A Comparative Study Of Inversion Optimization Algorithms For Underground Metal Target Detection
IEEE ACCESS(2020)
摘要
Underground metal target detection refers to estimating the properties of underground metal targets based on a set of observed data. Electromagnetic induction (EMI) method and the least-squares inversion provide the data acquisition method and the parameter estimation method for the detection, respectively. As an important part of least-squares inversion, optimization algorithms directly affect the efficiency of the least-squares inversion. To improve the efficiency of underground metal target detection, it is necessary to compare the performance of different optimization algorithms. In this paper, we analyzed the characteristic of the complex EMI forward model using sensitivity analysis and inverse sensitivity analysis at first. Then, the EMI forward model and least squares were used to build the objective function. The estimation error, run time and number of iterations of six numerical optimization algorithms were compared under different signal-to-noise ratios (SNRs) and under different data acquisition spacing. The algorithms include gradient descent, steepest descent, Newton's method, Broyden-Fletcher-Goldfarb-Shanno (BFGS) algorithm, conjugate gradient method and Levenberg-Marquardt (LM) algorithm. A simulation platform was established to generate observed data and compare the optimization algorithms. The results of algorithm comparison showed that the BFGS, conjugate gradient and LM algorithm can efficiently and accurately estimate the properties of underground metal targets and the LM algorithm has the shortest run time and the least number of iterations. Finally, we carefully analyzed the time consumption of each optimization algorithm. The results show that the calculation of the gradient and step length selection greatly affect the efficiency of the optimization algorithms.
更多查看译文
关键词
Electromagnetic induction (EMI),least-squares inversion,optimization algorithm,sensitivity analysis,underground metal target detection
AI 理解论文
溯源树
样例
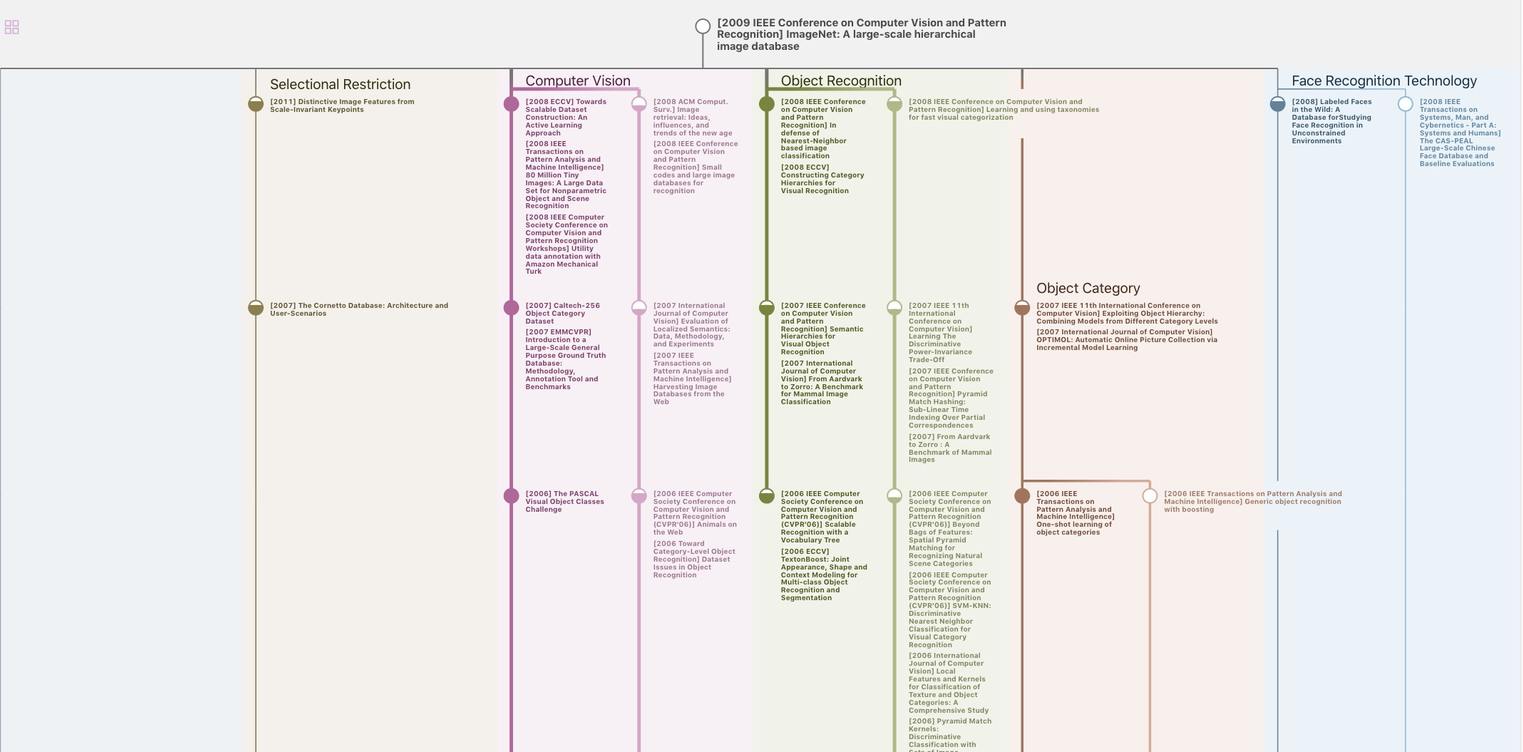
生成溯源树,研究论文发展脉络
Chat Paper
正在生成论文摘要