Glide And Zap Q-Learning
IEEE INFOCOM 2020 - IEEE CONFERENCE ON COMPUTER COMMUNICATIONS WORKSHOPS (INFOCOM WKSHPS)(2020)
摘要
As a powerful mathematical framework that allows intelligent agents to gradually learn their optimal strategies in unknown dynamic environments, reinforcement learning (RL) has found its success in many important applications. Nonetheless, a common stumbling block of RI, algorithms is their low learning speed. Although different methods have been developed in literature to enhance the learning speed when special structure or prior learning experience is available, expediting RL in the general settings still remains a challenge. The Zap Q-learning is a recent breakthrough in this direction, which is shown to be an order of magnitude faster than the conventional Q-learning and its cutting-edging variants. Inspired by this exciting result, a novel algorithm, termed Glide and Zap Q-learning (G-Zap Q-learning), is proposed in this work by incorporating a novel gliding step into the learning process. The proposed algorithm is provably convergent to the optimal strategy and can further increase the learning speed of the original Zap Q-learning by several folds. In addition, it is applicable to general Markov decision processes (MDPs) and hence assumes wide applications. Simulations over both randomly generated MDPs and an exemplary application of privacy-aware task offloading in mobile-edge computing are conducted to validate the effectiveness of the proposed algorithm.
更多查看译文
关键词
optimal strategy,reinforcement learning,RL algorithms,G-Zap Q-learning,glide and zap Q-learning,general Markov decision process,MDP,privacy-aware task offloading,mobile-edge computing
AI 理解论文
溯源树
样例
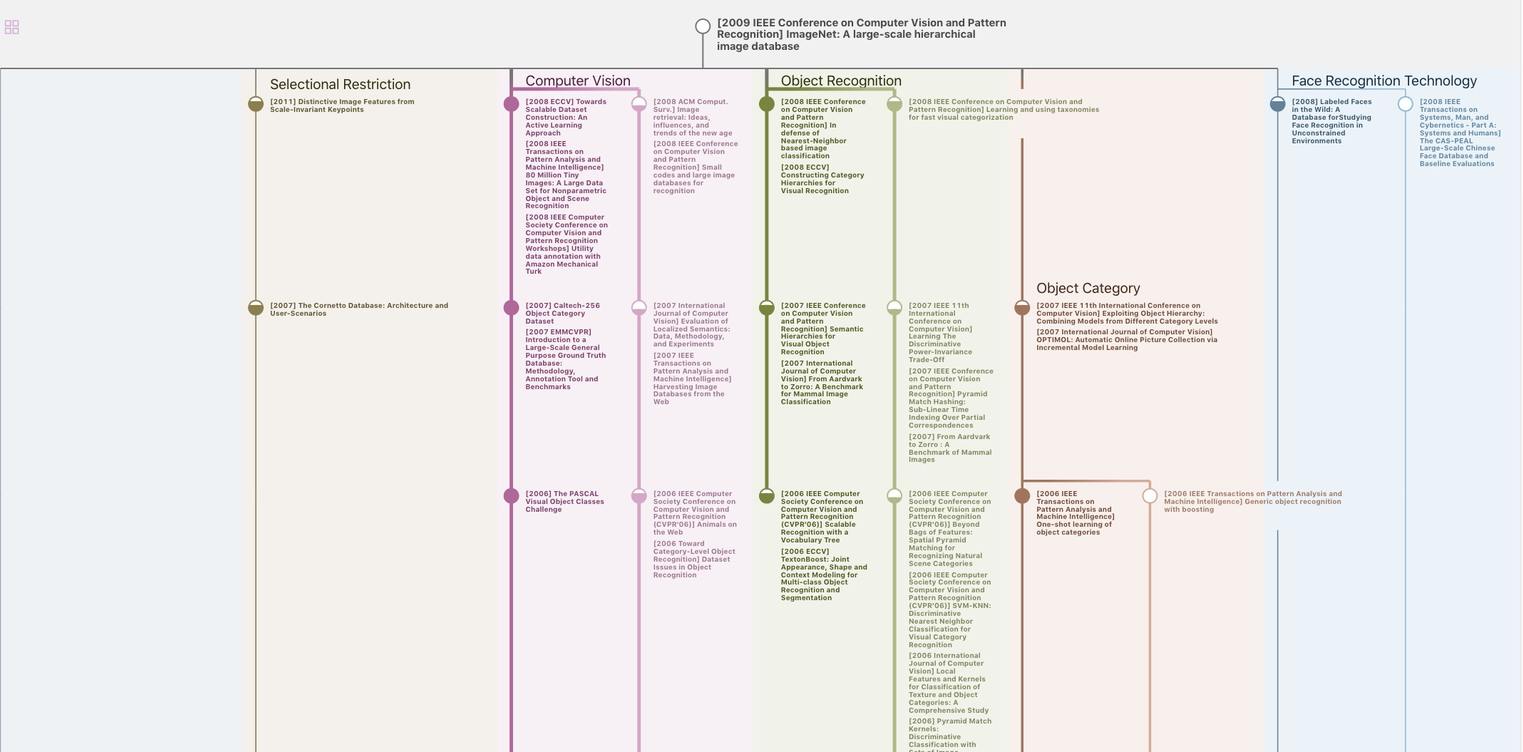
生成溯源树,研究论文发展脉络
Chat Paper
正在生成论文摘要