On Training Traffic Predictors via Broad Learning Structures: A Benchmark Study
IEEE Transactions on Systems, Man, and Cybernetics: Systems(2022)
摘要
A fast architecture for real-time (i.e., minute-based) training of a traffic predictor is studied, based on the so-called broad learning system (BLS) paradigm. The study uses various traffic datasets by the California Department of Transportation, and employs a variety of standard algorithms (LASSO regression, shallow and deep neural networks, stacked autoencoders, convolutional, and recurrent neural networks) for comparison purposes: all algorithms are implemented in MATLAB on the same computing platform. The study demonstrates a BLS training process two-three orders of magnitude faster (tens of seconds against tens-hundreds of thousands of seconds), allowing unprecedented real-time capabilities. Additional comparisons with the extreme learning machine architecture, a learning algorithm sharing some features with BLS, confirm the fast training of least-square training as compared to gradient training.
更多查看译文
关键词
Broad learning system (BLS),least-square methods,real-time software,real-time training,traffic flow prediction
AI 理解论文
溯源树
样例
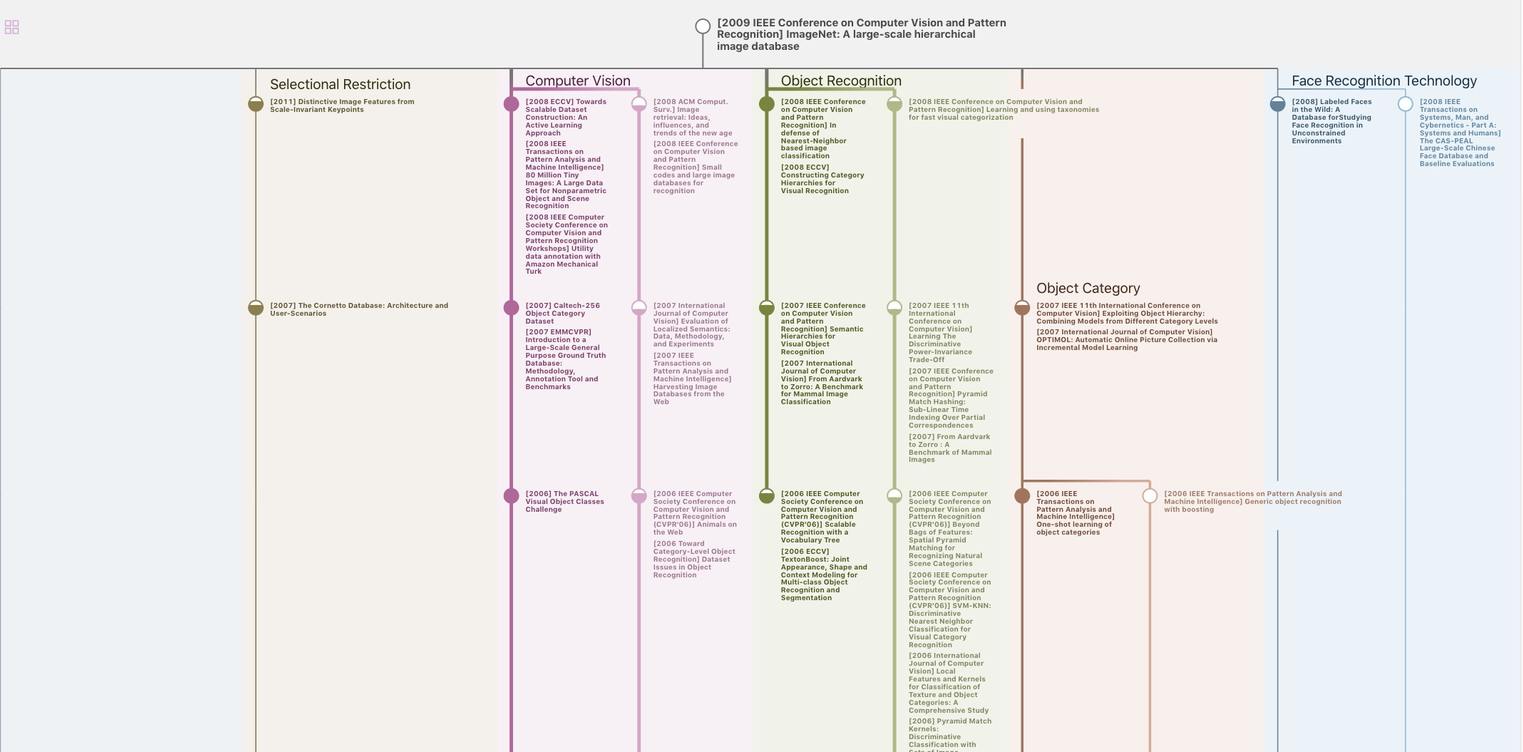
生成溯源树,研究论文发展脉络
Chat Paper
正在生成论文摘要