Informed Policies: Overcoming Subpolicy Dependence
international conference on robotics and automation(2019)
摘要
Hierarchical reinforcement learning addresses some of the difficulties that reinforcement learning has with long time horizon tasks by decomposing them into subtasks which are then solved separately. This formulation has the limitation that often the optimal solutions to the subtasks do not combine to be a globally optimal solution in terms of the overall task. This paper seeks to address this subpolicy independence problem through the use of informed policies. By passing information from subsequent subtask policies back into the current subtask policy, the disconnect between consecutive subtasks can be bridged and subtask policy can be considered in-formed. Using this method on an inverted pendulum domain task, we were able to show that using informed policies can outperform uninformed policies.
更多查看译文
关键词
policies,subpolicy dependence
AI 理解论文
溯源树
样例
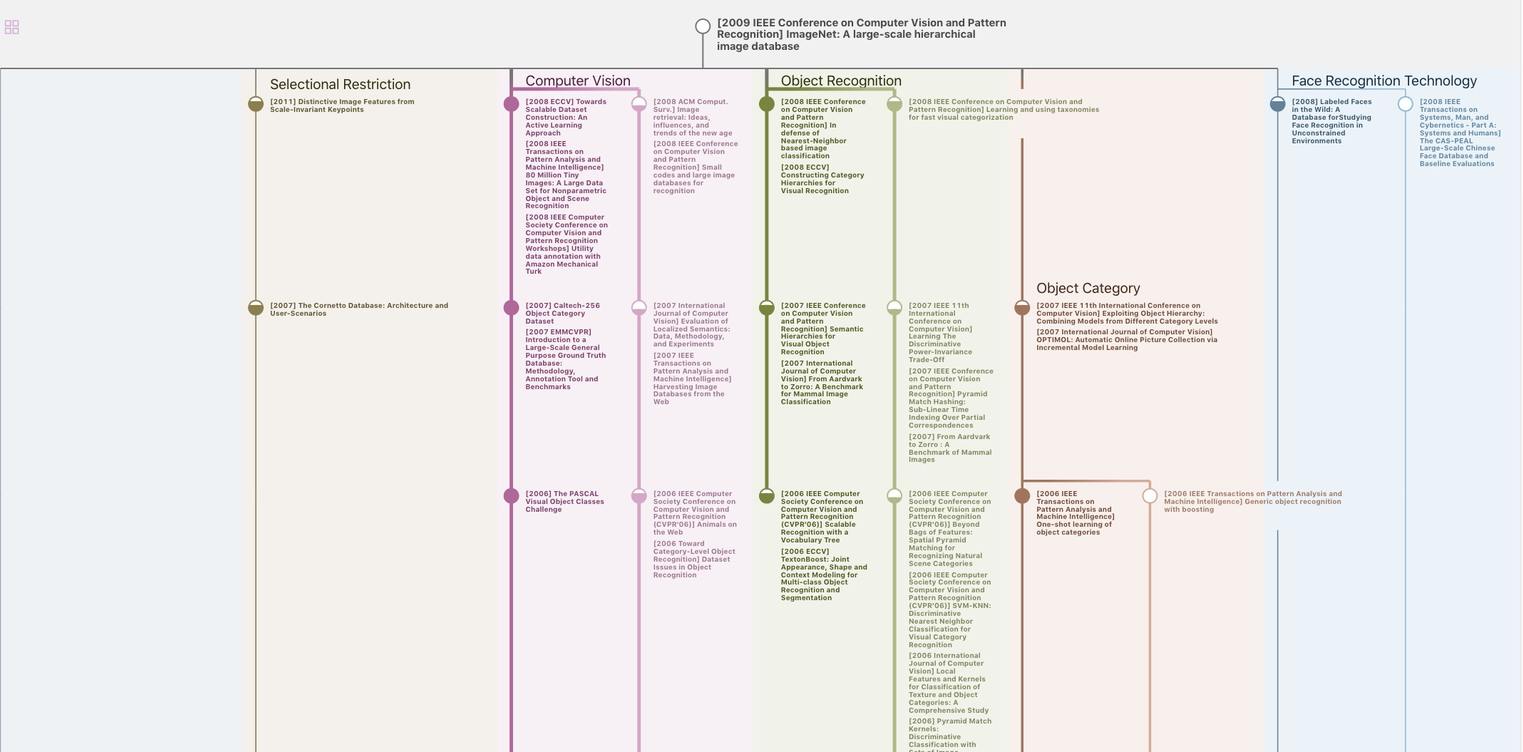
生成溯源树,研究论文发展脉络
Chat Paper
正在生成论文摘要