Automated data-driven prediction on aircraft Estimated Time of Arrival
Journal of Air Transport Management(2020)
摘要
4D trajectory prediction is the core element of the future air transportation system. It aims to improve the operational ability and the predictability of air traffic. In this paper, a novel automated data-driven framework to deal with the prediction of Estimated Time of Arrival (ETA) on the runway at the entry point of Terminal Manoeuvring Area (TMA) is introduced. The proposed framework mainly consists of data preprocessing and machine learning models. Firstly, the dataset is divided, analyzed, cleaned, and estimated. Then, the flights are clustered into partitions according to different runway-in-use (QFU). Several candidate machine learning models are trained and selected on the corresponding dataset of each QFU. Feature engineering is conducted to transform raw data into features. After that, the experiments are performed on real ADS-B data in Beijing TMA with nested cross validation. By comparing the prediction performance on the preprocessed and un-preprocessed datasets, the results demonstrate that the proposed data preprocessing is able to improve the data quality. It is also robust to outliers, missing data, and noise. Finally, an ensemble learning strategy named stacking is introduced. Compared to other individual models, the stacked model has a more complex structure and performs best in ETA prediction. This fact reveals that the framework proposed in this study could make accurate and reliable ETA predictions.
更多查看译文
关键词
Air traffic management,4D trajectory prediction,Estimated time of arrival,Data mining,Machine learning
AI 理解论文
溯源树
样例
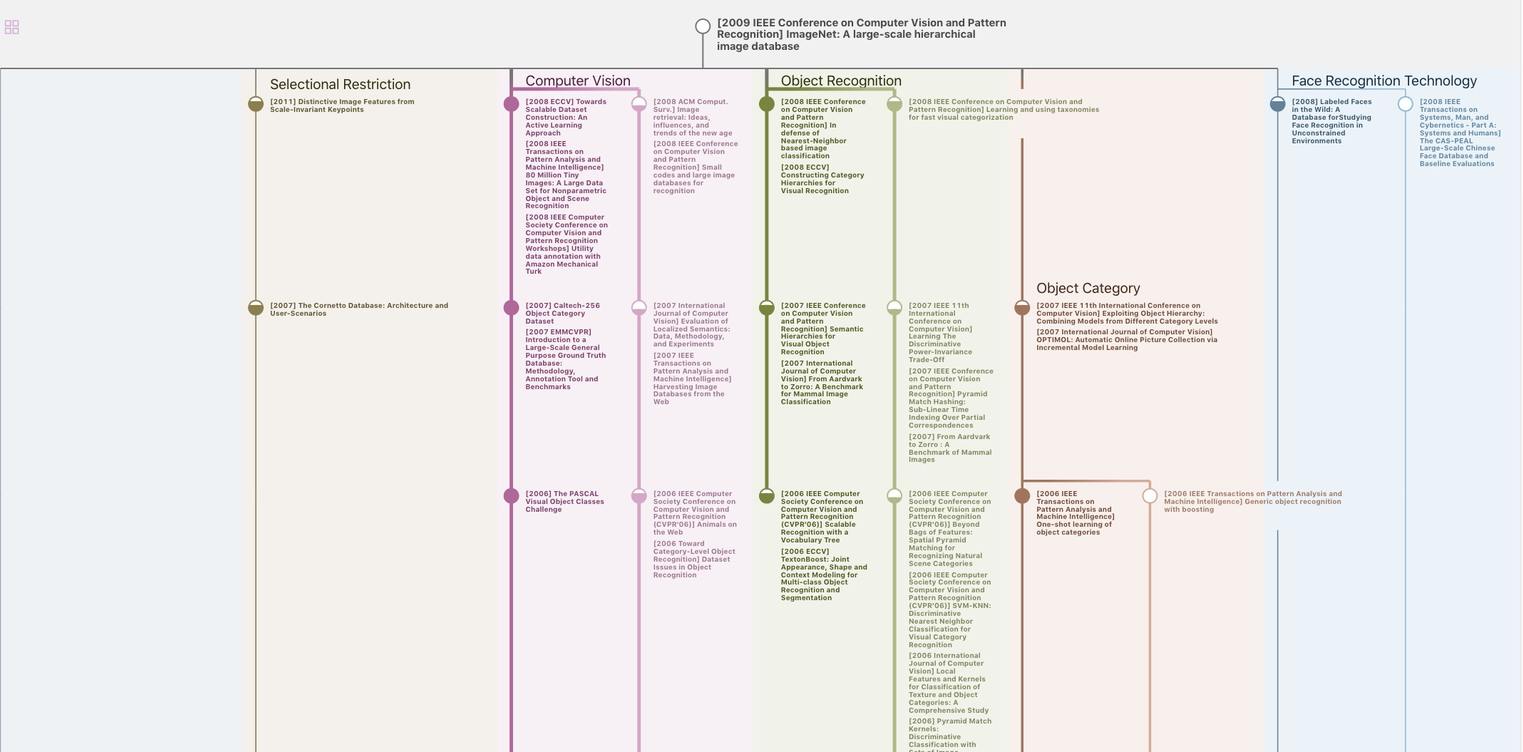
生成溯源树,研究论文发展脉络
Chat Paper
正在生成论文摘要