Research on Human Action Recognition Based on Improved Pooling Algorithm
PROCEEDINGS OF THE 32ND 2020 CHINESE CONTROL AND DECISION CONFERENCE (CCDC 2020)(2020)
摘要
With the rapid development of deep learning, human behavior recognition has become a focus of research. When using various artificial neural network training models to recognize human behavior, the problem of overfitting has always been a difficult point that hinders the improvement of the accuracy of recognition. This paper combines stochastic pooling and Dropout, proposes an improved stochastic pooling method based on Dropout, and applies it to a 3D convolutional neural network for human behavior recognition to solve the problem of overfitting. In the training phase, the Dropout method is introduced into the pooling layer of the 3DCNN, and the unit values of the pooling layer are randomly suppressed, so that more sub-models are generated on the pooling layer. During the testing phase, the idea of double probability weighting method is introduced The idea of combining the weight of the activation value of the selected neuron and the activation value of the selected neuron is introduced to obtain an approximate model of all sub-models. Finally, the trained model was input into the UCF101 data set for verification, and an accuracy rate of 70.09% was obtained. Experimental results show that this method can extract more general features, enhance generalization ability, effectively suppress overfitting, and improve accuracy.
更多查看译文
关键词
Stochastic pooling, Dropout, Model average, 3DCNN
AI 理解论文
溯源树
样例
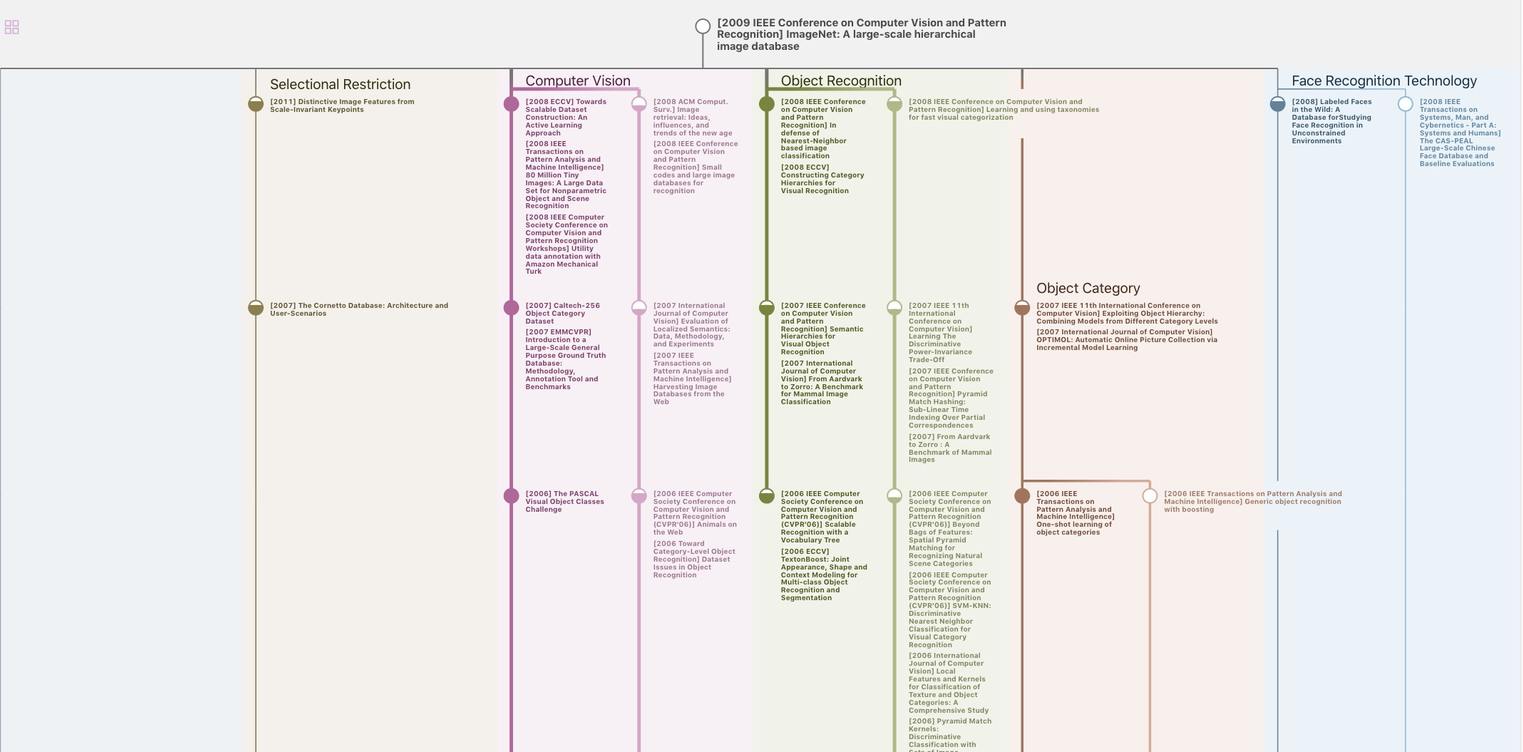
生成溯源树,研究论文发展脉络
Chat Paper
正在生成论文摘要