Extending Sliding-Step Importance Weighting from Supervised Learning to Reinforcement Learning
IJCAI(2019)
摘要
Stochastic gradient descent (SGD) has been in the center of many advances in modern machine learning. SGD processes examples sequentially, updating a weight vector in the direction that would most reduce the loss for that example. In many applications, some examples are more important than others and, to capture this, each example is given a non-negative weight that modulates its impact. Unfortunately, if the importance weights are highly variable they can greatly exacerbate the difficulty of setting the step-size parameter of SGD. To ease this difficulty, Karampatziakis and Langford [6] developed a class of elegant algorithms that are much more robust in the face of highly variable importance weights in supervised learning. In this paper we extend their idea, which we call “sliding step”, to reinforcement learning, where importance weighting can be particularly variable due to the importance sampling involved in off-policy learning algorithms. We compare two alternative ways of doing the extension in the linear function approximation setting, then introduce specific sliding-step versions of the TD(0) and Emphatic TD(0) learning algorithms. We prove the convergence of our algorithms and demonstrate their effectiveness on both on-policy and off-policy problems. Overall, our new algorithms appear to be effective in bringing the robustness of the sliding-step technique from supervised learning to reinforcement learning.
更多查看译文
关键词
Reinforcement learning,Temporal difference learning,Off-policy
AI 理解论文
溯源树
样例
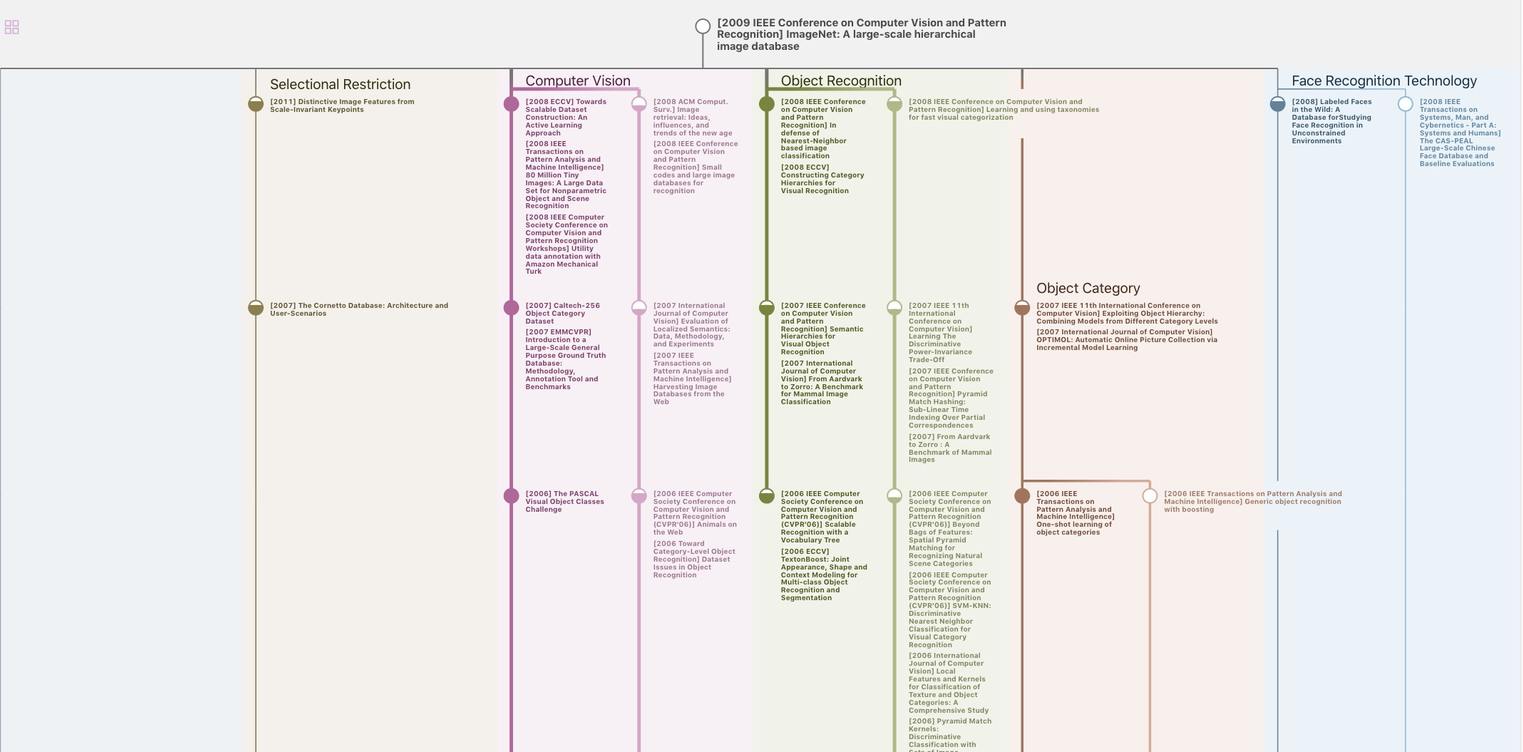
生成溯源树,研究论文发展脉络
Chat Paper
正在生成论文摘要