On the Impact of Perceptual Compression on Deep Learning
2020 IEEE Conference on Multimedia Information Processing and Retrieval (MIPR)(2020)
摘要
This paper proposes a fundamental answer to a frequently asked question in multimedia evaluation and data set creation: Do artifacts from perceptual compression contribute to error in the machine learning process and if so, how much? Our approach to the problem is an information reinterpretation of the Helmholtz free energy formula to explain the relationship between content and noise when using sensors (such as cameras or microphones) to capture multimedia data. The reinterpretation guides a bit-measurement of the noise contained in images, audio, and video by combining a classifier with perceptual compression, such as JPEG or MP3. Our experiments on CIFAR-10, ImageNet, and CSAIL Places as well as Fraunhofer's IDMT-SMT-Audio-Effects dataset indicate that, at the right quality level, perceptual compression is actually not harmful but contributes to a significant reduction of complexity of the machine learning process. That is, our noise quantification method can be used to speed up the training of deep learning classifiers significantly while maintaining, or sometimes even improving, overall classification accuracy.
更多查看译文
关键词
Neural Networks,Deep learning,Images,Audio,Compression,datasets,Information
AI 理解论文
溯源树
样例
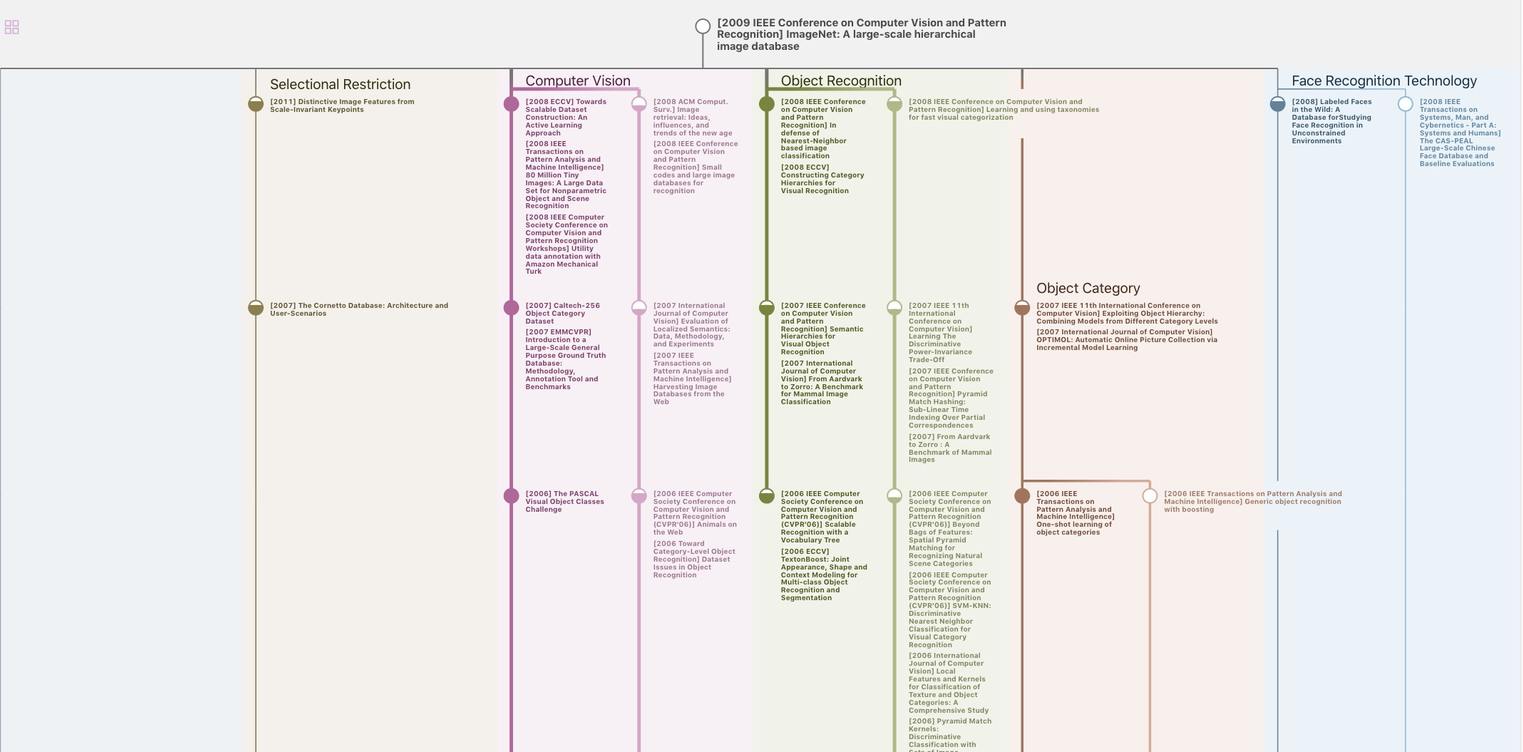
生成溯源树,研究论文发展脉络
Chat Paper
正在生成论文摘要