Machine-learning-based high-resolution DOA measurement and robust directional modulation for hybrid analog-digital massive MIMO transceiver
SCIENCE CHINA-INFORMATION SCIENCES(2020)
摘要
At hybrid analog-digital (HAD) transceiver, an improved HAD estimation of signal parameters via rotational invariance techniques (ESPRIT), called I-HAD-ESPRIT, is proposed to measure the direction of arrival (DOA) of a desired user, where the phase ambiguity due to HAD structure is dealt with successfully. Subsequently, a machine-learning (ML) framework is proposed to improve the precision of measuring DOA. Meanwhile, we find that the probability density function (PDF) of DOA measurement error (DOAME) can be approximated as a Gaussian distribution by the histogram method in ML. Then, a slightly large training data set (TDS) and a relatively small real-time set (RTS) of DOA are formed to predict the mean and variance of DOA/DOAME in the training stage and real-time stage, respectively. To improve the precisions of DOA/DOAME, three weight combiners are proposed to combine the-maximum-likelihood-learning outputs of TDS and RTS. Using the mean and variance of DOA/DOAME, their PDFs can be given directly, and we propose a robust beamformer for directional modulation (DM) transmitter with HAD by fully exploiting the PDF of DOA/DOAME, especially a robust analog beamformer on RF chain. Simulation results show that: (1) the proposed I-HAD-ESPRIT can achieve the HAD Cramer-Rao lower bound (CRLB); (2) the proposed ML framework performs much better than the corresponding real-time one without training stage; (3) the proposed robust DM transmitter can perform better than the corresponding non-robust ones in terms of secrecy rate.
更多查看译文
关键词
hybrid analog and digital,ESPRIT,statistical learning,DM,robust precoder
AI 理解论文
溯源树
样例
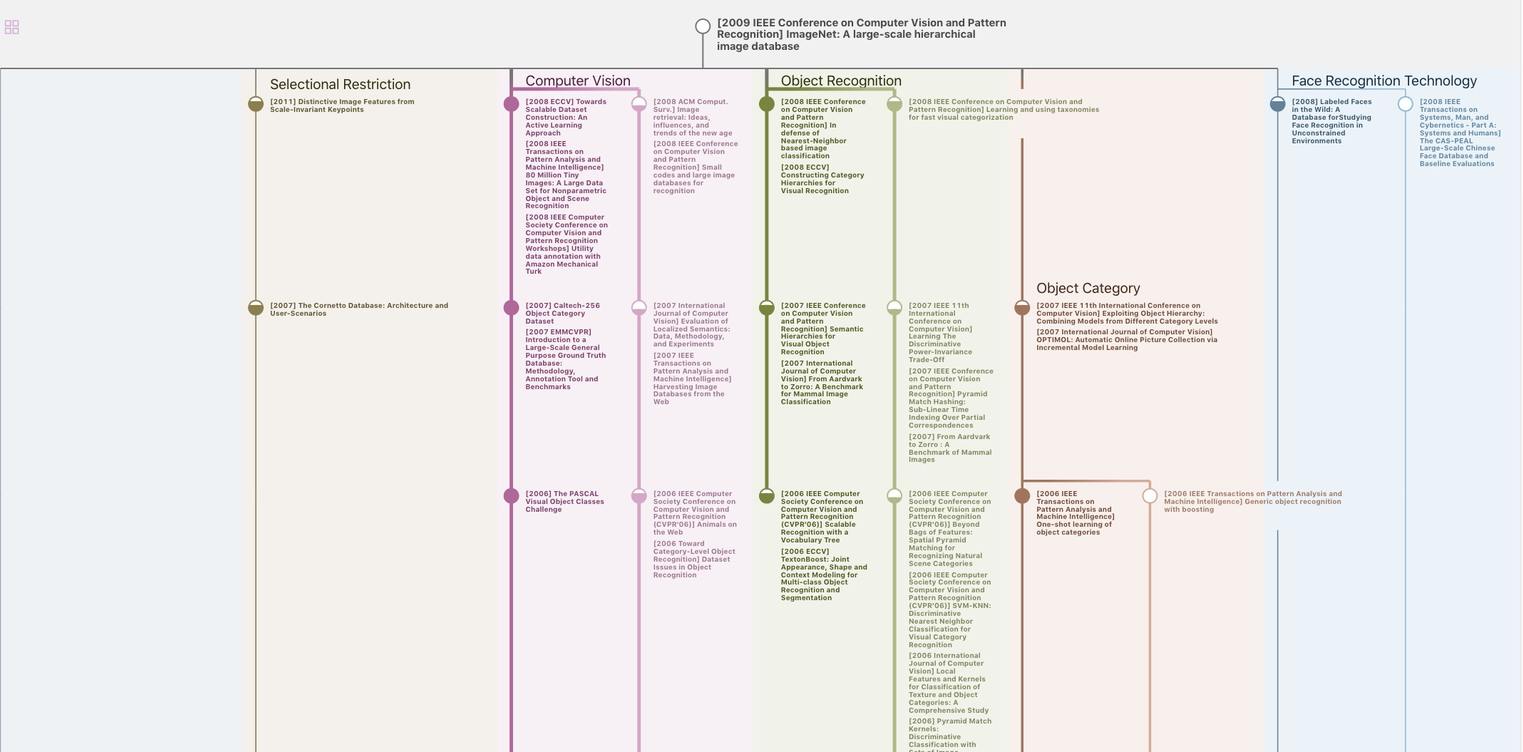
生成溯源树,研究论文发展脉络
Chat Paper
正在生成论文摘要