Spatio-temporal feature fusion for dynamic taxi route recommendation via deep reinforcement learning
Knowledge-Based Systems(2020)
摘要
Dynamic taxi route recommendation aims at recommending cruising routes to vacant taxis such that they can quickly find and pick up new passengers. Given citizens’ giant but unbalancing riding demand and the very limited taxis in a city, dynamic taxi route recommendation is essential for its ability to alleviate the waiting time of passengers and increase the earning of taxi drivers. Thus, in this paper we study the dynamic taxi route recommendation problem as a sequential decision-making problem and we design an effective two-step method to tackle it. First, we propose to consider and extract multiple real-time spatio-temporal features, which are related with the easiness degree of vacant taxis picking up new passengers. Second, we design an adaptive deep reinforcement learning method, which learns a carefully designed deep policy network to better fuse the extracted spatio-temporal features such that effective route recommendation can be done. Extensive experiments using real-world data from San Francisco and New York are conducted. Comparing with the state-of-the-arts, our method can increase at least 15.8% of average earning for taxi drivers and reduce at least 29.6% of average waiting time for passengers.
更多查看译文
关键词
Spatio-temporal feature fusion,Sequential decision making,Taxi route recommendation,Deep reinforcement learning,Transportation
AI 理解论文
溯源树
样例
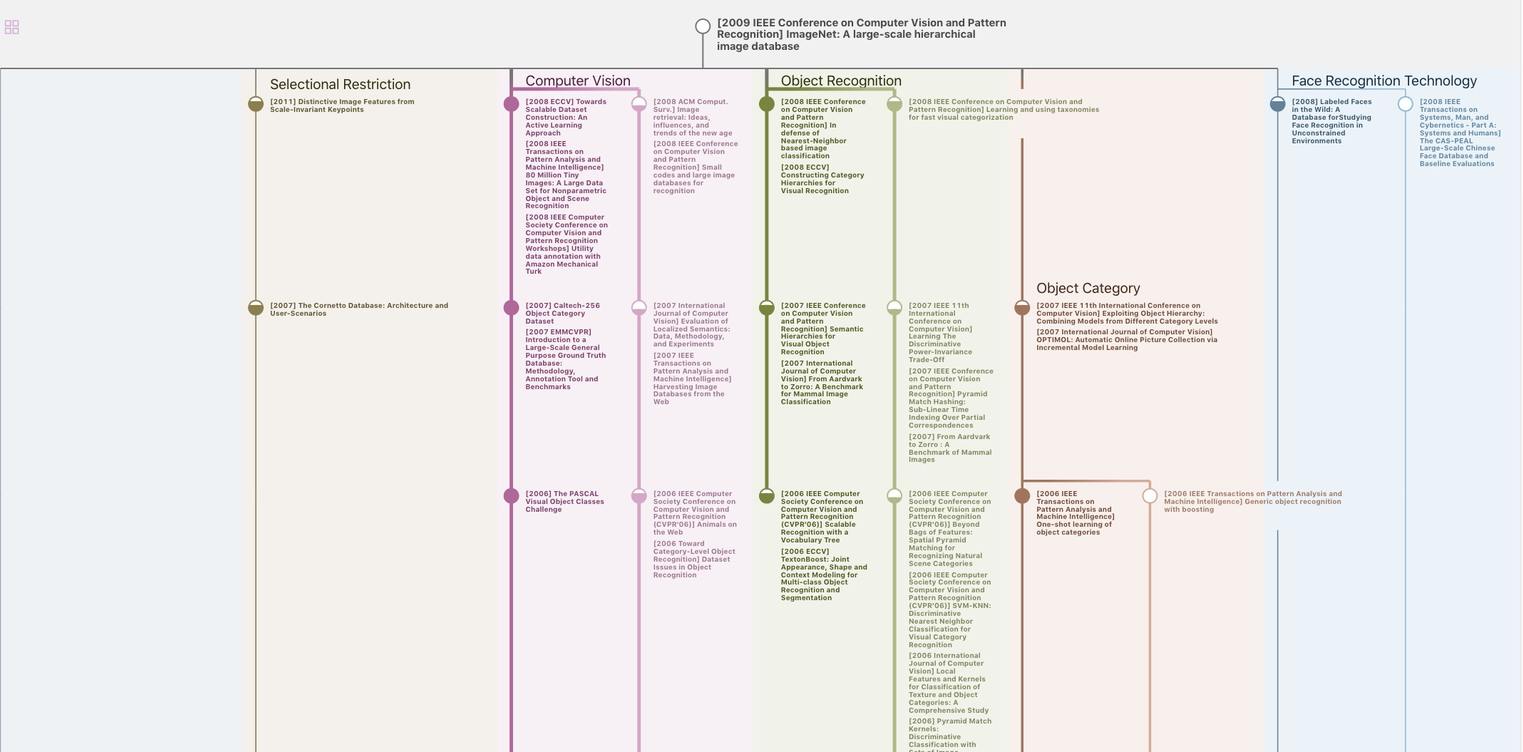
生成溯源树,研究论文发展脉络
Chat Paper
正在生成论文摘要