Risk Factors Discovery for Cancer Survivability Analysis Using Graph-Rule Mining
MATHEMATICAL PROBLEMS IN ENGINEERING(2020)
摘要
Mining and understanding patients' disease-development pattern is a major healthcare need. A huge number of research studies have focused on medical resource allocation, survivability prediction, risk management of diagnosis, etc. In this article, we are specifically interested in discovering risk factors for patients with high probability of developing cancers. We propose a systematic and data-driven algorithm and build around the idea of association rule mining. More precisely, the rule-mining method is firstly applied on the target dataset to unpack the underlying relationship of cancer-risk factors, via generating a set of candidate rules. Later, this set is represented as a rule graph, where informative rules are identified and selected with the aim of enhancing the result interpretability. Compared to hundreds of rules generated from the standard rule-mining approach, the proposed algorithm benefits from a concise rule subset, without losing the information from the original rule set. The proposed algorithm is then evaluated using one of the largest cancer data resources. We found that our method outperforms existing approaches in terms of identifying informative rules and requires affordable computational time. Additionally, relevant information from the selected rules can also be used to inform health providers and authorities for cancer-risk management.
更多查看译文
AI 理解论文
溯源树
样例
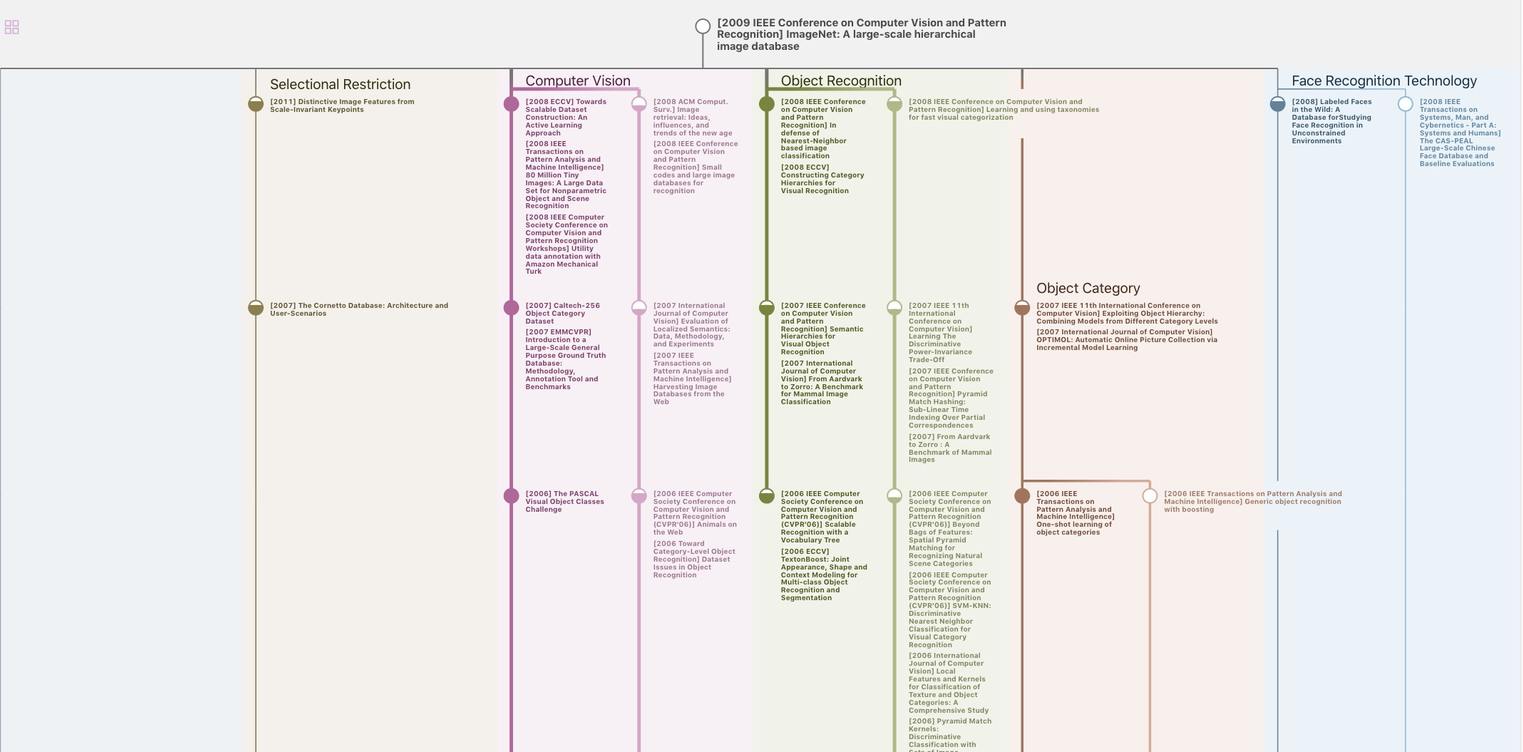
生成溯源树,研究论文发展脉络
Chat Paper
正在生成论文摘要