Develop Load Shape Dictionary Through Efficient Clustering Based on Elastic Dissimilarity Measure
IEEE Transactions on Smart Grid(2021)
摘要
Load shape dictionary (LSD) is a useful tool for utilizing the enormous amount of smart meter data to understand customers' electricity consumption behaviors. To tackle the big data challenge as well as to better capture the load shape features, this article develops a bilevel LSD generation framework to cluster and index the residential load profiles into a neat local LSD and a global LSD based on the Derivative Dynamic Time Warping (DDTW) elastic dissimilarity measure. Different from the classic Dynamic Time Warping (DTW), DDTW works on the derivative of the raw data to avoid DTW's problem of pathological alignments. To reduce the computational cost, a fast DDTW (FDDTW) is proposed to speed up the DDTW calculation. Based on the generated bilevel LSD, analytic approaches are proposed to extract features from the data indexed by the LSD to reveal useful information of customers' electricity consumption behaviors. Numerical experiments on real premise data verify the effectiveness of the proposed methodology in terms of clustering performance and computational efficiency. Our analysis suggests that the proposed methodology can be applied to improve load forecasting, tariff design and demand response (DR) customer targeting.
更多查看译文
关键词
Clustering,smart meter data,load profile,derivative dynamic time warping (DDTW),elastic dissimilarity measure,computational efficiency
AI 理解论文
溯源树
样例
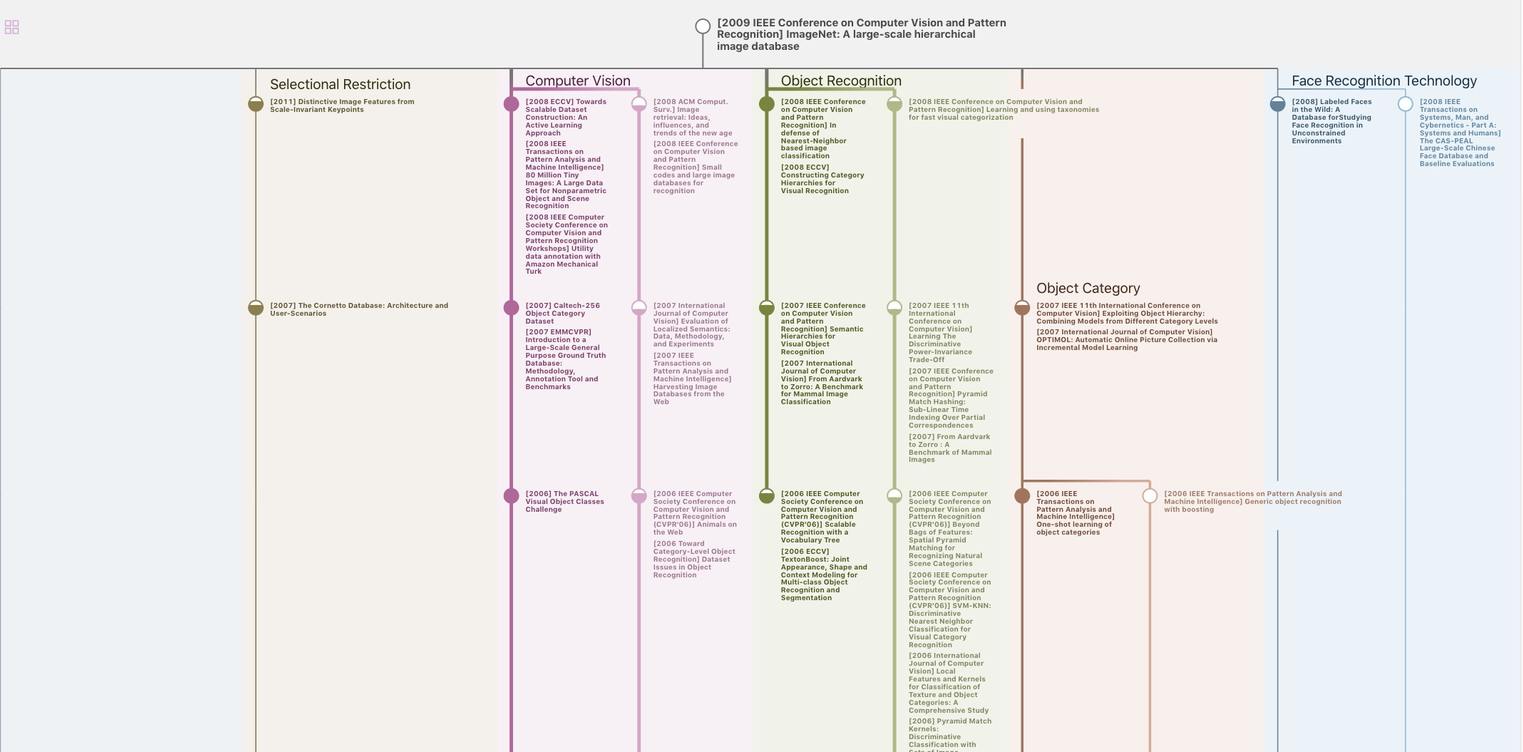
生成溯源树,研究论文发展脉络
Chat Paper
正在生成论文摘要