Taxi Demand Prediction Using Parallel Multi-Task Learning Model
IEEE Transactions on Intelligent Transportation Systems(2022)
摘要
Accurate and real-time taxi demand prediction can help managers pre-allocate taxi resources in cities, which assists drivers quickly finding passengers and reduce passengers’ waiting time. Most of the existing studies focus on mining spatial-temporal characteristics of taxi demand distributions, while lacking in modeling the correlations between taxi pick-up demand and the drop-off demand from the perspective of multi-task learning. In this article, we propose a multi-task learning model containing three parallel LSTM layers to co-predict taxi pick-up and drop-off demands, and compare the performance of single demand prediction methodology and that of two demands’ co-prediction methodology. Experimental results on real-world datasets demonstrate that the pick-up demand and the drop-off demand do depend on each other, and the effectiveness of the proposed co-prediction methods.
更多查看译文
关键词
Taxi demand prediction,pick-up/drop-off demand,multi-task learning,LSTM,deep learning
AI 理解论文
溯源树
样例
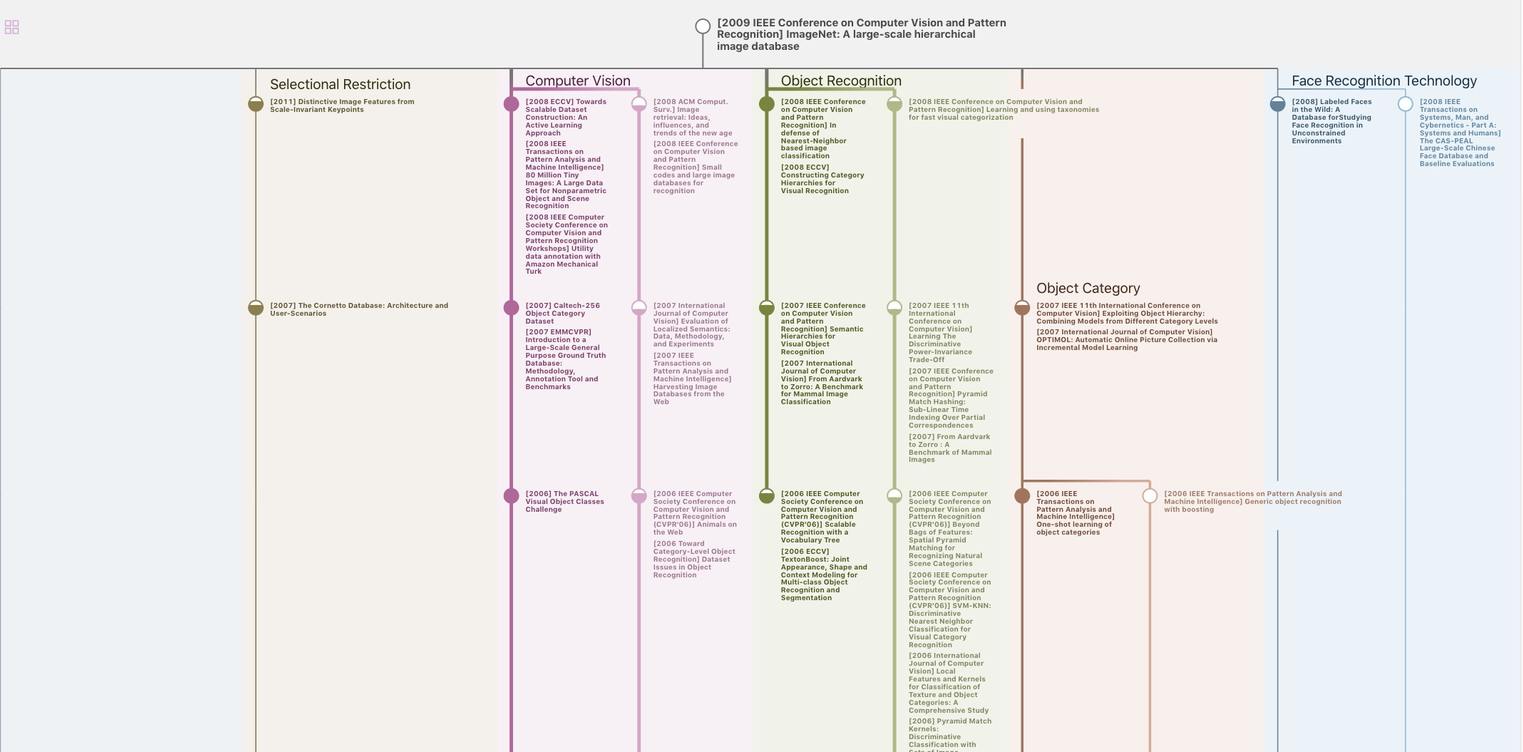
生成溯源树,研究论文发展脉络
Chat Paper
正在生成论文摘要