Few-labeled visual recognition for self-driving using multi-view visual-semantic representation
Neurocomputing(2021)
摘要
For a car self-driving system, it is vital to accurately recognize the objects on the road. This is often achieved by analyzing the images captured by various cameras. However, in real applications, we often have limited number of labeled images. Besides, it is very expensive to manually annotate objects in images. It is hard to learn reliable classifiers when only few-labeled images are available. To alleviate these problems, multi-view information is used. However, only using visual information is not enough for reliable classification. Besides, we often have limited images with labels. To cope with these problems, in this paper, we propose a novel multi-view visual-semantic representation method for few-labeled visual recognition (MV2S). We make use of the state-of-the-art deep convolutional neural networks by viewing them as different views to extract semantic representations of images. This is achieved by using the learned deep convolutional neural networks to make predictions of the semantics of images. Both the visual and semantic representations of images are then used to predict the categories of images by combining the predictions of multi-views with visual and semantic consistency constraints. Experiments on four public available datasets prove the effectiveness of the proposed MV2S method.
更多查看译文
关键词
Few-labeled view recognition,Self-driving object categorization,Multi-view learning,Semantic representation
AI 理解论文
溯源树
样例
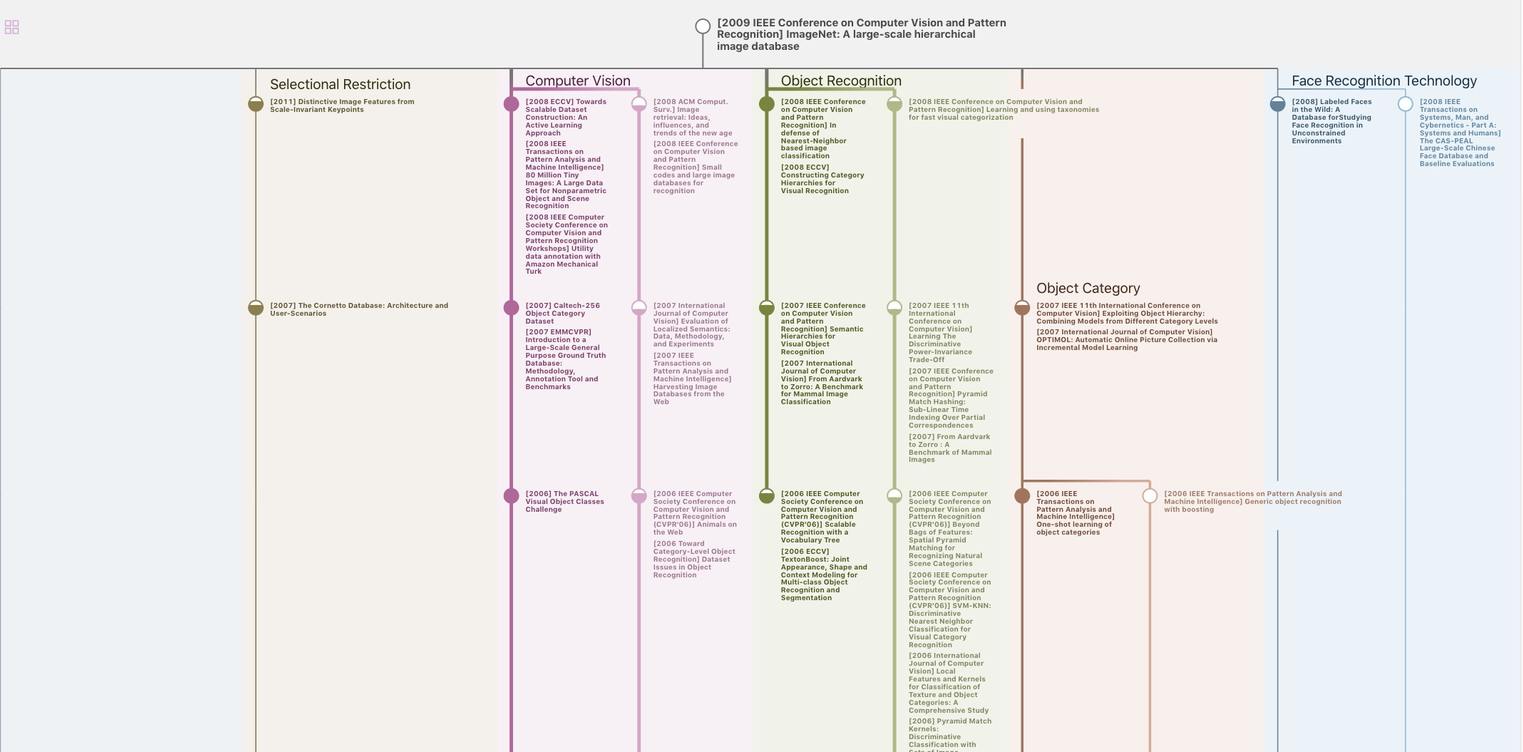
生成溯源树,研究论文发展脉络
Chat Paper
正在生成论文摘要