Small-Object Detection in UAV-Captured Images via Multi-Branch Parallel Feature Pyramid Networks
IEEE ACCESS(2020)
摘要
Small object is one of the primary challenges in the field of object detection, which is notably pronounced to the detection in the images from Unmanned Aerial Vehicles (UAV). Existing detectors based on deep-learning methods usually apply the feature extraction networks with a large down-sampling factor to obtain higher-level features. However, such big stride tends to make the feature information of small objects become the little point or even vanish in the low-resolution feature maps due to the limitation of pixels. Therefore, a novel structure called Multi-branch Parallel Feature Pyramid Networks (MPFPN) is proposed in this article, which aims to extract more abundant feature information of the objects with a small size. Specifically, the parallel branch is designed to recover the features that missed in the deeper layers. Meanwhile, a supervised spatial attention module (SSAM) is applied to weaken the impact of background noise inference and focus object information. Furthermore, we adopt cascade architecture in the Fast R-CNN stage for a more powerful localization capability. Experiments on the public drone-based datasets named VisDrone-DET demonstrate that our method achieves competitive performance compared with other state-of-the-art detection algorithms.
更多查看译文
关键词
Feature extraction,Object detection,Detectors,Proposals,Unmanned aerial vehicles,Noise measurement,Computer architecture,Unmanned aerial vehicle,object detection,multi-branch parallel feature pyramid networks (MPFPN),feature fusion,cascade architecture
AI 理解论文
溯源树
样例
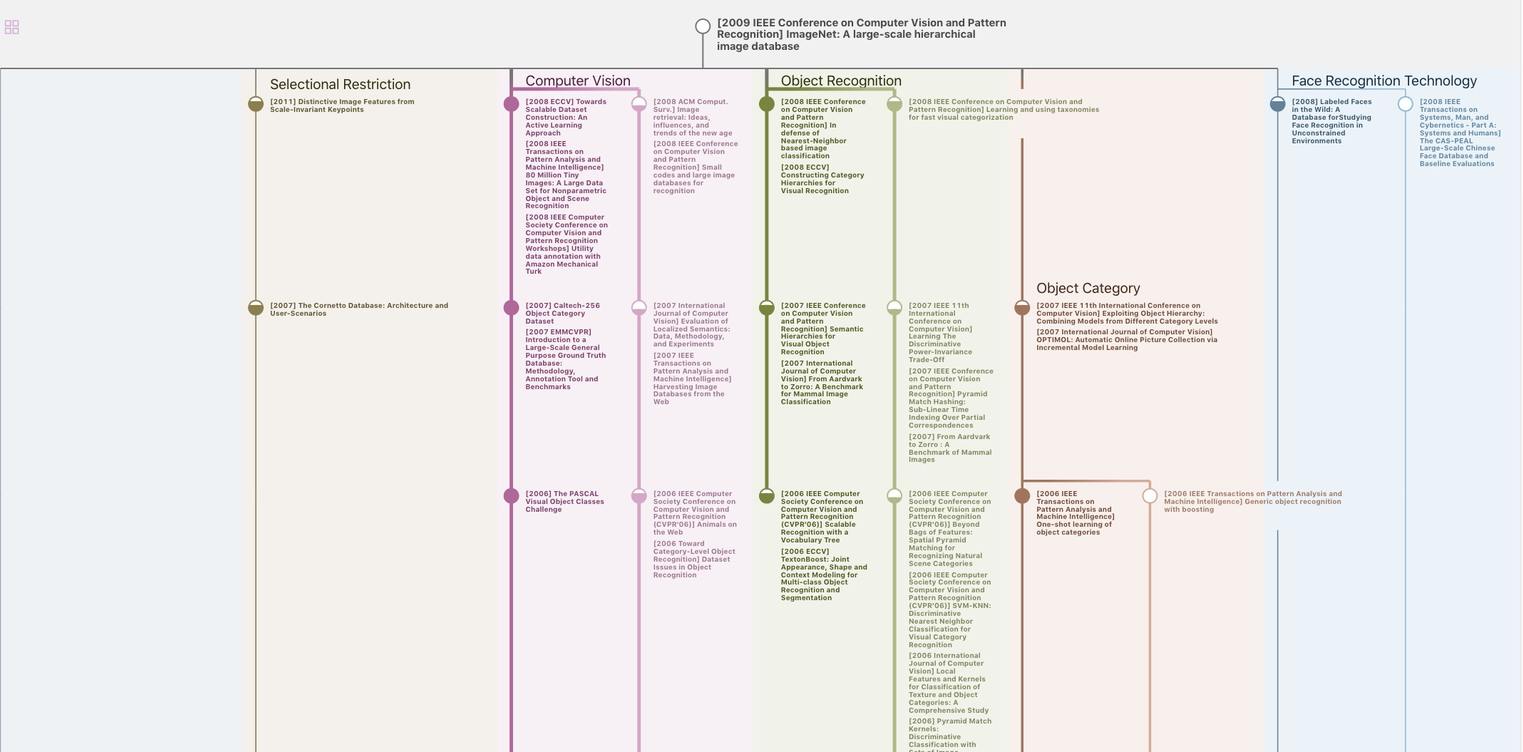
生成溯源树,研究论文发展脉络
Chat Paper
正在生成论文摘要