Incorporating Biterm Correlation Knowledge into Topic Modeling for Short Texts
The Computer Journal(2022)
摘要
The prevalence of short texts on the Web has made mining the latent topic structures of short texts a critical and fundamental task for many applications. However, due to the lack of word co-occurrence information induced by the content sparsity of short texts, it is challenging for traditional topic models like latent Dirichlet allocation (LDA) to extract coherent topic structures on short texts. Incorporating external semantic knowledge into the topic modeling process is an effective strategy to improve the coherence of inferred topics. In this paper, we develop a novel topic model-called biterm correlation knowledge-based topic model (BCK-TM)-to infer latent topics from short texts. Specifically, the proposed model mines biterm correlation knowledge automatically based on recent progress in word embedding, which can represent semantic information of words in a continuous vector space. To incorporate external knowledge, a knowledge incorporation mechanism is designed over the latent topic layer to regularize the topic assignment of each biterm during the topic sampling process. Experimental results on three public benchmark datasets illustrate the superior performance of the proposed approach over several state-of-the-art baseline models.
更多查看译文
关键词
topic model, short texts, biterm correlation knowledge, word embedding, Gibbs sampling
AI 理解论文
溯源树
样例
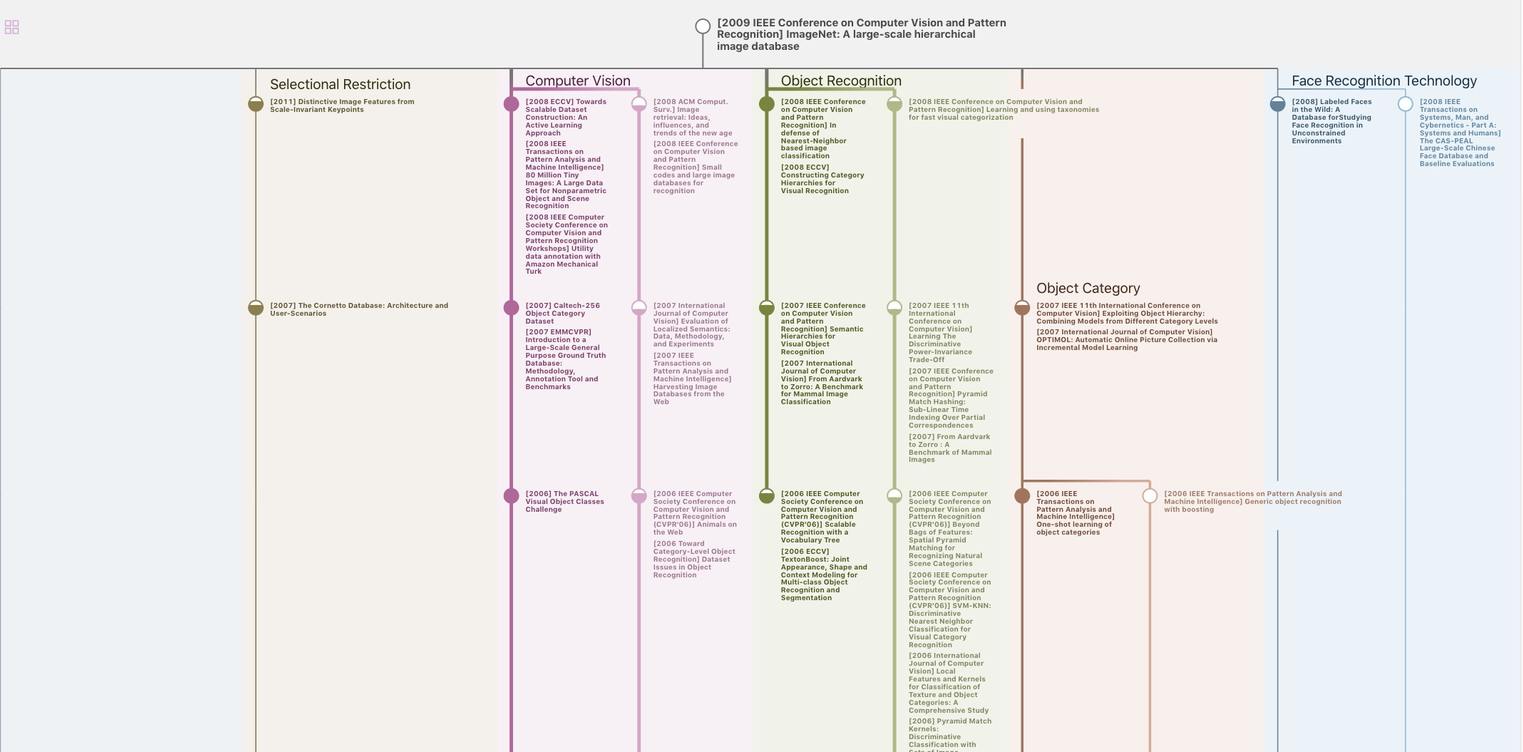
生成溯源树,研究论文发展脉络
Chat Paper
正在生成论文摘要