Knowledge-shot learning: An interpretable deep model for classifying imbalanced electrocardiography data
Neurocomputing(2020)
摘要
Classification of Electrocardiogram (ECG) data has been an important research topic in the machine learning area for many years. Recently, deep learning methods have been used in classifying ECG data and have achieved superior results than traditional methods. However, in real-world applications, two challenges existing deep learning methods cannot handle well – imbalanced data and model interpretability. In this paper, we propose an ECG classification method named Knowledge-Shot Learning (KSL) that can handle with the above two challenges. KSL constructs a novel neural network architecture that can be effectively trained on imbalanced ECG data. Besides, KSL can also extract interpretable feature vectors and give support cases as result explanations. Moreover, KSL can even classify unseen diseases if provided with the necessary medical knowledge. Experiments on real-world ECG data show that KSL improves 10.00% of F1-score on imbalanced classes, and 43.75% of F1-score on unseen classes, compared with the second-best baseline. KSL also provides interpretable results that are consistent with medical domain knowledge.
更多查看译文
关键词
Heartbeats classification,Imbalance problem,Neural network,Interpretable Model
AI 理解论文
溯源树
样例
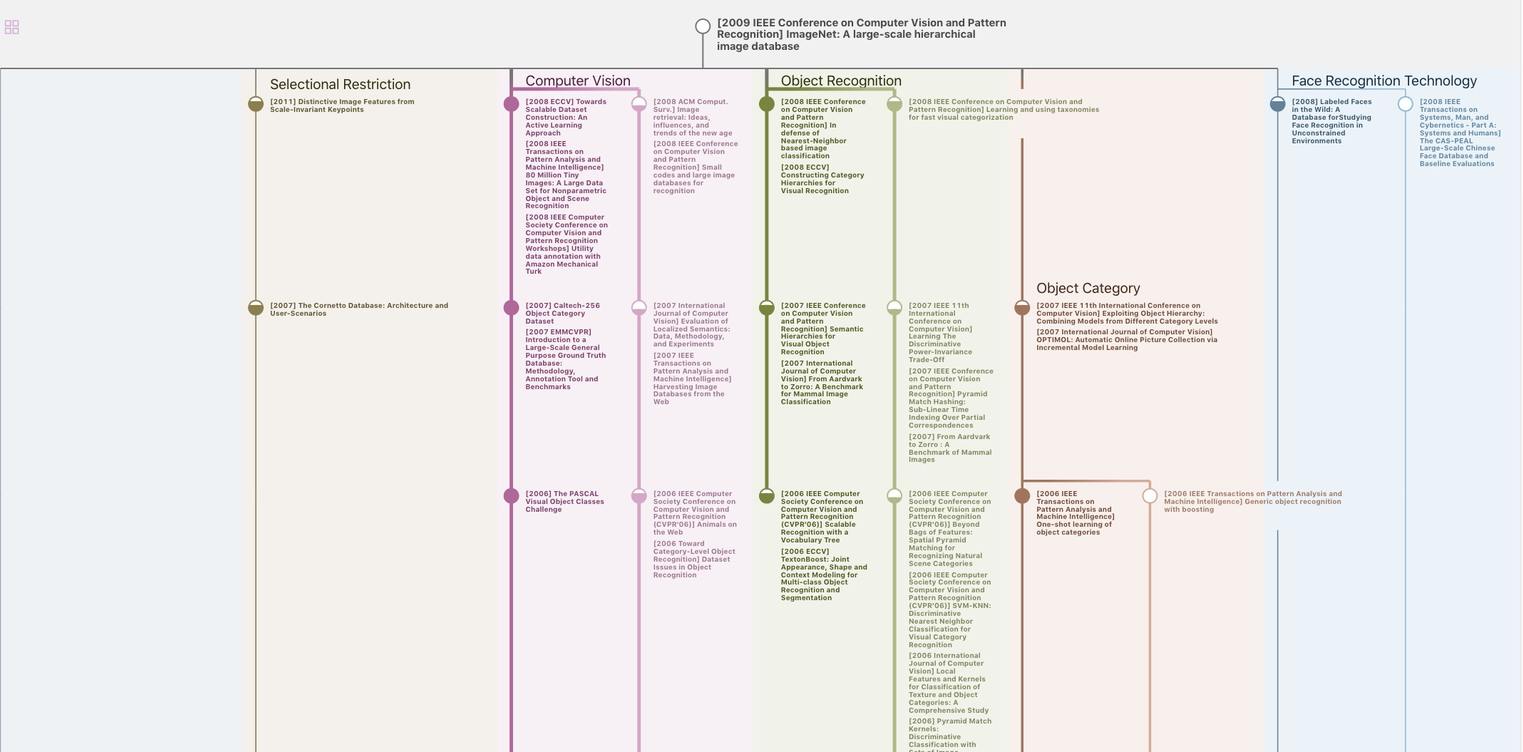
生成溯源树,研究论文发展脉络
Chat Paper
正在生成论文摘要