Using Information Criteria Under Missing Data: Full Information Maximum Likelihood Versus Two-Stage Estimation
STRUCTURAL EQUATION MODELING-A MULTIDISCIPLINARY JOURNAL(2021)
摘要
The full information maximum likelihood (FIML) and the two-stage (TS) procedure are two popular likelihood-based approaches to SEM model estimation with missing data. After model estimation, one often needs to choose the best model from a group of candidate models. A popular type of model selection tools is information criteria. Because FIML and TS both give consistent model parameter estimates, it is tempting to assume both FIML-based and TS-based information criteria are appropriate and useful. However, in this paper we show FIML and TS do not both give appropriate information criteria, and model selection results may be different from those under complete data, even in large samples. We first analytically study the implications of missing (completely) at random data for information criteria. Next, we conduct simulations to verify our theoretical proof and understand the empirical performance of information criteria. Our conclusions apply to AIC, BIC, and their variants.
更多查看译文
关键词
Information criteria, missing data, full information maximum likelihood, two-stage estimation
AI 理解论文
溯源树
样例
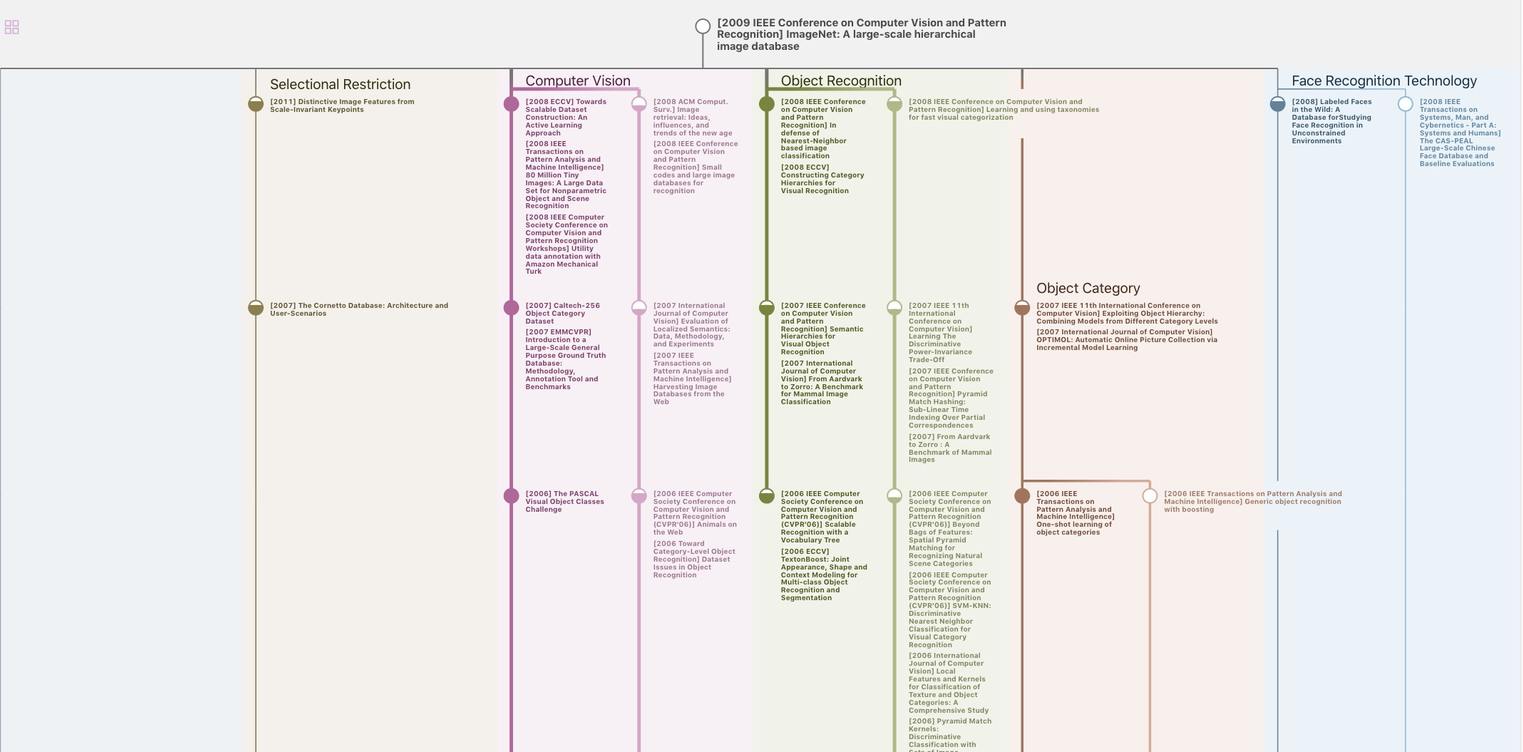
生成溯源树,研究论文发展脉络
Chat Paper
正在生成论文摘要