Feature Selection for High Dimensional Data Using Weighted K-Nearest Neighbors and Genetic Algorithm
IEEE ACCESS(2020)
摘要
Too many input features in applications may lead to over-fitting and reduce the performance of the learning algorithm. Moreover, in most cases, each feature containing different information content has different effects on the prediction target. Therefore, a feature selection method for calculating the importance of each feature, called WKNNGAFS, is proposed in this paper. In this method, the genetic algorithm (GA) is adopted to search the optimal weight vector, the value of the i th component of which corresponds to the contribution degree of the i th feature to the classification from a global perspective. Besides, weighted K-nearest neighbors algorithm (WKNN), which takes both the different contributions of nearest neighbors and the different classification ability of each feature into account, is used to determine the target label. To evaluate the effectiveness of the proposed method, nine existing feature selection methods are compared with it on 13 real datasets, including 6 high dimensional microarray datasets. Experimental results demonstrate the method is more effective and can improve classification performance.
更多查看译文
关键词
Feature selection,weighted K-nearest neighbors,genetic algorithm,real coding
AI 理解论文
溯源树
样例
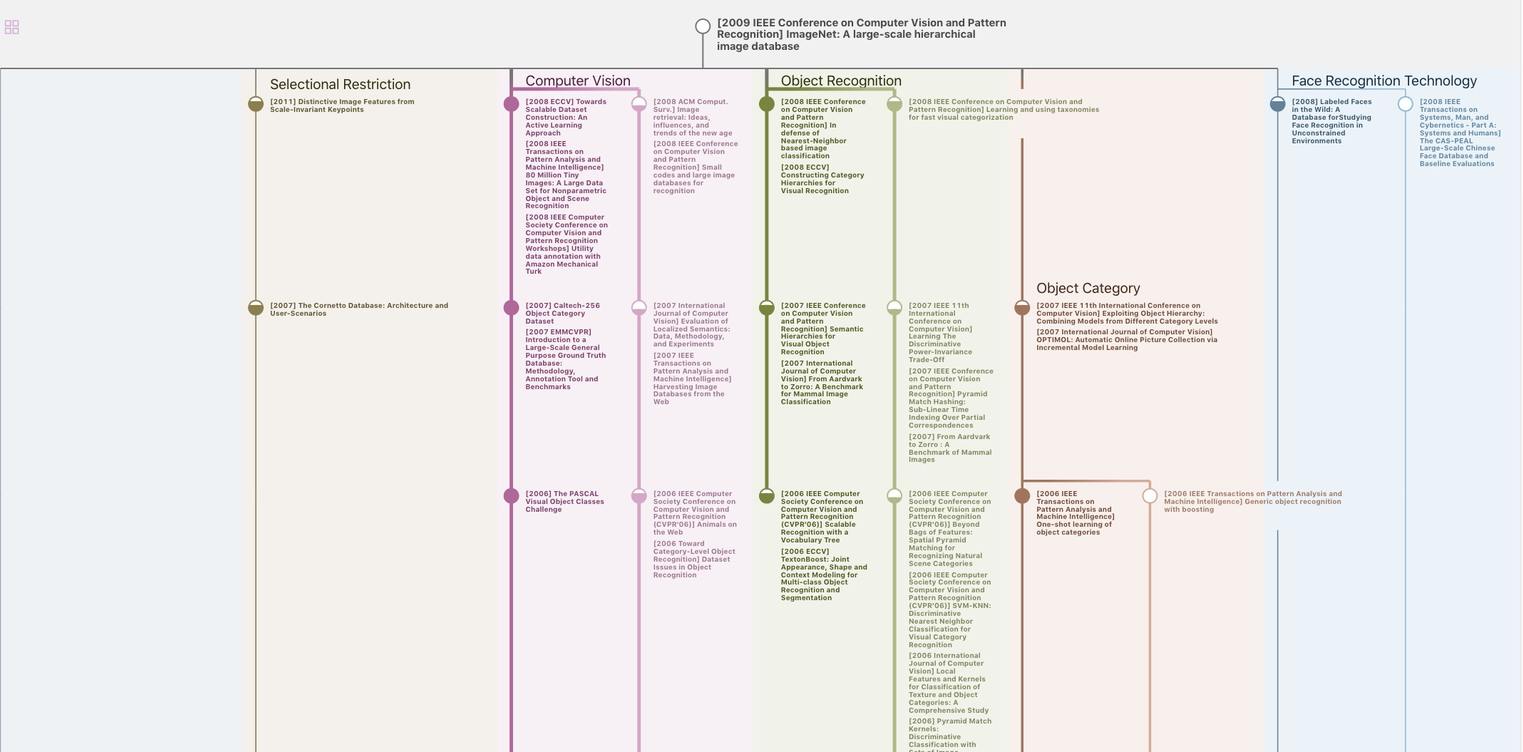
生成溯源树,研究论文发展脉络
Chat Paper
正在生成论文摘要