Base-Reconfigurable Segmented Logarithmic Quantization and Hardware Design for Deep Neural Networks
JOURNAL OF SIGNAL PROCESSING SYSTEMS FOR SIGNAL IMAGE AND VIDEO TECHNOLOGY(2020)
摘要
The growth in the size of deep neural network (DNN) models poses both computational and memory challenges to the efficient and effective implementation of DNNs on platforms with limited hardware resources. Our work on segmented logarithmic (SegLog) quantization, adopting both base-2 and base- √(2) logarithmic encoding, is able to reduce inference cost with a little accuracy penalty. However, weight distribution varies among layers in different DNN models, and requires different base-2 : base- √(2) ratios to reach the best accuracy. This means different hardware designs for the decoding and computing parts are required. This paper extends the idea of SegLog quantization by using layer-wise base-2 : base- √(2) ratio on weight quantization. The proposed base-reconfigurable segmented logarithmic (BRSLog) quantization is able to achieve 6.4x weight compression with 1.66
更多查看译文
关键词
Logarithmic quantization,Neural network,Arithmetic element,Embedded intelligence
AI 理解论文
溯源树
样例
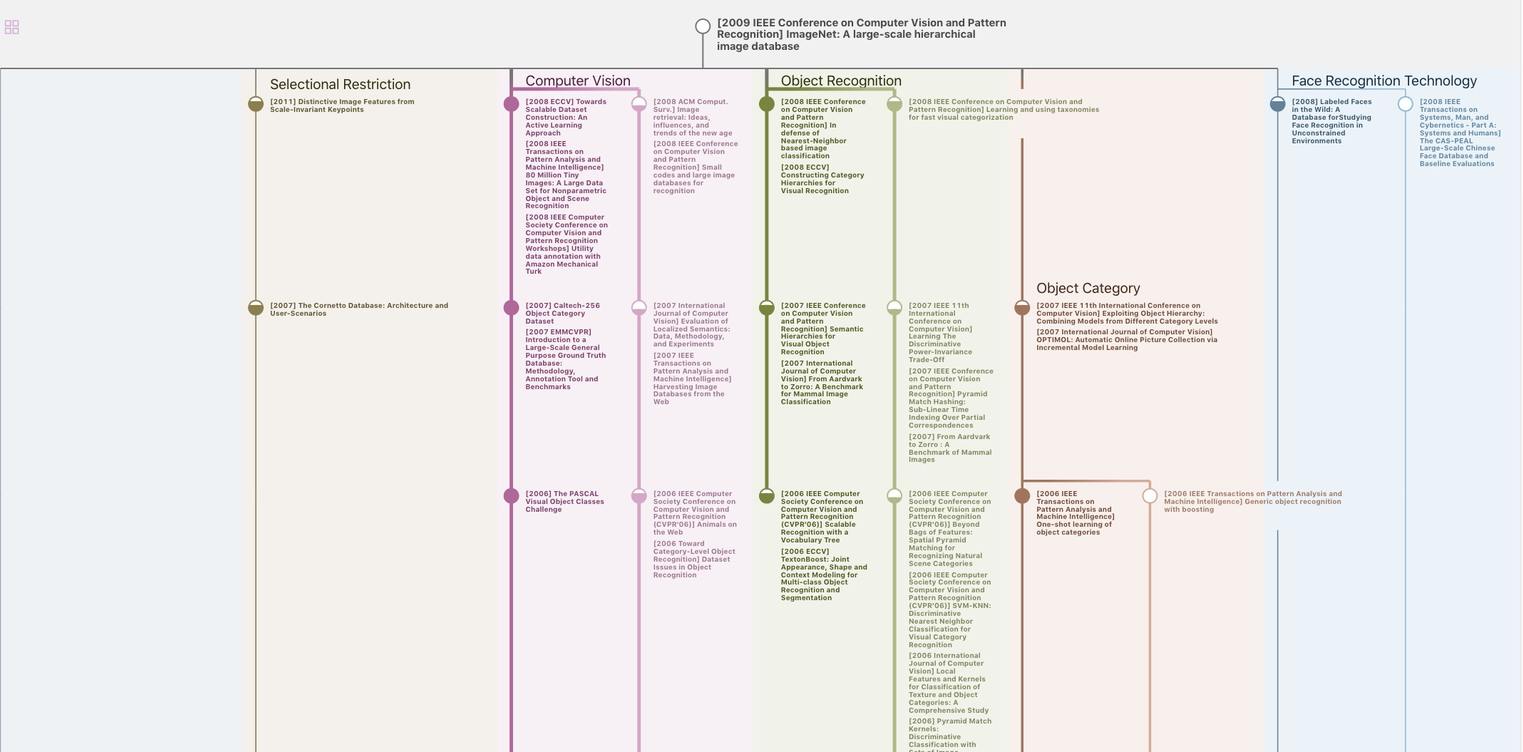
生成溯源树,研究论文发展脉络
Chat Paper
正在生成论文摘要