GDCRN: Global Diffusion Convolutional Residual Network for Traffic Flow Prediction.
KSEM (2)(2020)
摘要
Traffic flow prediction is a crucial issue for intelligent transportation system. Because of complicated topological structures of road networks and dynamic spatial-temporal patterns of traffic conditions, predicting flows on the road networks is still a challenging task. Most existing approaches focus on the local spatial-temporal correlations, ignoring the global spatial dependences and the global dynamic spatial-temporal correlations. In this paper, we propose a novel deep learning model for traffic flow prediction, called Global Diffusion Convolution Residual Network (GDCRN), which consists of multiple periodic branches with the same structure. Each branch applies global graph convolution layer to capture both local and global spatial dependencies, and further apply GRes to describe global spatial-temporal correlations simultaneously. Extensive experiments on two real-world datasets demonstrate that our model can capture both the global and local spatial-temporal dependencies dynamically. The experimental results show the effectiveness of our method.
更多查看译文
关键词
Traffic prediction, Spatial-temporal network, Graph convolution network
AI 理解论文
溯源树
样例
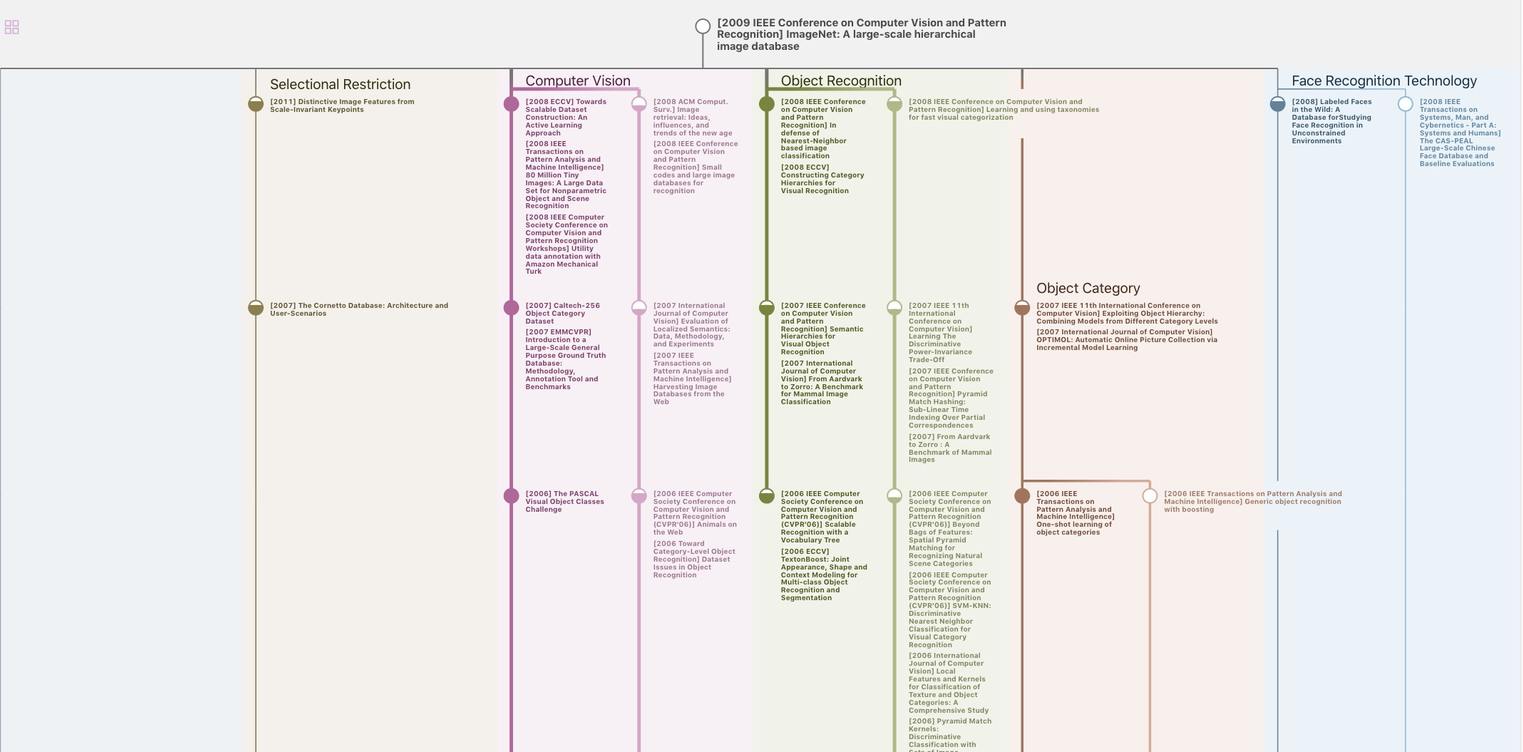
生成溯源树,研究论文发展脉络
Chat Paper
正在生成论文摘要