A Unified Labeling Model for Open-Domain Aspect-Based Sentiment Analysis
DSC(2020)
摘要
Aspect-based sentiment analysis involves aspect term extraction and sentiment prediction towards aspect terms. Recently, more researchers have proposed integrated approaches to accomplish two tasks simultaneously. However, such approaches always limit the domain, quantity, length and category of aspect terms, which greatly restricts its use. This paper aims to model the joint task as an extension of sequence labeling and presents a novel unified labeling model that supports a wide range of aspect terms. Unlike a conventional tagging scheme that predicts the boundary of an aspect term and classifies its sentiment step by step, our proposed model deals with two tasks simultaneously through one set of labels. Sentiment polarities are labeled directly on aspect term tokens, thus combining the boundary information with sentiment polarity in this unified tagging scheme. In this paper, we take Bidirectional Encoder Representations from Transformer (BERT) as the first representation layer to capture contextual features of the entire sentence. Conditional Random Field (CRF) follows BERT for minimizing empirical risk and labeling each token representation within given label sets based on the learned transition matrix. In our experiments, the proposed method demonstrates superior performance against multiple baselines on three benchmark datasets and one Twitter datasets collected by ourselves containing open-domain sentences and aspect terms with various categories, lengths and quantities.
更多查看译文
关键词
aspect-based sentiment analysis,sequence labeling,deep learning
AI 理解论文
溯源树
样例
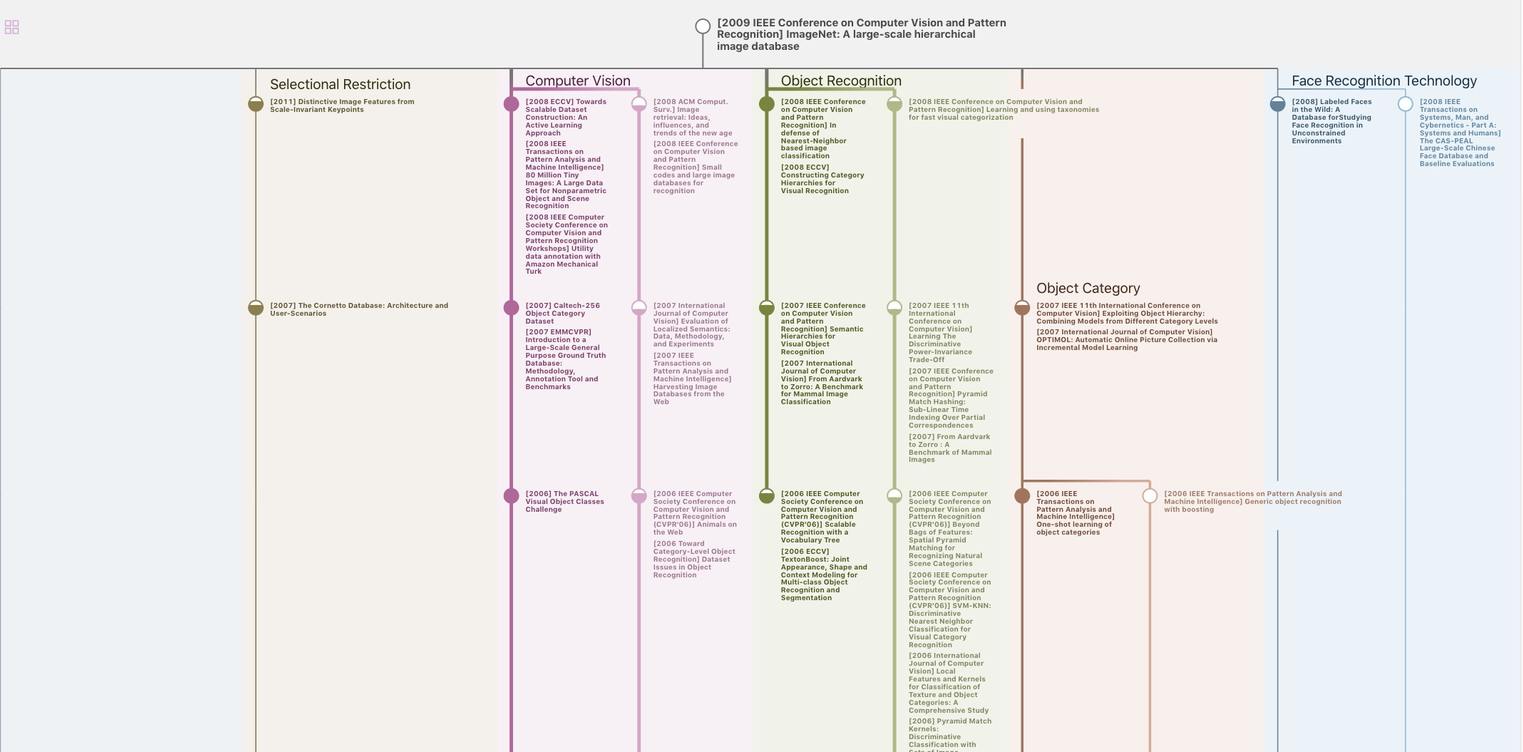
生成溯源树,研究论文发展脉络
Chat Paper
正在生成论文摘要