3D Model Retrieval Based on a 3D Shape Knowledge Graph
IEEE ACCESS(2020)
摘要
A development of 3D construction technology has led to 3D models being applied in many fields, and the number of 3D models has exploded in recent years. Thus, 3D model retrieval has become a popular topic with many proposed approaches. However, all of the methods focus on the 3D model's global structural descriptor design based on various deep learning networks and ignore the local structural information of the 3D model and the correlation of the local structures. In this paper, we propose a novel 3D model retrieval method based on a 3D shape knowledge graph. We first introduce the concept of a geometric word that can be utilized to assemble other 3D model. Second, we construct a 3D shape knowledge graph based on the geometric words, models and their relations. Additionally, we propose a novel graph embedding method to generate embeddings of nodes. Finally, an effective multiple entities' retrieval method is used to handle the 3D model retrieval problem. More specifically, the 3D shape knowledge graph retains the basic structural information and saves these as a set of triples. Any 3D model can find its geometric words in a rich enough knowledge graph. It is reasonable that our approach can solve the cross-domain model retrieval problem. Our approach focuses on the structural information of 3D model and is not restricted by the database. We evaluate the proposed method on the ModelNet40 dataset for the 3D model retrieval task. Meanwhile, we also utilize the ShapeNet dataset to evaluate the performance of cross-domain retrieval task. Experimental results and comparisons with state-of-the-art methods demonstrate that our framework can achieve superior performance.
更多查看译文
关键词
Three-dimensional displays,Solid modeling,Shape,Task analysis,Data models,Machine learning,Biological system modeling,3D model retrieval,knowledge graph,graph embedding,multi-view representation
AI 理解论文
溯源树
样例
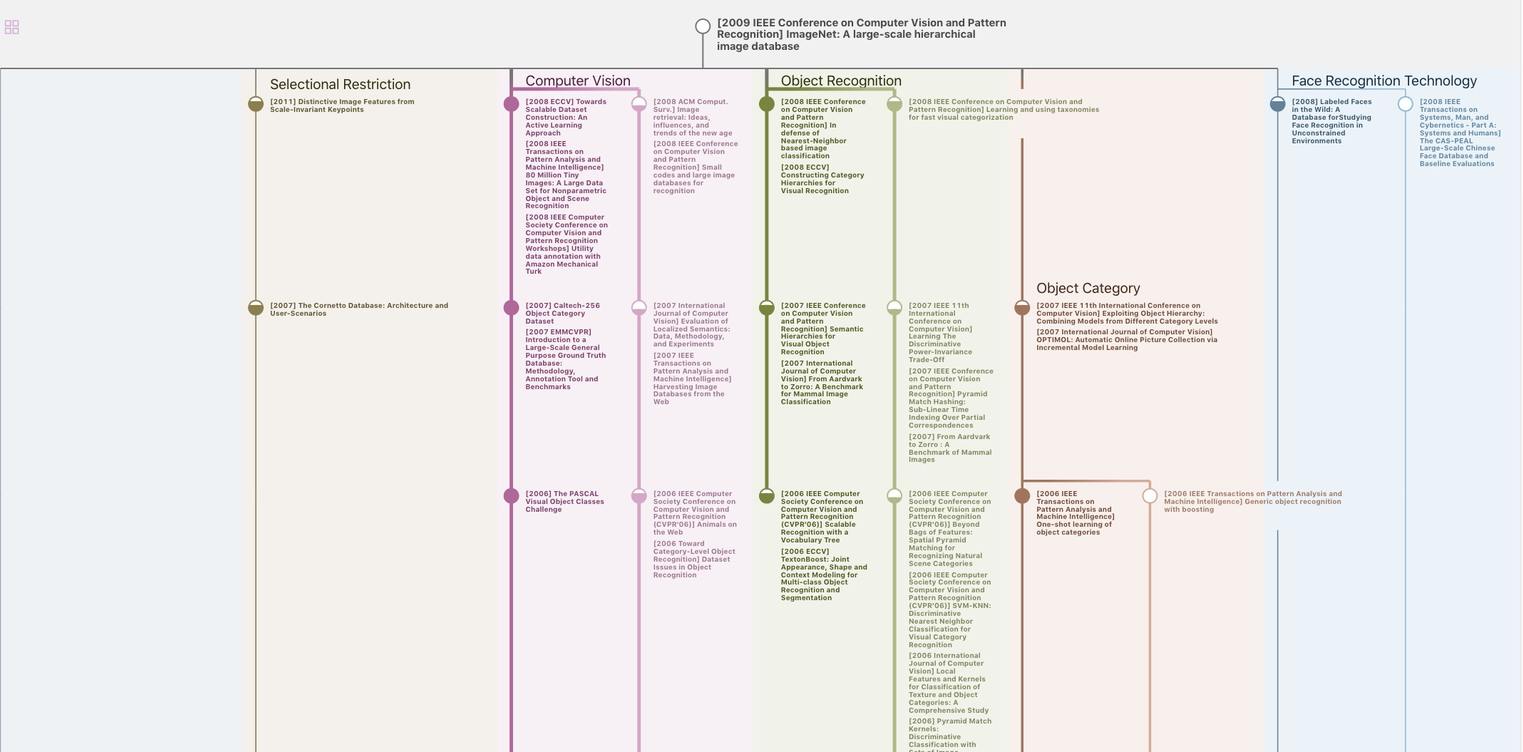
生成溯源树,研究论文发展脉络
Chat Paper
正在生成论文摘要