Momentum-Incorporated Symmetric Non-Negative Latent Factor Models
IEEE Transactions on Big Data(2022)
摘要
Symmetric high-dimensional and sparse (SHiDS) networks are frequently found in various industrial applications. A symmetric non-negative latent factor (SNLF) model can acquire essential features from them precisely, yet it suffers from slow convergence. To address this issue, this article integrates a generalized momentum method into a symmetric, single latent factor-dependent, non-negative and multiplicative update (S
2
LF-NMU) algorithm, thereby achieving a
m
omentum-incorporated,
s
ymmetric,
s
ingle-latent-factor-dependent
n
on-negative-multiplicative-update (MS
2
N) algorithm. Based on an MS
2
N algorithm, momentum-incorporated symmetric non-negative latent factor (MSNLF) models are proposed for an SHiDS network, which ensures fast convergence as well as high representative learning ability. Empirical studies on four SHiDS networks from industrial applications demonstrate that compared with state-of-the-art models, the proposed MSNLF models have significantly higher computational efficiency and representative learning ability.
更多查看译文
关键词
Big Data,data science,computational intelligence,symmetric high-dimensional and sparse network,non-negative latent factor analysis,generalized momentum,momentum effects,undirected network,missing data estimation
AI 理解论文
溯源树
样例
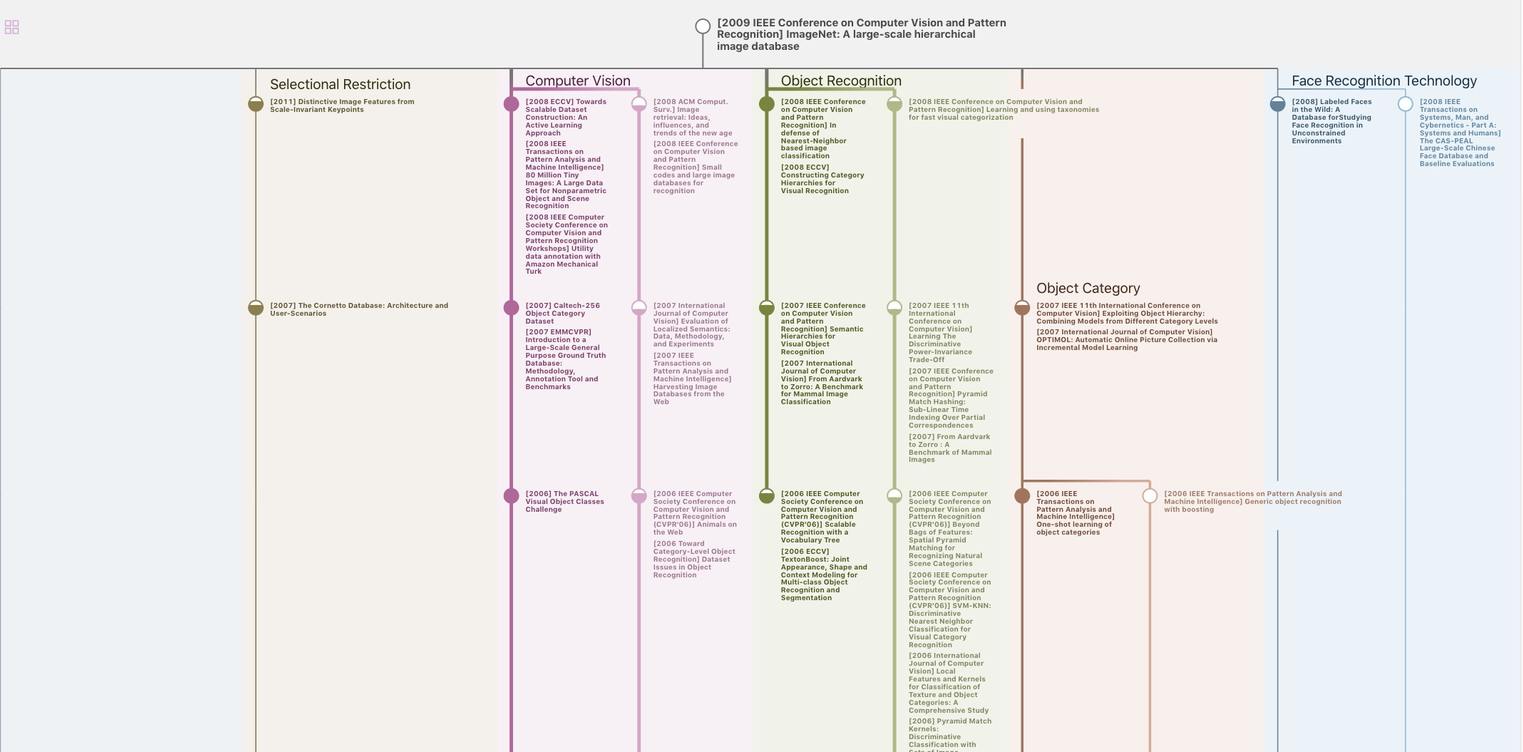
生成溯源树,研究论文发展脉络
Chat Paper
正在生成论文摘要