Generalized sparse filtering for rotating machinery fault diagnosis
The Journal of Supercomputing(2020)
摘要
This paper develops generalized sparse filtering (GSF) by applying general norm normalization to improve the feature learning ability. A rotating machinery fault diagnosis method is then developed by combining the GSF and softmax regression. A rolling bearing dataset is applied to validate the performance of the developed method. The influences of normalization parameters on the diagnostic performance are investigated in detail, and thus, the best parameter combinations are determined based on the diagnostic accuracy and computing time. A planetary gearbox dataset is also applied to further validate the diagnostic performance on rotating machinery. Finally, the mechanism of the GSF is explained using a simple example. The results show that the GSF has a more powerful feature learning capacity than standard sparse filtering, and the developed method can obtain excellent diagnostic performance. Two variants of the developed method are recommended for the rotating machinery fault diagnosis.
更多查看译文
关键词
Intelligent fault diagnosis, Sparse filtering, Unsupervised feature learning, Rotating machinery
AI 理解论文
溯源树
样例
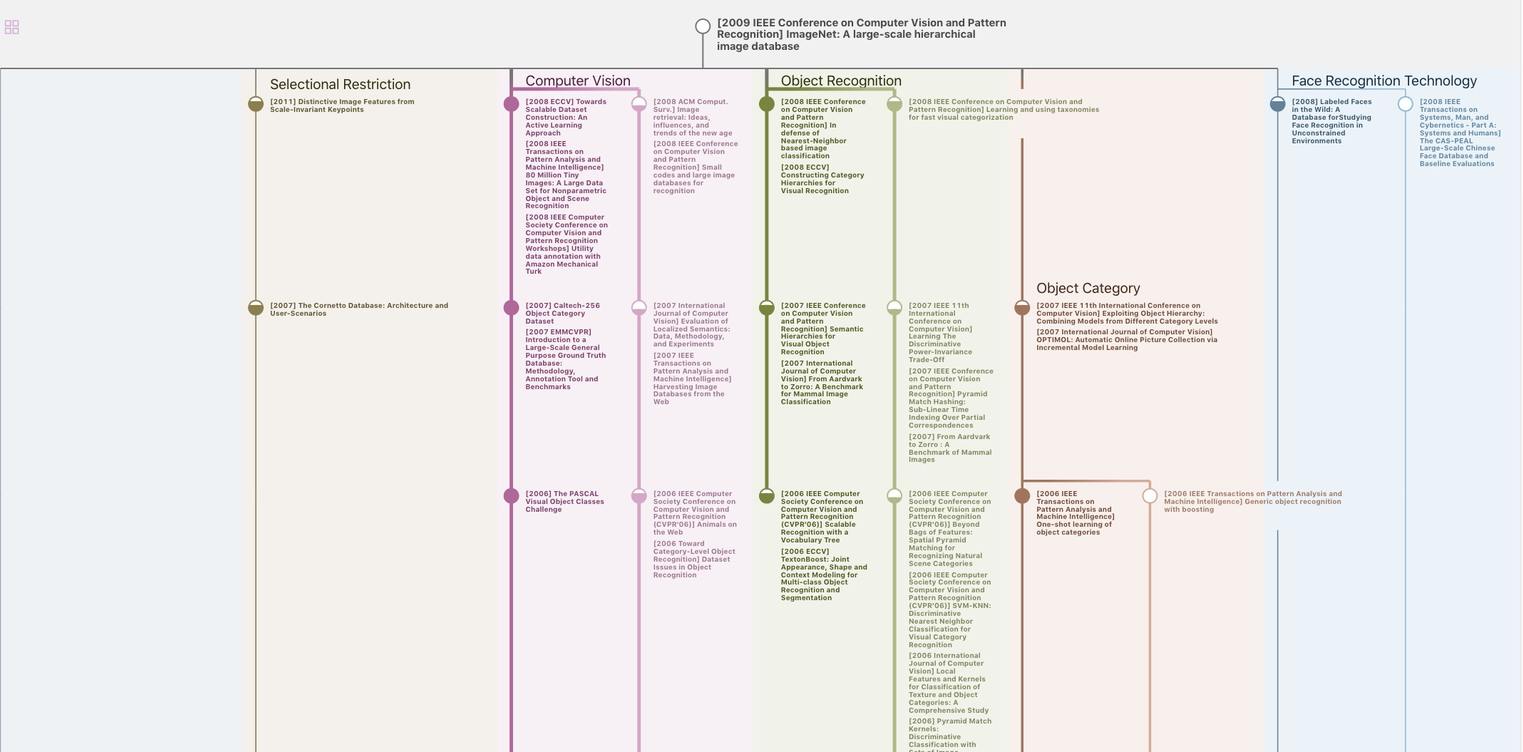
生成溯源树,研究论文发展脉络
Chat Paper
正在生成论文摘要