operations, which prevents the relaxing quantization errors and improves the model learning efficiency. Experiments prove the superior performance of EPAMH on three public multimedia retrieval datasets. Our source codes and testing datasets can be obtained at https://github.com/ChaoqunZheng/EPAMH.
更多查看译文
关键词
Multi-modal hashing, parameter-free, fast discrete optimization, adaptive weights
AI 理解论文
溯源树
样例
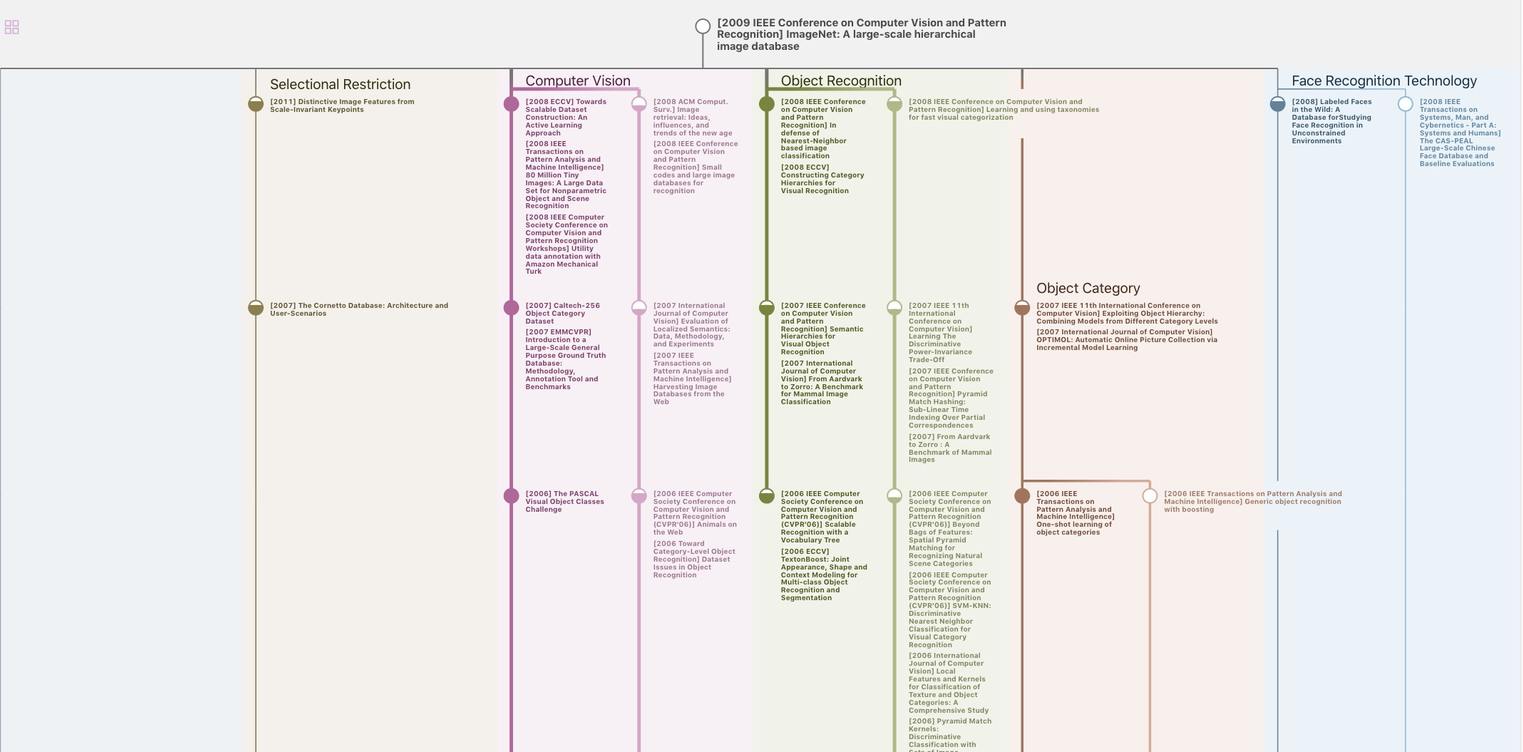
生成溯源树,研究论文发展脉络
Chat Paper
正在生成论文摘要