Large Factor Image Super-Resolution With Cascaded Convolutional Neural Networks
IEEE TRANSACTIONS ON MULTIMEDIA(2021)
摘要
Recently, convolutional neural networks (CNNs) have attracted considerable attention in single image super-resolution (SISR) and have enabled great performance improvements. However, most of the existing methods super-resolve input images to the desired size with an interpolation operation during the beginning stage, which brings about heavy aliasing artifacts and high computational costs. Especially for large upsampling factors (e.g., 8x), it remains a challenge to restore high-quality results for deeply degraded images. To tackle this problem, we propose a cascaded super-resolution convolutional neural network (CSRCNN), which takes a single low-resolution (LR) image as an input and reconstructs high-resolution (HR) images in a progressive way. At each cascaded level, to help converge and improve the accuracy, a novel U-net based block with backprojection is first introduced, which exploits the mutual relation between HR and LR feature spaces. A refined block following the U-net block is also used to reconstruct the realistic texture details. In addition, we naturally utilize the strategy of curriculum learning, organizing the learning process from easy (small factors) to hard (large factors). Comprehensive experiments on benchmark datasets demonstrate that the proposed network achieves superior results compared with those of other state-of-the-art methods, particularly with the 8x upsampling factor.
更多查看译文
关键词
Convolution, Convolutional neural networks, Image reconstruction, Computer architecture, Computational efficiency, Cascaded architecture, convolutional neural networks, image super-resolution
AI 理解论文
溯源树
样例
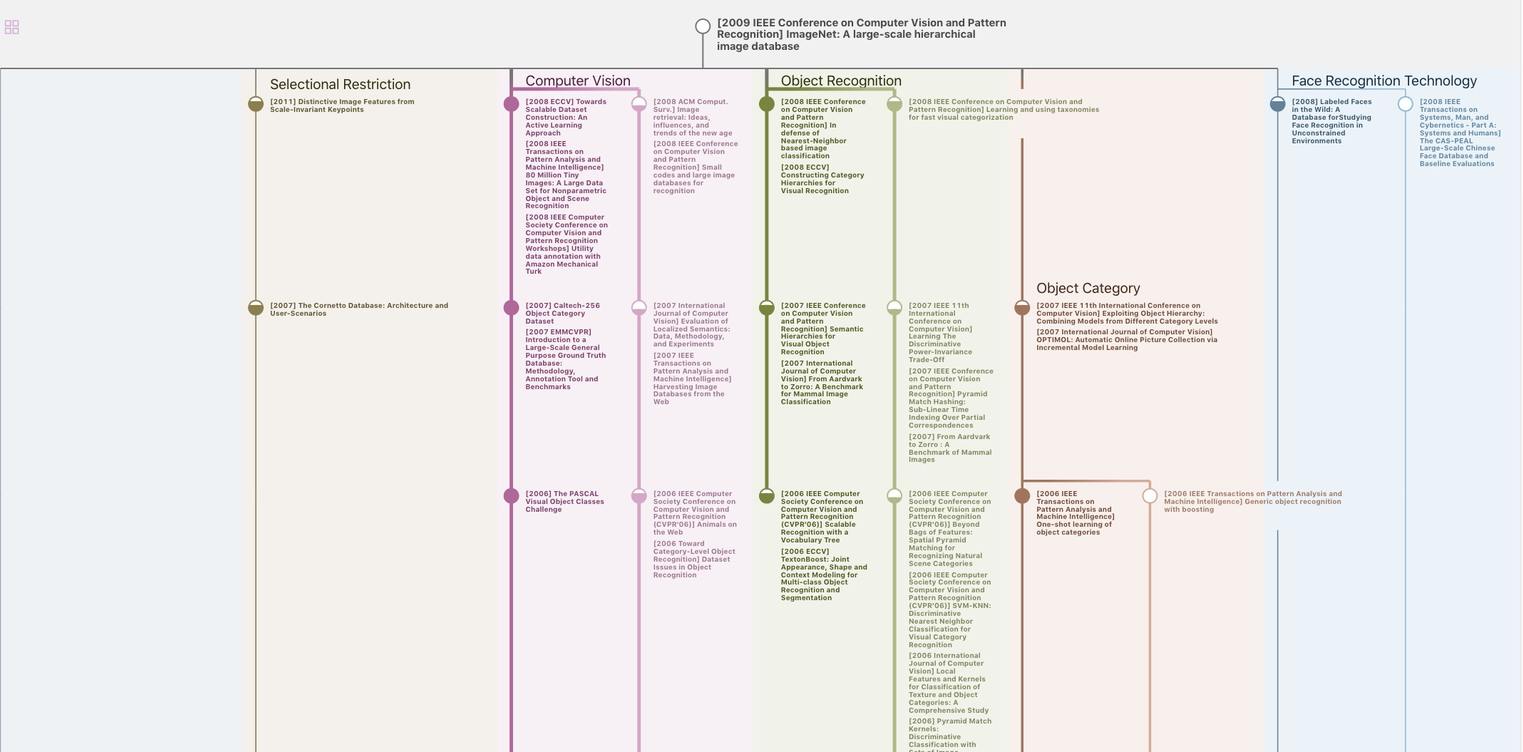
生成溯源树,研究论文发展脉络
Chat Paper
正在生成论文摘要