Learned Scheduling of LDPC Decoders Based on Multi-armed Bandits
ISIT(2020)
摘要
The multi-armed bandit (MAB) problem refers to the dilemma encountered by a gambler when deciding which arm of a multi-armed slot machine to pull in order to maximize the total reward earned in a sequence of pulls. In this paper, we model the scheduling of a node-wise sequential LDPC decoder as a Markov decision process, where the underlying Tanner graph is viewed as a slot machine with multiple arms corresponding to the check nodes. A fictitious gambler decides which check node to pull (schedule) next by observing a reward associated with each pull. This interaction enables the gambler to discover an optimized scheduling policy that aims to reach a codeword output by propagating the fewest possible messages. Based on this policy, we contrive a novel MAB-based node-wise scheduling (MABNS) algorithm to perform sequential decoding of LDPC codes. Simulation results show that the MAB-NS scheme, aided by an appropriate scheduling policy, outperforms traditional scheduling schemes in terms of complexity and bit error probability.
更多查看译文
关键词
Markov decision process,multiarmed bandits,LDPC decoder learned scheduling,multi-armed slot machine,node-wise sequential LDPC decoder scheduling,Tanner graph,codeword,MAB-based node-wise scheduling algorithm,MABNS algorithm,LDPC code sequential decoding,bit error probability
AI 理解论文
溯源树
样例
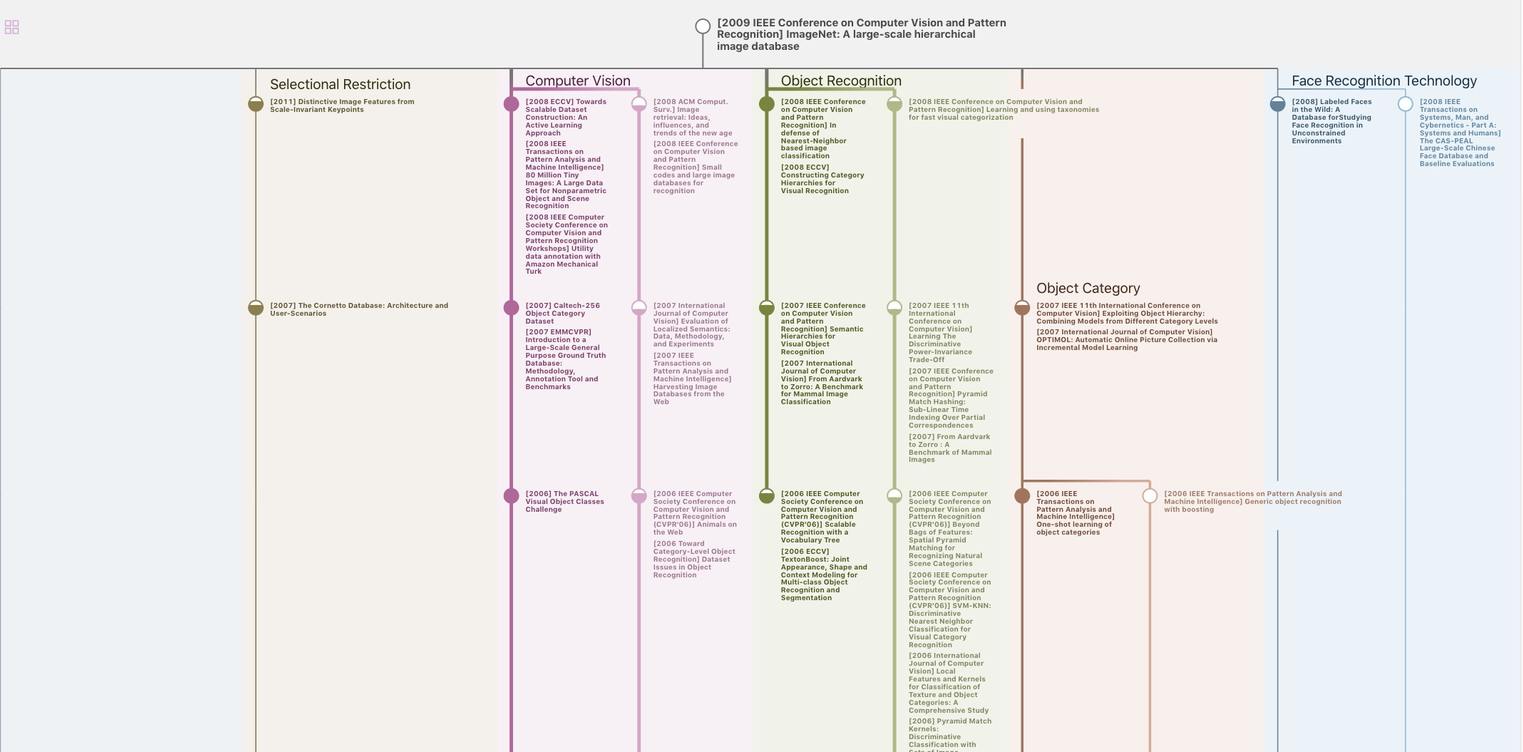
生成溯源树,研究论文发展脉络
Chat Paper
正在生成论文摘要