Large-Scale Least Squares Twin SVMs
ACM Transactions on Internet Technology(2021)
摘要
AbstractIn the last decade, twin support vector machine (TWSVM) classifiers have achieved considerable emphasis on pattern classification tasks. However, the TWSVM formulation still suffers from the following two shortcomings: (1) TWSVM deals with the inverse matrix calculation in the Wolfe-dual problems, which is intractable for large-scale datasets with numerous features and samples, and (2) TWSVM minimizes the empirical risk instead of the structural risk in its formulation. With the advent of huge amounts of data today, these disadvantages render TWSVM an ineffective choice for pattern classification tasks. In this article, we propose an efficient large-scale least squares twin support vector machine (LS-LSTSVM) for pattern classification that rectifies all the aforementioned shortcomings. The proposed LS-LSTSVM introduces different Lagrangian functions to eliminate the need for calculating inverse matrices. The proposed LS-LSTSVM also does not employ kernel-generated surfaces for the non-linear case, and thus uses the kernel trick directly. This ensures that the proposed LS-LSTSVM model is superior to the original TWSVM and LSTSVM. Lastly, the structural risk is minimized in LS-LSTSVM. This exhibits the essence of statistical learning theory, and consequently, classification accuracy on datasets can be improved due to this change. The proposed LS-LSTSVM is solved using the sequential minimal optimization (SMO) technique, making it more suitable for large-scale problems. We further proved the convergence of the proposed LS-LSTSVM. Exhaustive experiments on several real-world benchmarks and NDC-based large-scale datasets demonstrate that the proposed LS-LSTSVM is feasible for large datasets and, in most cases, performed better than existing algorithms.
更多查看译文
关键词
Machine learning, support vector machines (SVMs), large scale SVMs, least squares twin SVM
AI 理解论文
溯源树
样例
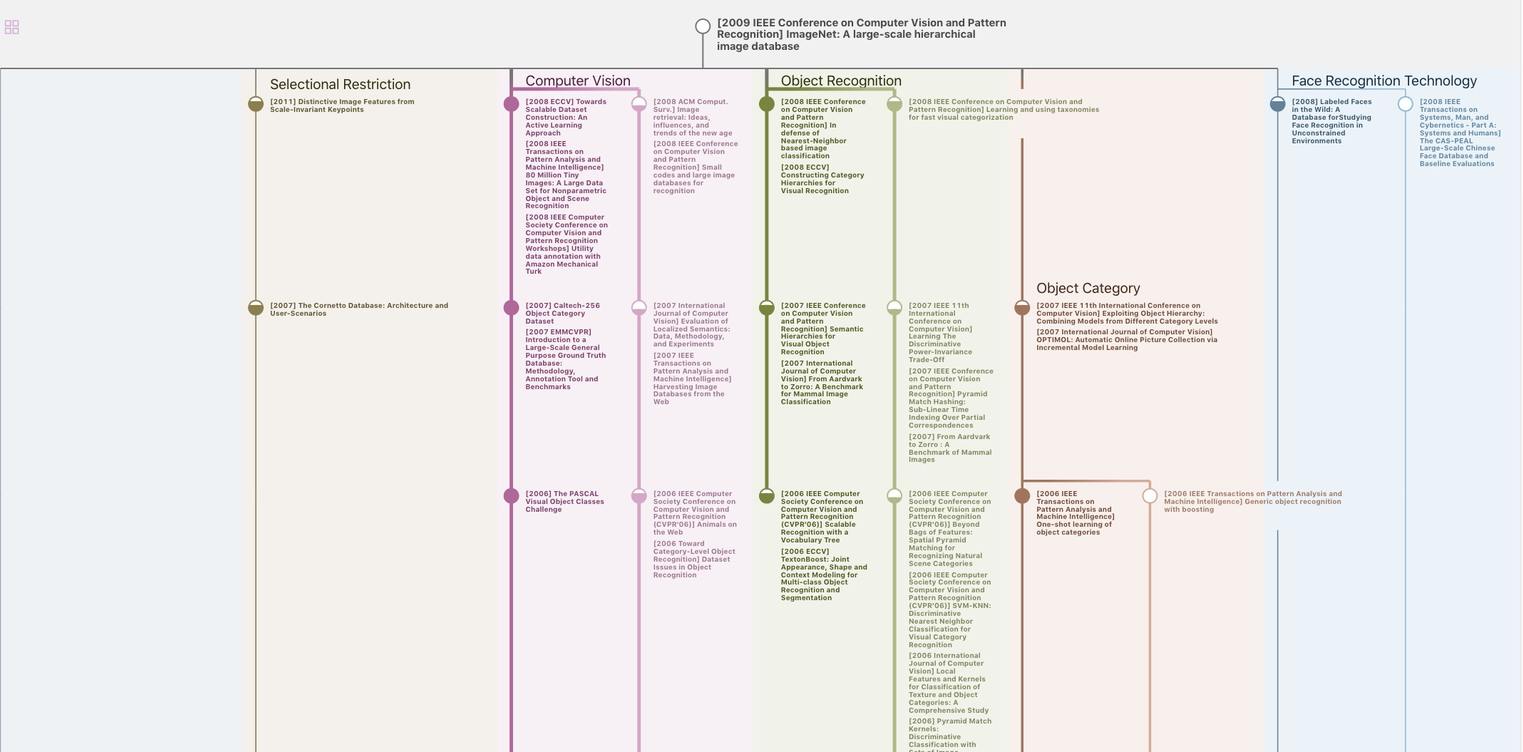
生成溯源树,研究论文发展脉络
Chat Paper
正在生成论文摘要