Nonlocal Regularized Cnn For Image Segmentation
INVERSE PROBLEMS AND IMAGING(2020)
摘要
Non-local dependency is a very important prior for many image segmentation tasks. Generally, convolutional operations are building blocks that process one local neighborhood at a time which means the convolutional neural networks(CNNs) usually do not explicitly make use of the non-local prior on image segmentation tasks. Though the pooling and dilated convolution techniques can enlarge the receptive field to use some nonlocal information during the feature extracting step, there is no nonlocal priori for feature classification step in the current CNNs' architectures. In this paper, we present a nonlocal total variation (TV) regularized softmax activation function method for semantic image segmentation tasks. The proposed method can be integrated into the architecture of CNNs. To handle the difficulty of back-propagation for CNNs due to the non-smoothness of nonlocal TV, we develop a primal-dual hybrid gradient method to realize the back-propagation of nonlocal TV in CNNs. Experimental evaluations of the non-local TV regularized softmax layer on a series of image segmentation datasets showcase its good performance. Many CNNs can benefit from our proposed method on image segmentation tasks.
更多查看译文
关键词
CNN, nonlocal TV, regularization, duality, image segmentation
AI 理解论文
溯源树
样例
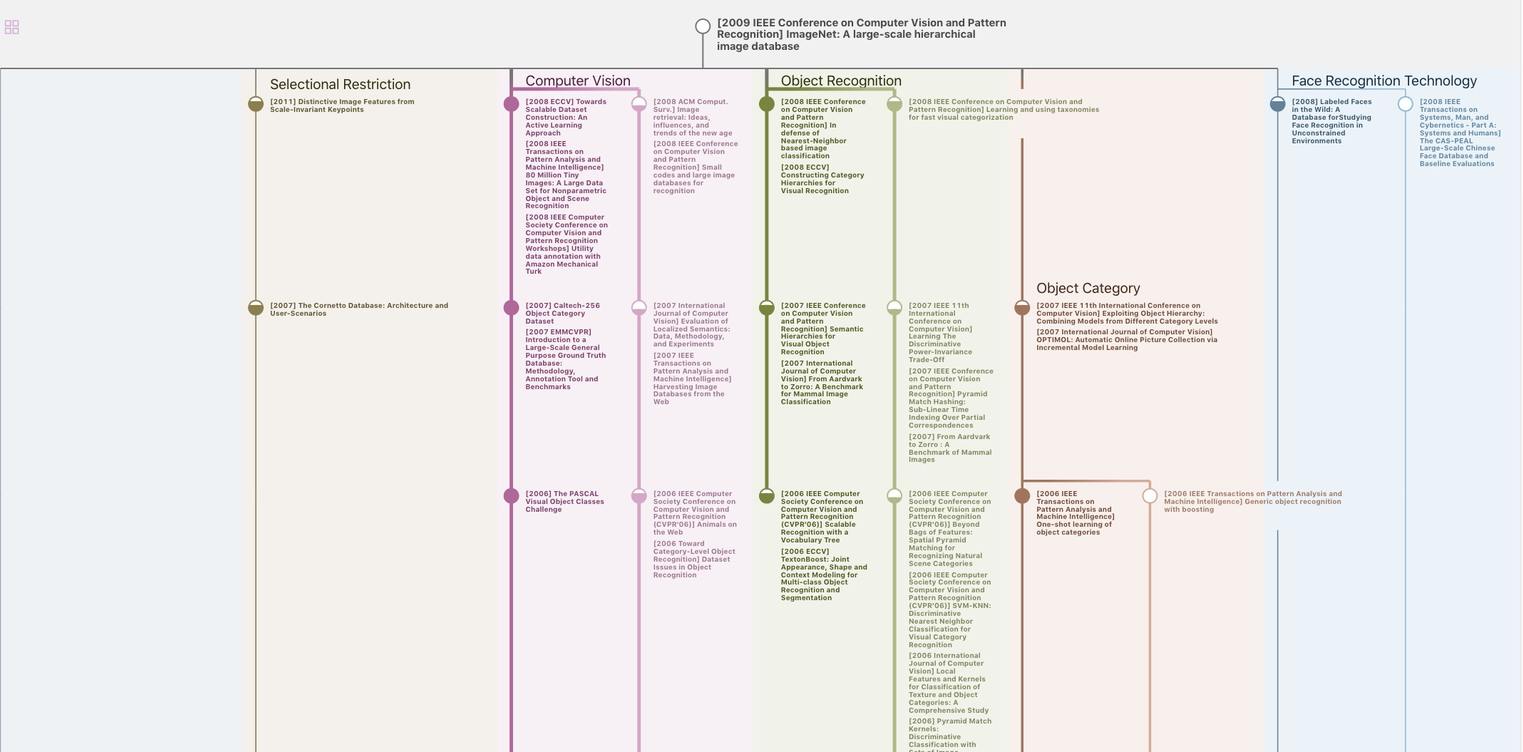
生成溯源树,研究论文发展脉络
Chat Paper
正在生成论文摘要