A 4.45 ms Low-Latency 3D Point-Cloud-Based Neural Network Processor for Hand Pose Estimation in Immersive Wearable Devices
2020 IEEE Symposium on VLSI Circuits(2020)
摘要
A 3D point-cloud-based neural network (PNN) processor is proposed for the low-latency hand pose estimation (HPE) system. The processor adopts the heterogeneous core architecture to accelerate both convolution layers (CLs) and sampling-grouping layers (SGLs). The proposed window-based sampling-grouping (WSG) directly samples and groups the 3D points from the streaming depth image to boost up the throughput by ×2.34. Furthermore, the max pooling prediction (MPP) predicts the 64- and 128-to-1 max pooling outputs with ×1.31 throughput enhancement. In addition, the tiled data based MPP (TMPP) performs the MPP with the tiled input data to hide the latency of the MPP. As a result, the processor achieves 4.45 ms latency on the HPE system.
更多查看译文
关键词
immersive wearable devices,point-cloud-based,low-latency hand,estimation system,heterogeneous core architecture,convolution layers,sampling-grouping layers,max pooling prediction,MPP,128-to-1 max pooling,HPE system,hand pose estimation,ms low-latency 3D,time 4.45 ms
AI 理解论文
溯源树
样例
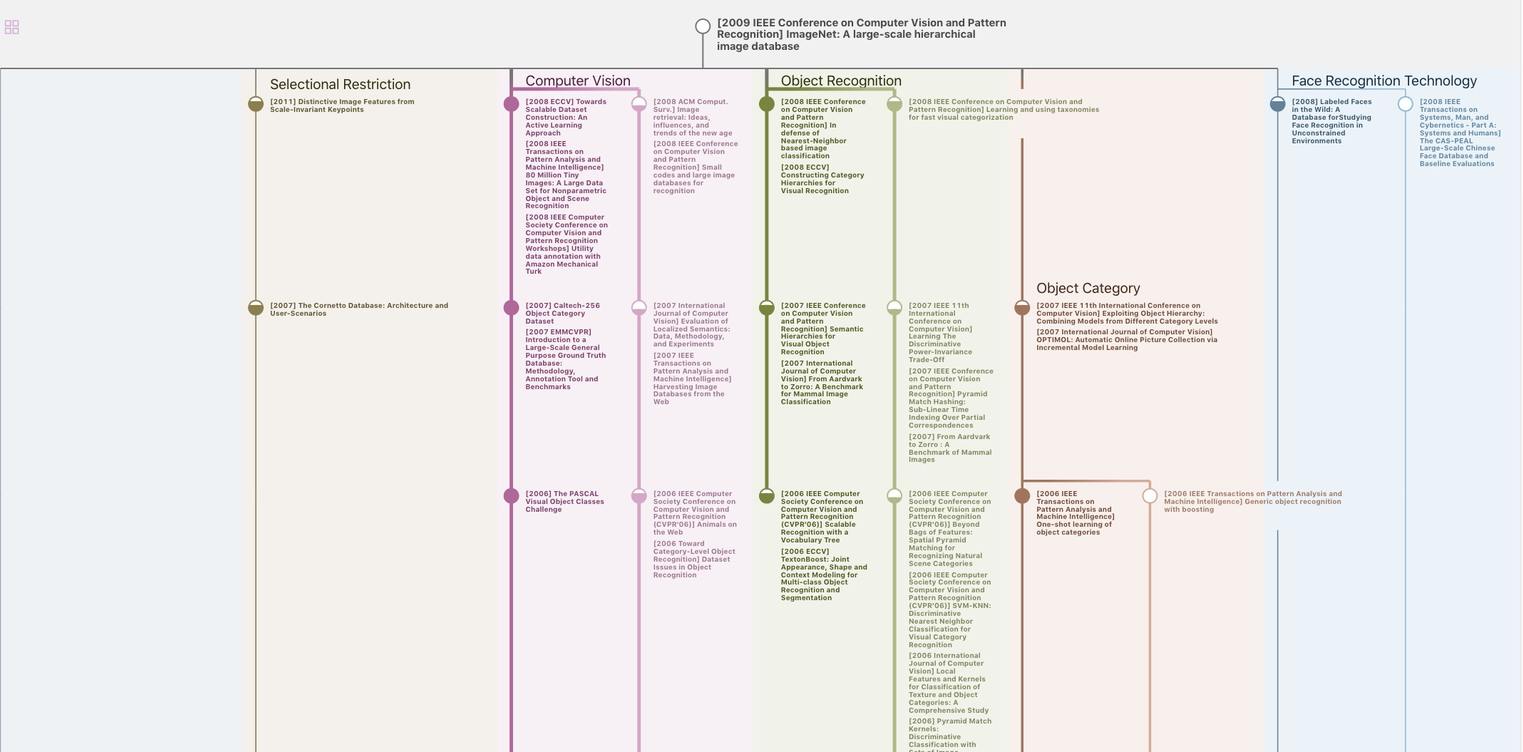
生成溯源树,研究论文发展脉络
Chat Paper
正在生成论文摘要