Community Detection Based On Modularized Deep Nonnegative Matrix Factorization
INTERNATIONAL JOURNAL OF PATTERN RECOGNITION AND ARTIFICIAL INTELLIGENCE(2021)
摘要
Community detection is a well-established problem and nontrivial task in complex network analysis. The goal of community detection is to discover community structures in complex networks. In recent years, many existing works have been proposed to handle this task, particularly nonnegative matrix factorization-based method, e.g. HNMF, BNMF, which is interpretable and can learn latent features of complex data. These methods usually decompose the original matrix into two matrixes, in one matrix, each column corresponds to a representation of community and each column of another matrix indicates the membership between overall pairs of communities and nodes. Then they discover the community by updating the two matrices iteratively and learn the shallow feature of the community. However, these methods either ignore the topological structure characteristics of the community or ignore the microscopic community structure properties. In this paper, we propose a novel model, named Modularized Deep NonNegative Matrix Factorization (MDNMF) for community detection, which preserves both the topology information and the instinct community structure properties of the community. The experimental results show that our proposed models can significantly outperform state-of-the-art approaches on several well-known dataset.
更多查看译文
关键词
Community detection, nonnegative matrix factorization, modularity, deep matrix factorization
AI 理解论文
溯源树
样例
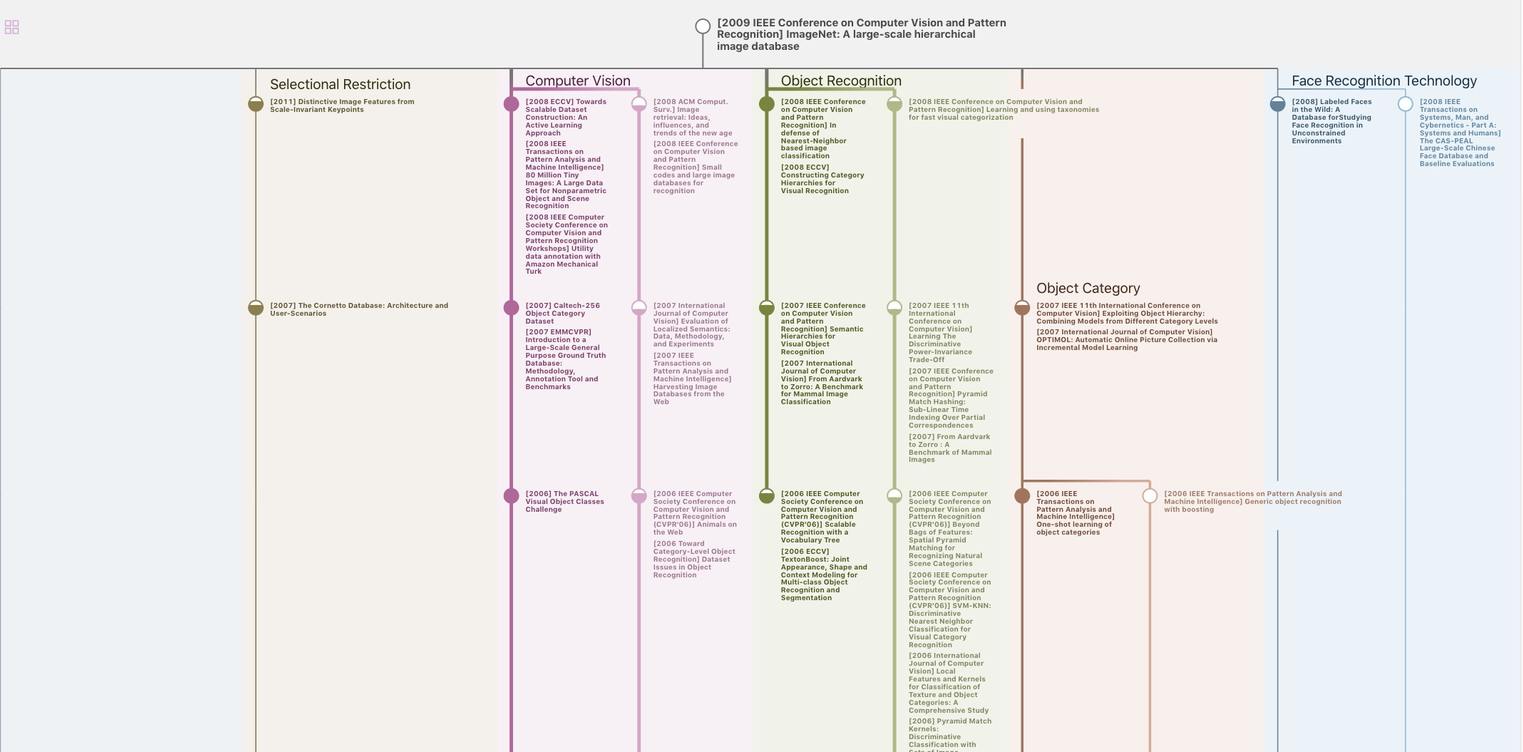
生成溯源树,研究论文发展脉络
Chat Paper
正在生成论文摘要