MG-BERT: A Multi-glosses BERT Model for Word Sense Disambiguation.
KSEM (2)(2020)
摘要
Word Sense Disambiguation (WSD) is a core task in NLP fields and has many potential applications. Traditional supervised methods still have obstacles, such as the problem of variable size of label candidates and the lack of annotated corpora. Although attempts are made to integrate gloss information to the model, no existing models have paid attention to the divergences among glosses. In this paper, we propose a Multi-Glosses BERT (MG-BERT) model with two main advantages for WSD task. Our model jointly encodes the context and multi-glosses of the target word. We show that our Context with Multi-Glosses mechanism can find out and emphasize the divergences among glosses and generate nearly orthogonal gloss embeddings, which makes it more accuracy to match the context with the correct gloss. We design three classification algorithms, Gloss Matrix Classifier (GMC), General Gloss Matrix Classifier (GGMC) and Space Transforming Classifier (STC), all of which can disambiguate words with full-coverage of WordNet. In GMC and GGMC, we utilize gloss embeddings as weight matrix. For STC, we transform different label space to a same label space. Experiment shows that our MG-BERT model achieves new state-of-the-art performance on all WSD benchmarks.
更多查看译文
关键词
word sense disambiguation,mg-bert,multi-glosses
AI 理解论文
溯源树
样例
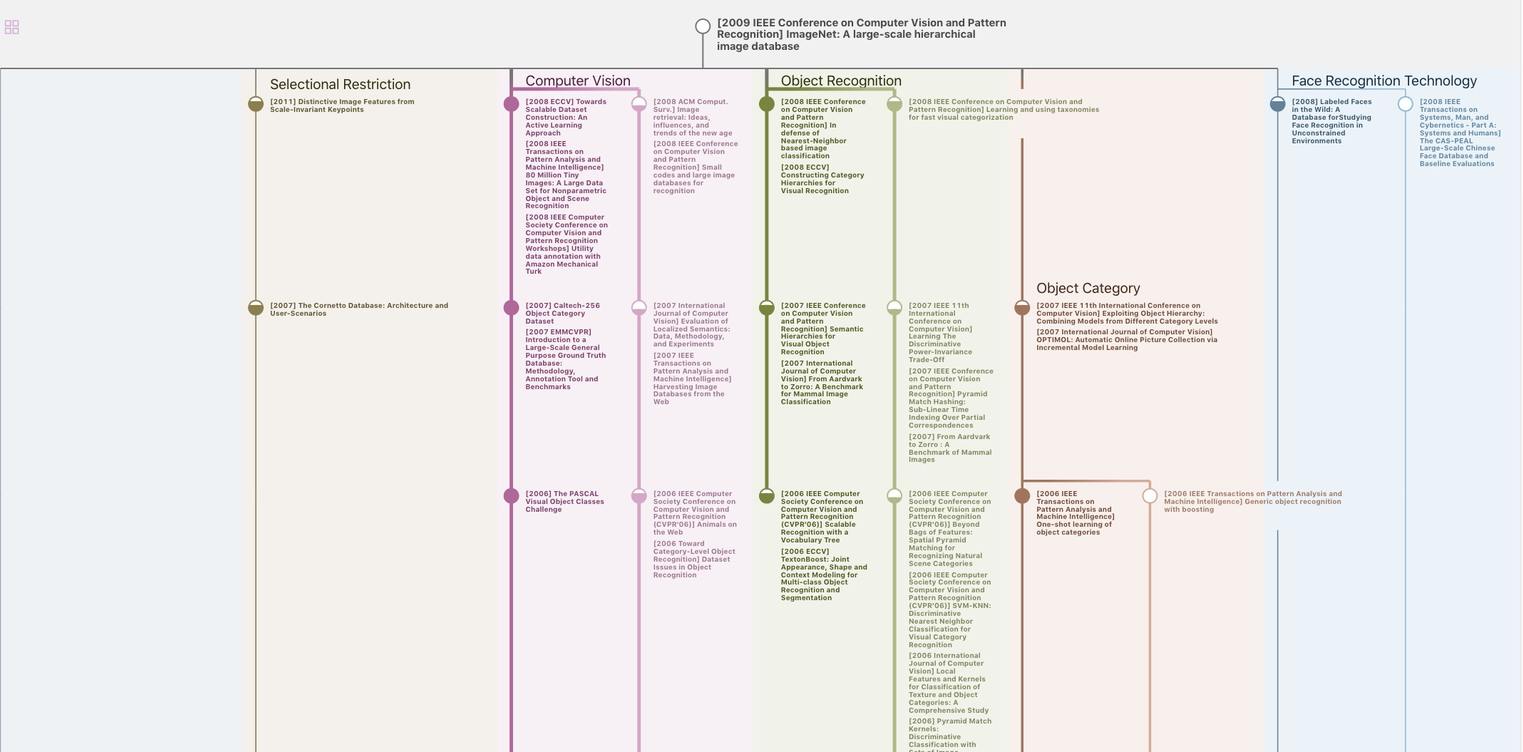
生成溯源树,研究论文发展脉络
Chat Paper
正在生成论文摘要