Poster Abstract: Model Average-Based Distributed Training For Sparse Deep Neural Networks
IEEE INFOCOM 2020 - IEEE CONFERENCE ON COMPUTER COMMUNICATIONS WORKSHOPS (INFOCOM WKSHPS)(2020)
摘要
Distributed training of large-scale deep neural networks(DNNs) is a challenging work for it's time costing and complicated communication. Existing works have achieved scalable performance on GPU clusters for dense DNNs in the computer vision area. However, little progress has been made on the distributed training of sparse DNNs which is commonly used in the area of natural language processing (NLP). In this poster, we introduce SA-HMA, a sparsity -aware hybrid training method for sparse deep models. SA-HMA combines Model Average (MA) and synchronous optimization methods together, expecting to reduce the communication cost for spare model training. The experimental results show that SA-HMA achieves 133x speedup over the state-of-the-art work.
更多查看译文
关键词
sparse DNNs,SA-HMA,sparsity-aware hybrid training method,synchronous optimization methods,sparse deep neural networks,model average-based distributed training
AI 理解论文
溯源树
样例
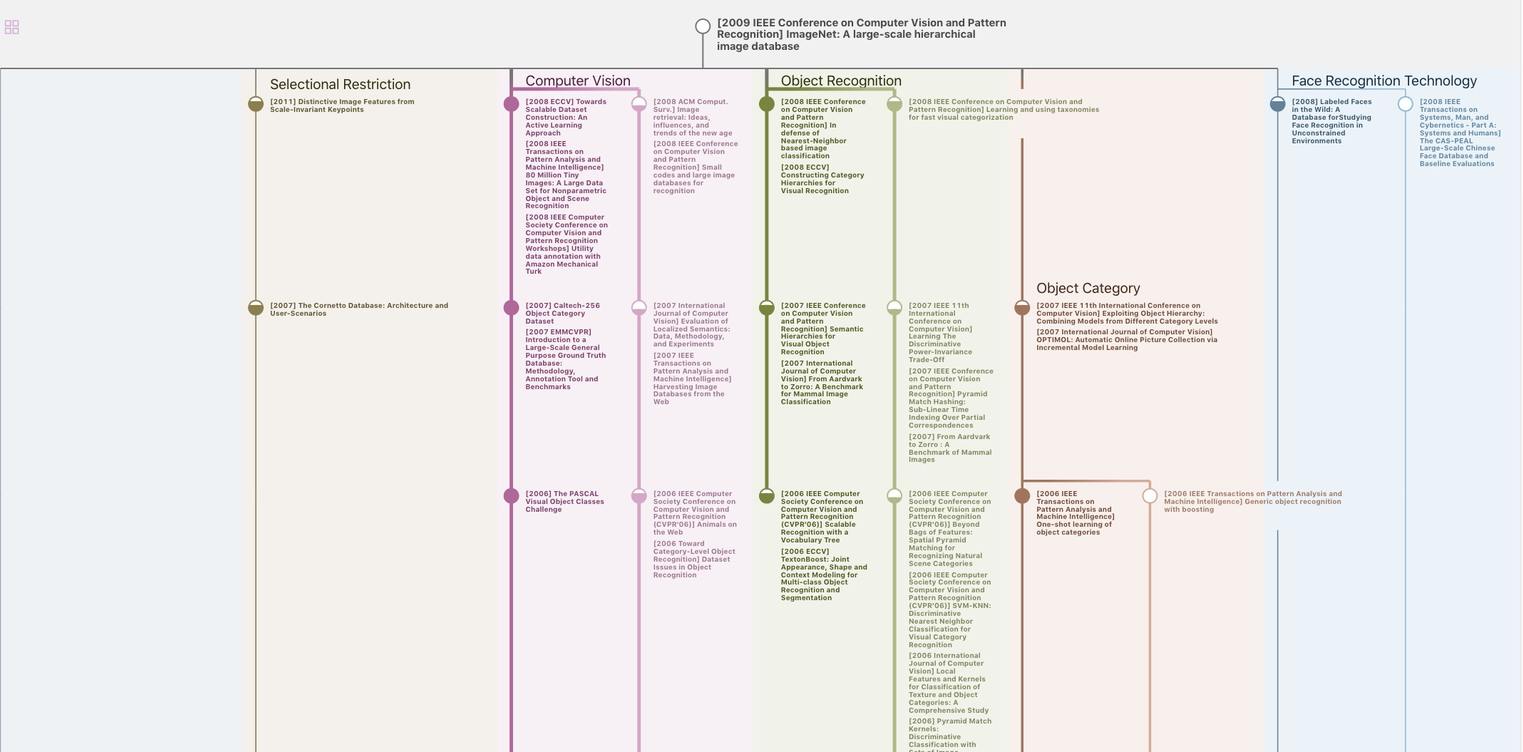
生成溯源树,研究论文发展脉络
Chat Paper
正在生成论文摘要