Two stages double attention convolutional neural network for crowd counting
MULTIMEDIA TOOLS AND APPLICATIONS(2020)
摘要
Crowd counting has captured wide attention in computer vision, which aims to accurately count the number of people in still images or video scenes. However, it’s still a challenging task due to the scale variation and cluttered background in crowd scenes. In this paper, we propose a 2-stage Double Attention convolutional neural network for crowd counting, and call it 2-DA-CNN, which could deal with scale variation and cluttered background in crowd counting. The proposed 2-DA-CNN includes three parts. The first part is the front-end module which consists of a set of convolution operations, whose function is to extract abundant feature of crowd. The second part is the first double attention module, which contains trunk branch and mask branch. The former is mainly composed by multi-column CNN module, which is to deal with scale variation in crowd scenes. The latter can generate two masks, which aims to assign interesting regions reasonably in cluttered situation. The third part is the second double attention module, similar to the first double attention module, which can enhance the performance of multi-column CNN module further. In addition, we propose progressive training method to improve the drawback of using geometry-adaptive kernels to generate ground truth. The experimental results on three mainstream datasets (ShanghaiTech part B, ShanghaiTech part A and UCF_CC_50) suggest that the proposed 2-DA-CNN is competitive with the state-of-the-art methods.
更多查看译文
关键词
Crowd counting,Convolutional neural network,Double attention,Progressive training
AI 理解论文
溯源树
样例
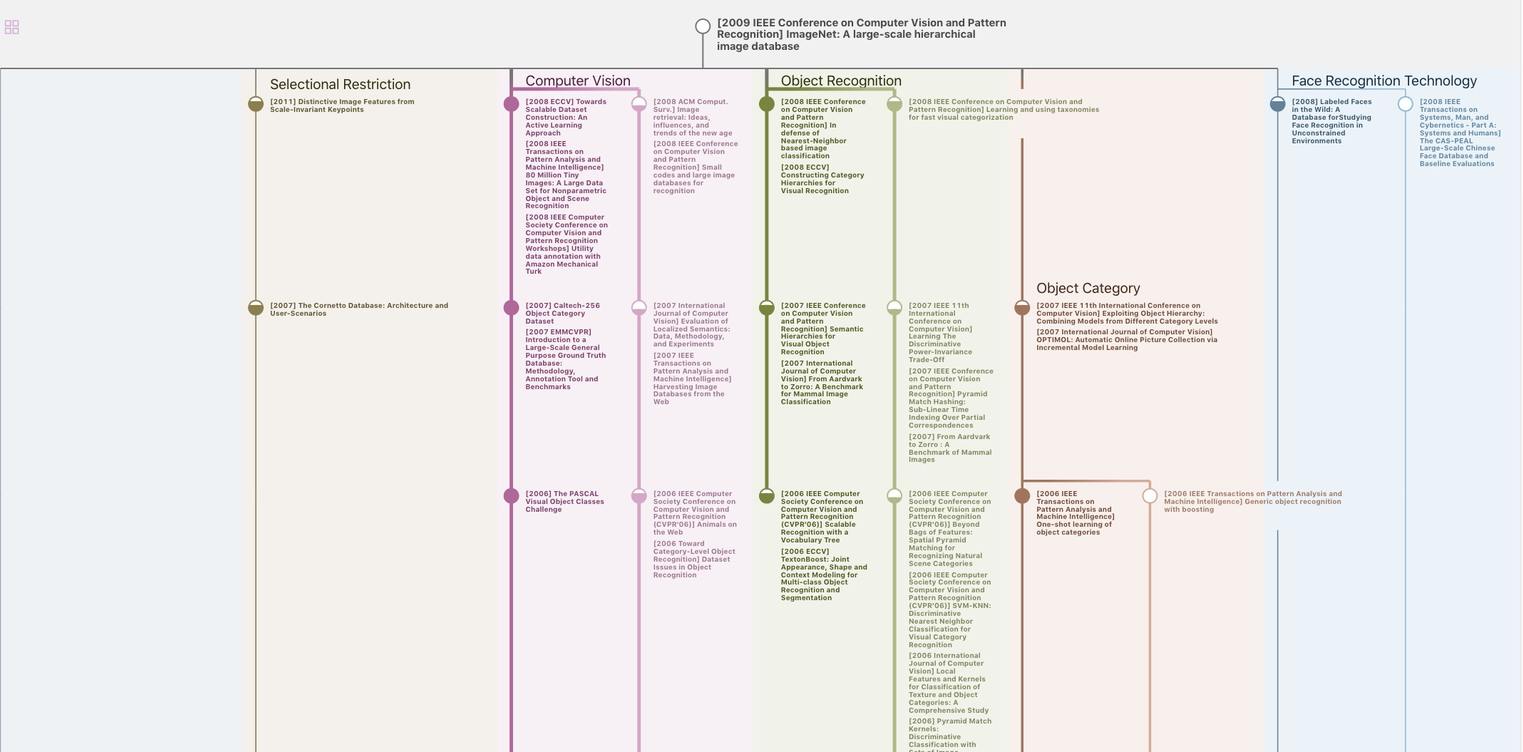
生成溯源树,研究论文发展脉络
Chat Paper
正在生成论文摘要