Sparse Logistic Maximum Likelihood Estimation for Optimal Well-Being Determinants
IEEE transactions on emerging topics in computing(2021)
摘要
Optimal well-being is a new multi-dimensional construct, which incorporates the well-known preexisting notions of subjective and psychological well-being. Classical models for describing the predictors of optimal well-being binary response variable are generalized linear models (GLM), such as logistic regression model. Since the number of predictors might be relatively large in these models, we devise a sparse optimization method for the regression problem based on subsequent iterations of a suitable sparse quadratic approximant problem, so that the resulting parameter vector estimate is sparse and indicates few significant predictors. We conduct empirical assessments using data of the European Social Survey (ESS), in order to identify the set of determinants which better predict optimal well-being by means of the proposed sparse regression method. ESS data analysis confirms that few selected predictors provide good data interpretation and no loss of information in the frequency of correct classification for people meeting the criteria of optimal well-being. Moreover, simulations with different structural parameter values indicate that sparse logistic model performs better in terms of the estimation of the true vector of parameters in a more parsimonious setting compared to classical logistic regression. The benefits increase as the structural sparsity of the optimization problem becomes stronger.
更多查看译文
关键词
Europe,Psychology,Logistics,Predictive models,Maximum likelihood estimation,Data analysis,Sparse representation,Optimal well-being,determinant predictors,sparse logistic regression,sparsity constraints,maximum likelihood
AI 理解论文
溯源树
样例
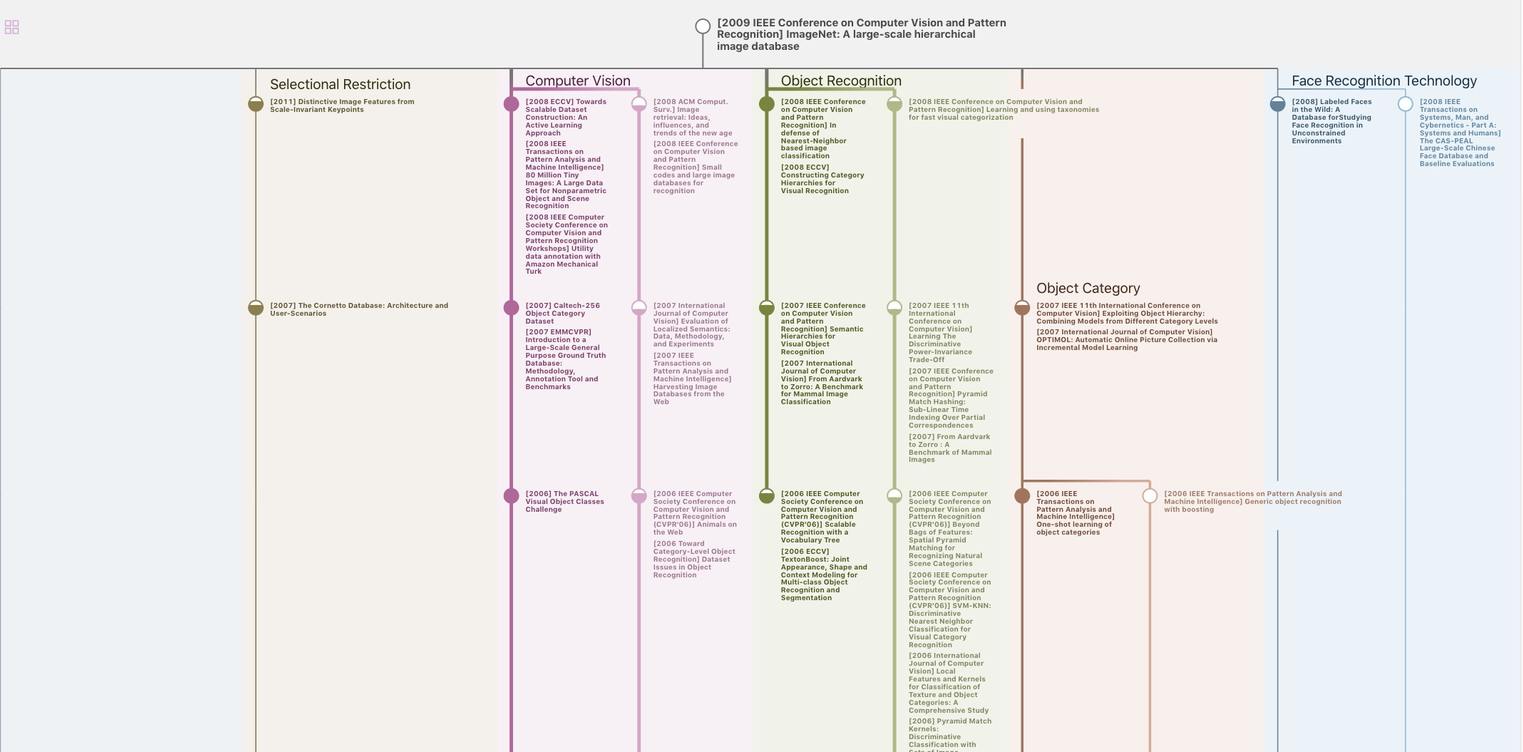
生成溯源树,研究论文发展脉络
Chat Paper
正在生成论文摘要