An adversarial semi-supervised approach for action recognition from pose information
Neural Comput. Appl.(2020)
摘要
The collection of video data for action recognition is very susceptible to measurement bias; the equipment used, camera angle and environmental conditions are all factors that majorly affect the distribution of the collected dataset. Inevitably, training a classifier that can successfully generalize to new data becomes a very hard problem, since it is impossible to gather general enough training sets. Recent approaches in the literature attempt to solve this problem by augmenting a given training set, with synthetic data, so as to better represent the global distribution of the covariates. However, these approaches are limited because they essentially involve hand-crafted data synthesizers, which are typically hard to implement and problem specific. In this work, we propose a different approach to tackling the above issues, which relies on the combination of two techniques: pose extraction, and domain adaptation as a means to improve the generalization capabilities of classifiers. We show that adapted skeletal representations can be retrieved automatically in a semi-supervised setting and these help to generalize classifiers to new forms of measurement bias. We empirically validate our approach for generalizing across different camera angles.
更多查看译文
关键词
Action recognition,Domain adaptation,Adversarial neural networks
AI 理解论文
溯源树
样例
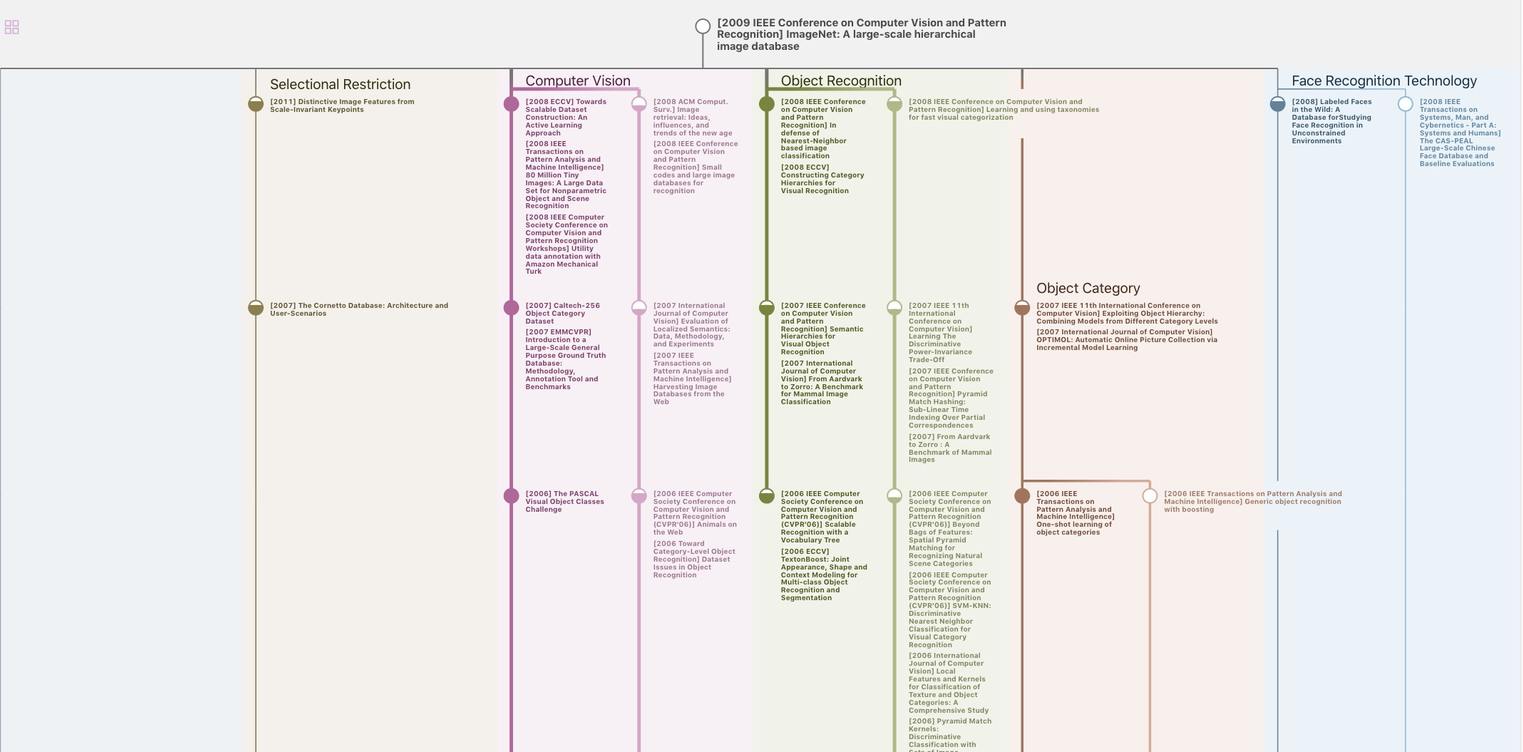
生成溯源树,研究论文发展脉络
Chat Paper
正在生成论文摘要