An Improved Sparse Autoencoder and Multilevel Denoising Strategy for Diagnosing Early Multiple Intermittent Faults
IEEE Transactions on Systems, Man, and Cybernetics: Systems(2022)
摘要
Currently, the diagnosis of single fault marked by distinct characteristics has received mass concern, and the related research achievements are remarkable. However, the diagnosis of early multiple intermittent (EMI) faults commonly existing in industrial systems is still an intractable problem, owing to: 1) the scarcity of fault data and the faintness of features and 2) the mutual interaction of components and the coupling of fault characteristics. In order to address the problem, this article proposes an improved sparse autoencoder and multilevel denoising strategy (MDS-ISAE) for diagnosing EMI faults. First, a relational constraint term is constructed to mitigate the effect of data correlation. Second, a multilevel denoising strategy is designed to enhance the robustness of AE feature learning with strong background noise. Third, a resizable resampling strategy is planned to tackle the skew distribution of diagnosis data. Based on the above strategies, an MDS-ISAE-based model is constructed to achieve EMI faults diagnosis. Further, the evaluation criteria of diagnosis performance are proposed and the applicability of the method is tested. Finally, the effectiveness and practicability of the proposed method are verified by artificial damage and real fault experiments, respectively.
更多查看译文
关键词
Early multiple intermittent (EMI) faults,fault diagnosis,multilevel denoising,relational constraint term,resizable resampling,sparse autoencoder
AI 理解论文
溯源树
样例
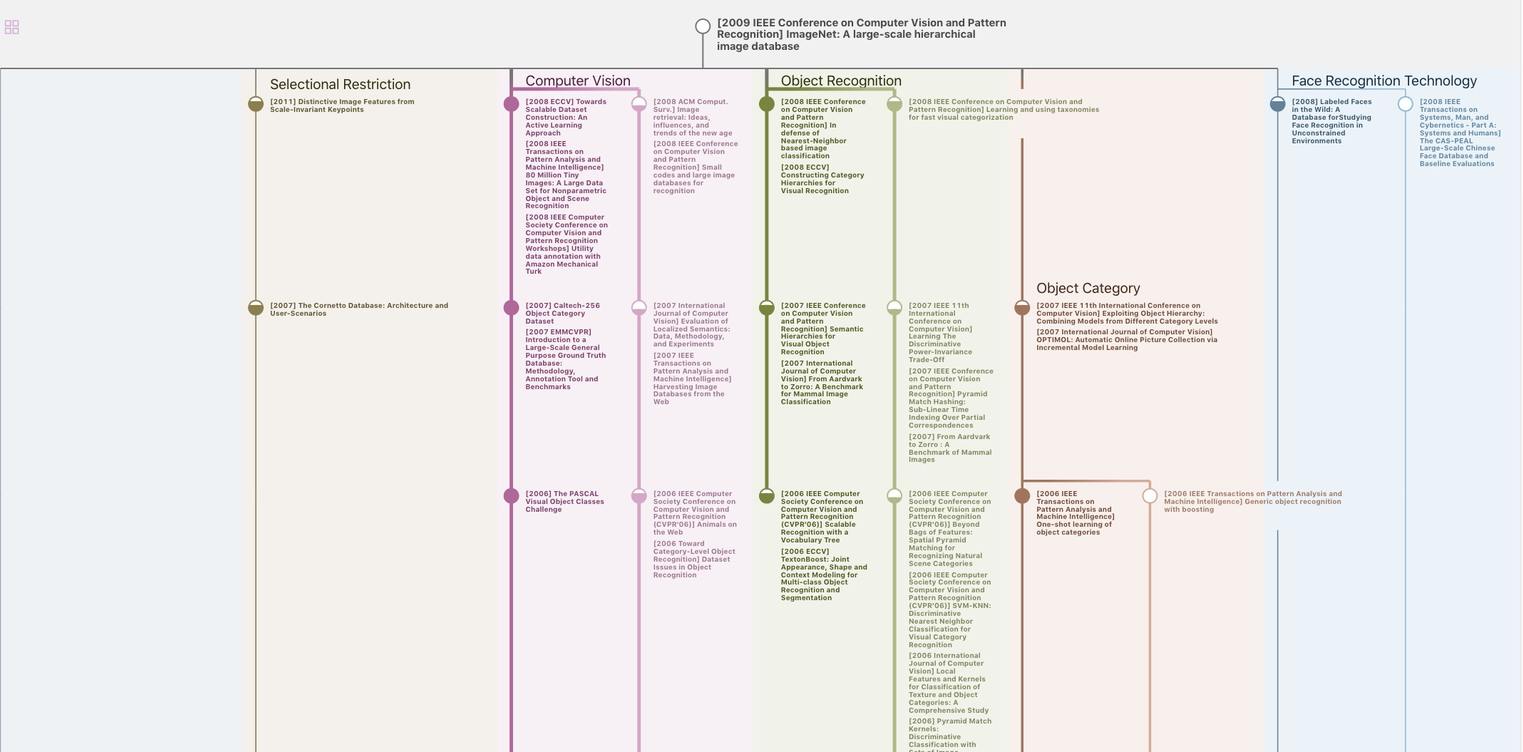
生成溯源树,研究论文发展脉络
Chat Paper
正在生成论文摘要