Community detection method using improved density peak clustering and nonnegative matrix factorization
Neurocomputing(2020)
摘要
Community detection in networks is valuable in analyzing, designing, and optimizing complex network. Recently, the nonnegative matrix factorization (NMF) method has successfully uncovered the community structure in the complex networks. However, most of community detection methods based on NMF require the number of the community as a prior information. To address this problem, in this paper, we use the improved density peak clustering (DPC) to obtain the number of centers as the pre-assigned parameter for nonnegative matrix factorization. The proposed algorithm first calculates the modified PageRank of nodes as the density indexes and then draws a decision graph to obtain the hubs of the network. By means of Markov chain model of a random walk, we execute NMF on one expansion feature matrix. Finally, we compare and analyze the performance of different algorithms on artificial networks and real-world networks. Experimental results indicate that the proposed method is superior to the state-of-the-art methods.
更多查看译文
关键词
Community detection,Nonnegative matrix factorization,Density peak clustering,Random walk,Amendatory PageRank
AI 理解论文
溯源树
样例
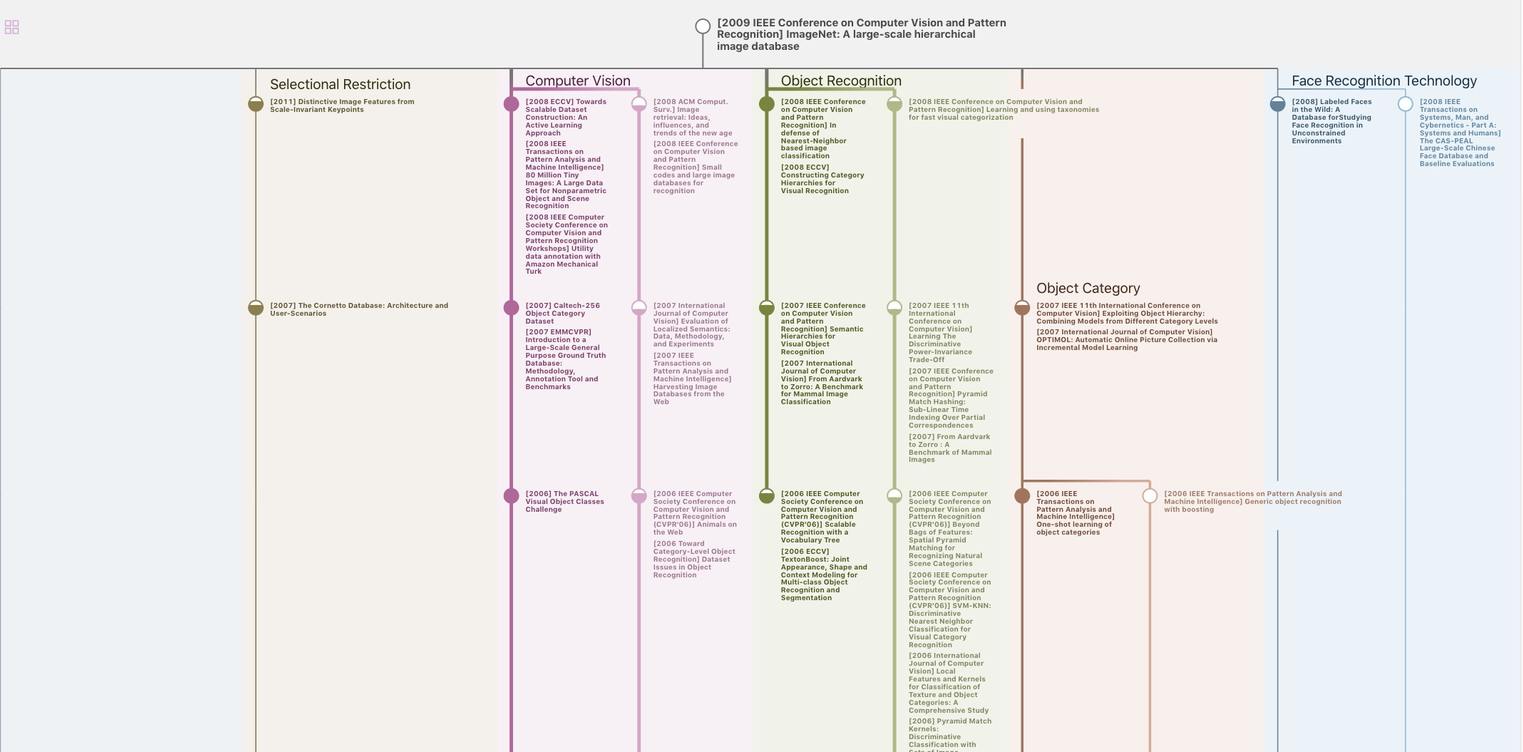
生成溯源树,研究论文发展脉络
Chat Paper
正在生成论文摘要