Iterative Learning Control For Nonlinear Multi-Agent Systems With Initial Shifts
IEEE ACCESS(2020)
Abstract
In this paper, a discussion is made on the consensus tracking control by iterative learning method for high-order nonlinear multi-agent systems. Among them, all agents with initial state errors are enabled to perform a given repetitive task over a finite interval. The method proposed can achieve consensus tracking through a series of initial shifts correction actions. In the process of tracking, this algorithm rectifies the initial error of the state x(n) of each agent at first, then the error of x(n-1), and so on. All of these rectifying actions are finished in a specified interval. Furthermore, the algorithm has shown effective in the improvement of tracking performance through simulation.
MoreTranslated text
Key words
Convergence,Iterative learning control,Trajectory,Licenses,Law enforcement,Robustness,Task analysis,Multi-agent systems,iterative learning control,convergence,step-by-step correction
AI Read Science
Must-Reading Tree
Example
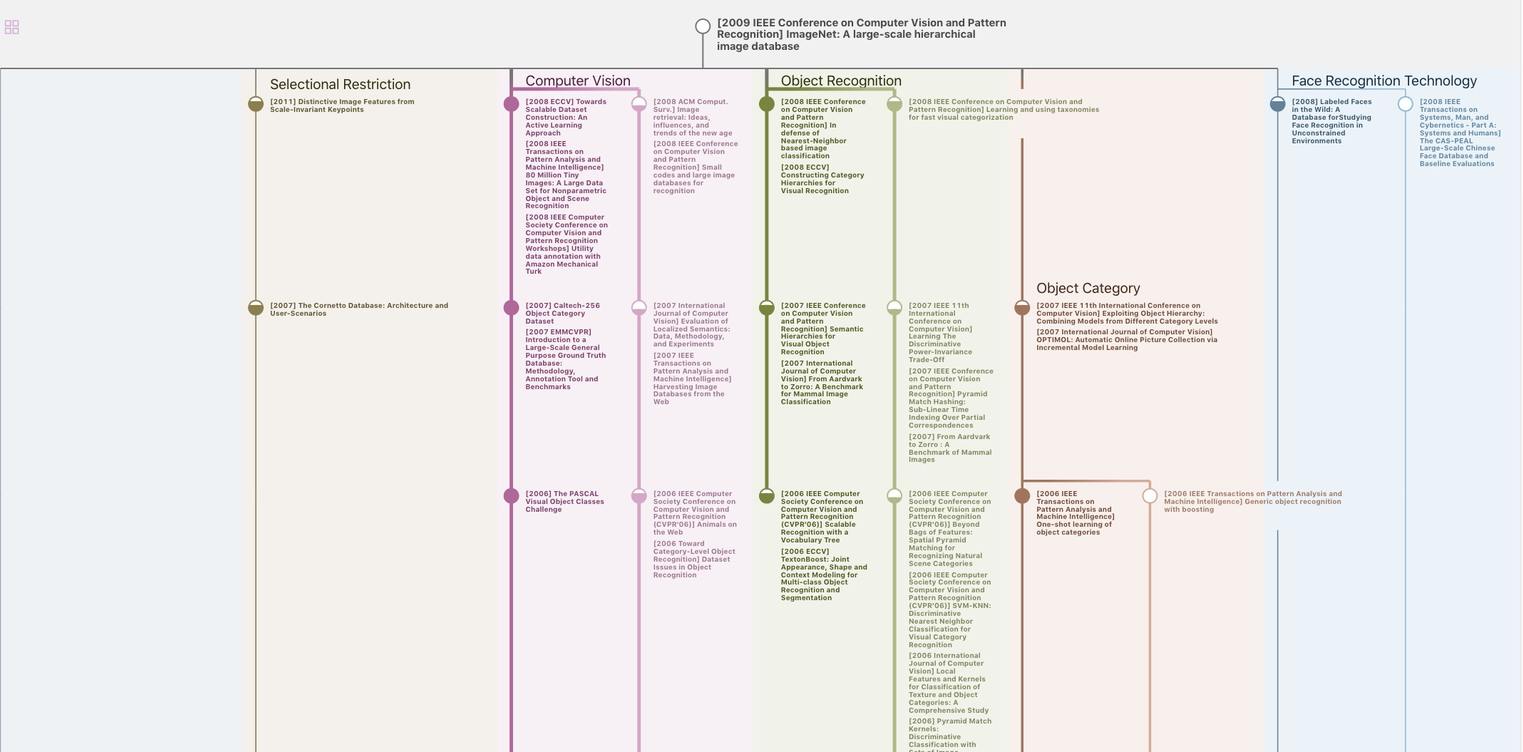
Generate MRT to find the research sequence of this paper
Chat Paper
Summary is being generated by the instructions you defined