Modeling highly imbalanced crash severity data by ensemble methods and global sensitivity analysis
JOURNAL OF TRANSPORTATION SAFETY & SECURITY(2022)
摘要
Crash severity has been extensively studied and numerous methods have been developed for investigating the relationship between crash outcome and explanatory variables. Crash severity data are often characterized by highly imbalanced severity distributions, with most crashes in the Property-Damage-Only (PDO) category and the severe crash category making up only a fraction of the total observations. Many methods perform better on outcome categories with the most observations than other categories. This often leads to a high modeling accuracy for PDO crashes but poor accuracies for other severity categories. This research introduces two ensemble methods to model imbalanced crash severity data: AdaBoost and Gradient Boosting. It also adopts a more reasonable performance metric, F1 score, for model selection. It is found that AdaBoost and Gradient Boosting outperform other benchmark methods and generate more balanced prediction accuracies. Additionally, a global sensitivity analysis is adopted to determine the individual and joint impacts of explanatory factors on crash severity outcome. Vertical curve, seat belt use, accident type, road characteristics, and truck percentage are found to be the most influential factors. Finally, a simulation-based approach is used to further study how the impact of a particular factor may vary with respect to different value ranges.
更多查看译文
关键词
Crash severity, data mining, ensemble methods, global sensitivity analysis, safety
AI 理解论文
溯源树
样例
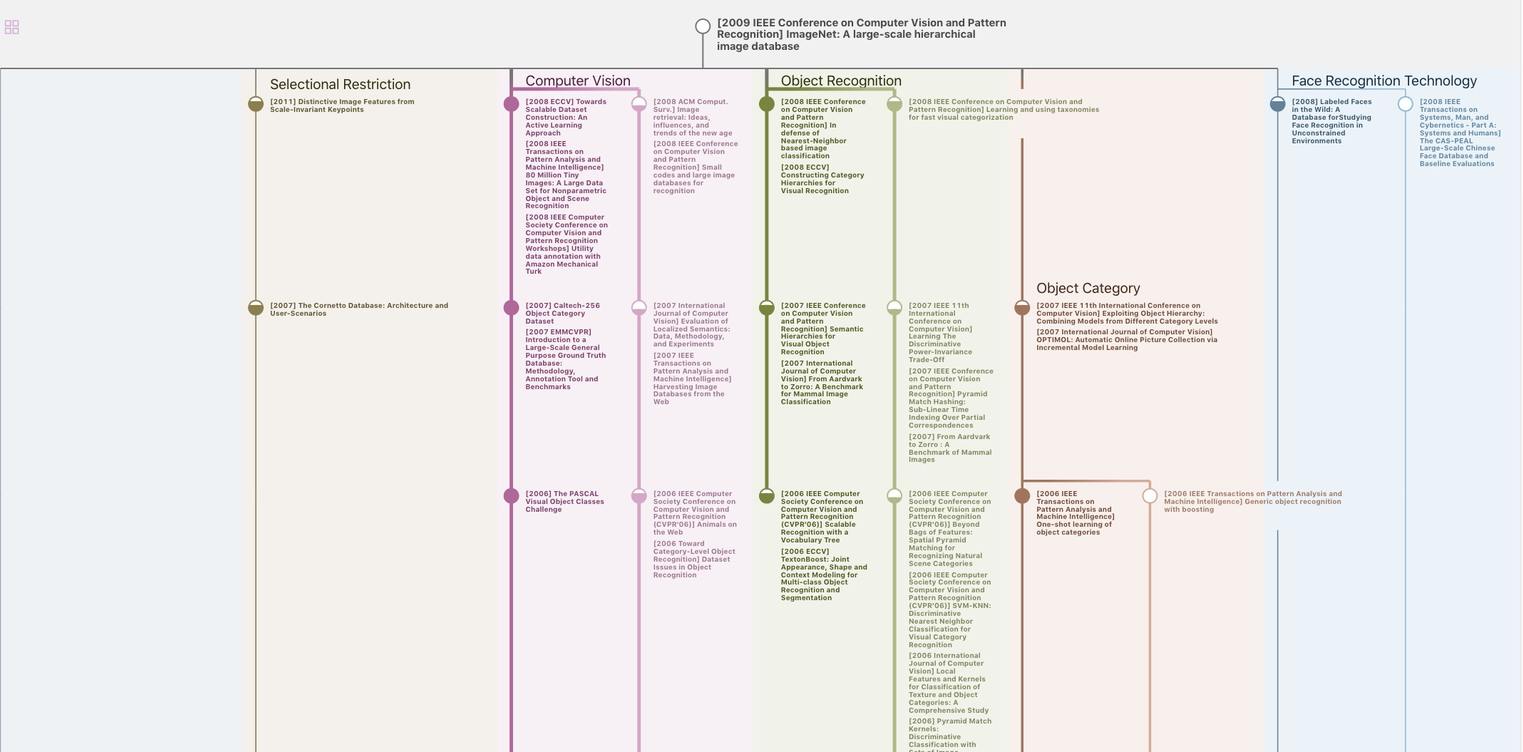
生成溯源树,研究论文发展脉络
Chat Paper
正在生成论文摘要