Explaining Misclassification and Attacks in Deep Learning via Random Forests.
MDAI(2020)
摘要
Artificial intelligence, and machine learning (ML) in particular, is being used for different purposes that are critical for human life. To avoid an algorithm-based authoritarian society, AI-based decisions should generate trust by being explainable. Explainability is not only a moral requirement, but also a legal requirement stated in the European General Data Protection Regulation (GDPR). Additionally, it is also beneficial for researchers and practitioners relying on AI methods, who need to know whether the decisions made by the algorithms they use are rational, lack bias and have not been subjected to learning attacks. To achieve AI explainability, it must be possible to derive explanations in a systematic and automatic way. A common approach is to use a simpler, more understandable decision algorithm to build a surrogate model of the unexplainable, a.k.a. black-box model (typically a deep learning algorithm). To this end, we should avoid surrogate models that are too large to be understood by humans. In this work we focus on explaining the behavior of black-box models by using random forests containing a fixed number of decision trees of limited depth as surrogates. In particular, our aim is to determine the causes underlying misclassification by the black box model. Our approach is to leverage partial decision trees in the forest to calculate the importance of the features involved in the wrong decisions. We achieve great accuracy in detecting and explaining misclassification by deep learning models constructed via federated learning that have suffered attacks.
更多查看译文
关键词
Explainability, Machine learning, Deep learning, Random forest
AI 理解论文
溯源树
样例
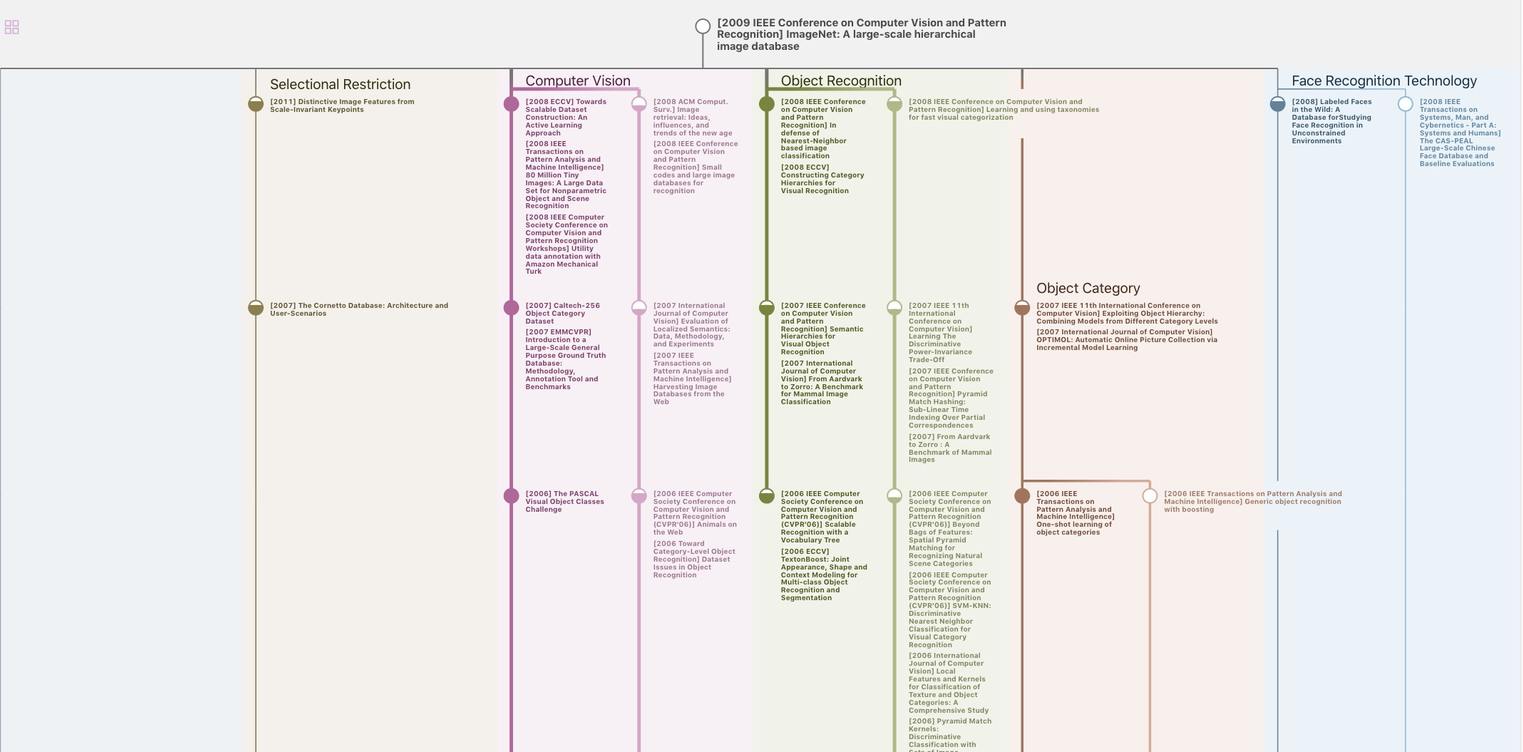
生成溯源树,研究论文发展脉络
Chat Paper
正在生成论文摘要