Calibrating Human-AI Collaboration: Impact of Risk, Ambiguity and Transparency on Algorithmic Bias
CD-MAKE(2020)
摘要
Transparent Machine Learning (ML) is often argued to increase trust into predictions of algorithms however the growth of new interpretability approaches is not accompanied by a growth in studies investigating how interaction of humans and Artificial Intelligence (AI) systems benefits from transparency. The right level of transparency can increase trust in an AI system, while inappropriate levels of transparency can lead to algorithmic bias. In this study we demonstrate that depending on certain personality traits, humans exhibit different susceptibilities for algorithmic bias. Our main finding is that susceptibility to algorithmic bias significantly depends on annotators’ affinity to risk. These findings help to shed light on the previously underrepresented role of human personality in human-AI interaction. We believe that taking these aspects into account when building transparent AI systems can help to ensure more responsible usage of AI systems.
更多查看译文
关键词
Transparent AI,Machine learning,HCI,Risk affinity
AI 理解论文
溯源树
样例
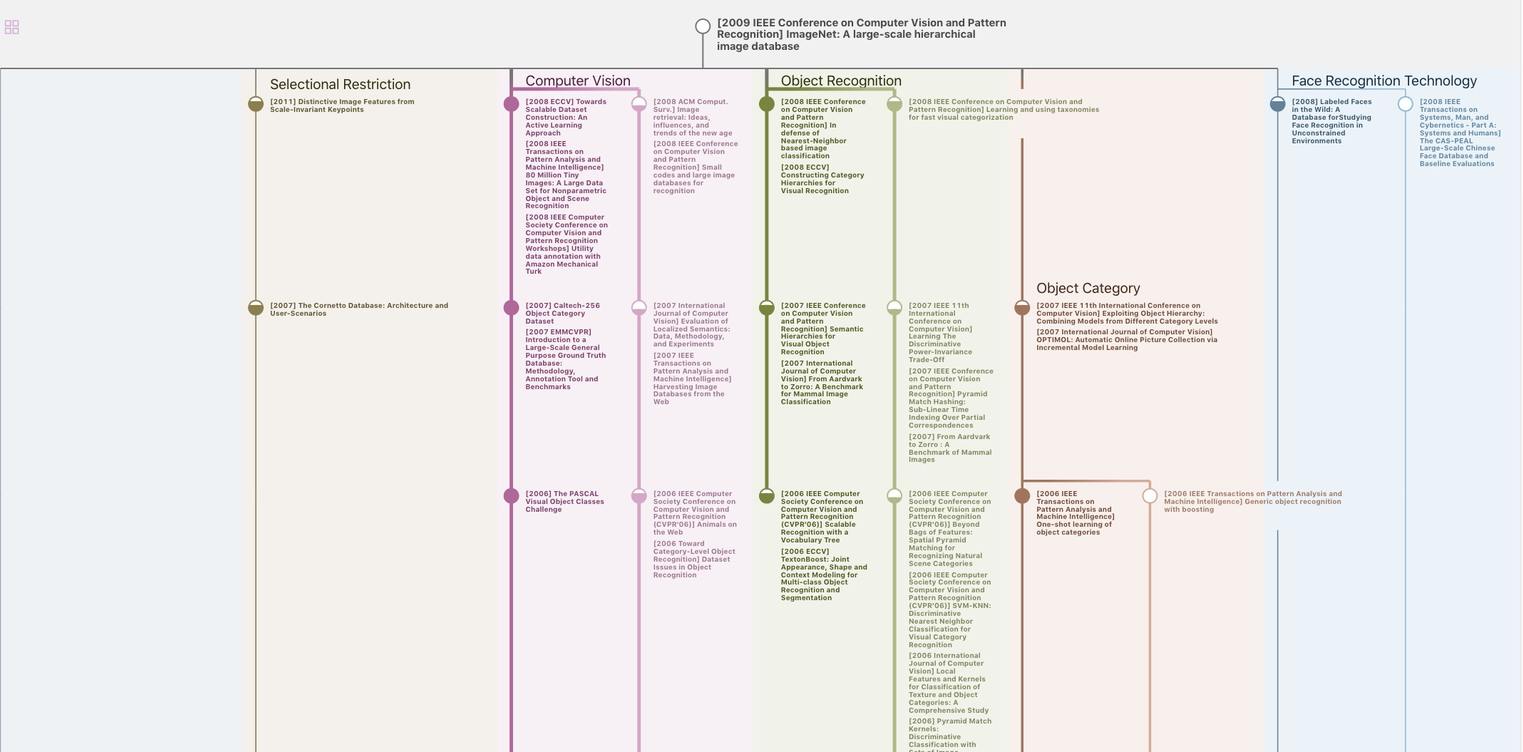
生成溯源树,研究论文发展脉络
Chat Paper
正在生成论文摘要