Robust Self-Sparse Fuzzy Clustering for Image Segmentation
IEEE ACCESS(2020)
摘要
Traditional fuzzy clustering algorithms suffer from two problems in image segmentations. One is that these algorithms are sensitive to outliers due to the non-sparsity of fuzzy memberships. The other is that these algorithms often cause image over-segmentation due to the loss of image local spatial information. To address these issues, we propose a robust self-sparse fuzzy clustering algorithm (RSSFCA) for image segmentation. The proposed RSSFCA makes two contributions. The first concerns a regularization under Gaussian metric that is integrated into the objective function of fuzzy clustering algorithms to obtain fuzzy membership with sparsity, which reduces a proportion of noisy features and improves clustering results. The second concerns a connected-component filtering based on area density balance strategy (CCF-ADB) that is proposed to address the problem of image over-segmentation. Compared to the integration of local spatial information into the objective functions, the presented CCF-ADB is simpler and faster for the removal of small areas. Experimental results show that the proposed RSSFCA addresses two problems in current fuzzy clustering algorithms, i.e., the outlier sensitivity and the over-segmentation, and it provides better image segmentation results than state-of-the-art algorithms.
更多查看译文
关键词
Clustering algorithms,Image segmentation,Linear programming,Robustness,Microsoft Windows,Computational efficiency,Noise measurement,Fuzzy c-means clustering (FCM),image segmentation,sparse membership,over-segmentation
AI 理解论文
溯源树
样例
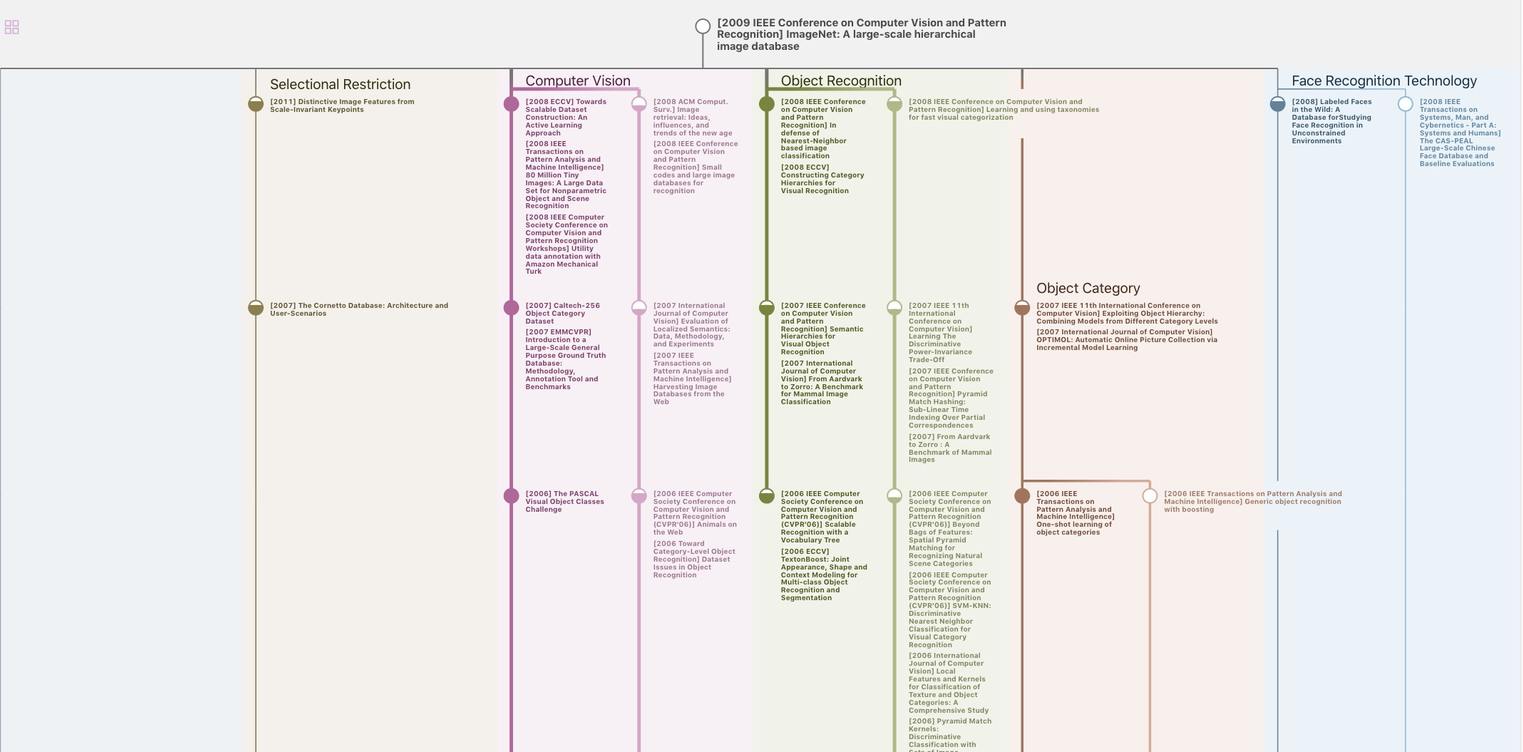
生成溯源树,研究论文发展脉络
Chat Paper
正在生成论文摘要