End-Edge-Cloud Collaborative System: A Video Big Data Processing and Analysis Architecture
2020 IEEE Conference on Multimedia Information Processing and Retrieval (MIPR)(2020)
摘要
In the current surveillance system, video streams are firstly captured and compressed at the cameras, and then transmitted to the backend severs or cloud for big data analysis. It is impractical to aggregate all video streams from hundreds of thousands of cameras for big data analysis. Transcoding the videos to low-bitrate ones is the conventional solution to solve the aggregation bottleneck. However, it is recognized that transcoding will inevitably affect visual feature extraction, consequently degrading the subsequent analysis performance. To address these challenges, we thus propose a new video big data analysis framework, called end-edge-cloud collaborative system. Under the end-edge-cloud collaborative framework, a camera can output two streams simultaneously, including a compressed video stream for viewing and data storage, and a compact feature stream extracted from the original video signals for visual analysis. Video stream and feature stream are synchronized by unified identification. We identify three key technologies to enable the end-edge-cloud collaborative system, including analysis-friendly video coding, visual feature compact descriptor, and user-defined neural network and parameter updating. By real-time feeding only the feature streams into the cloud center, these cameras thus form a large-scale brain-like vision system for the smart city. A prototype has been implemented to demonstrate its feasibility. Experiment results show that our system can achieve high efficient video compression and guarantee the analysis performance. Furthermore, our system makes the big data analysis feasible which only need aggregate low bit-rate compressed feature stream.
更多查看译文
关键词
City Brain,Analysis-friendly Video Coding,Compact Feature Representation,Data Aggregation,Collaborative
AI 理解论文
溯源树
样例
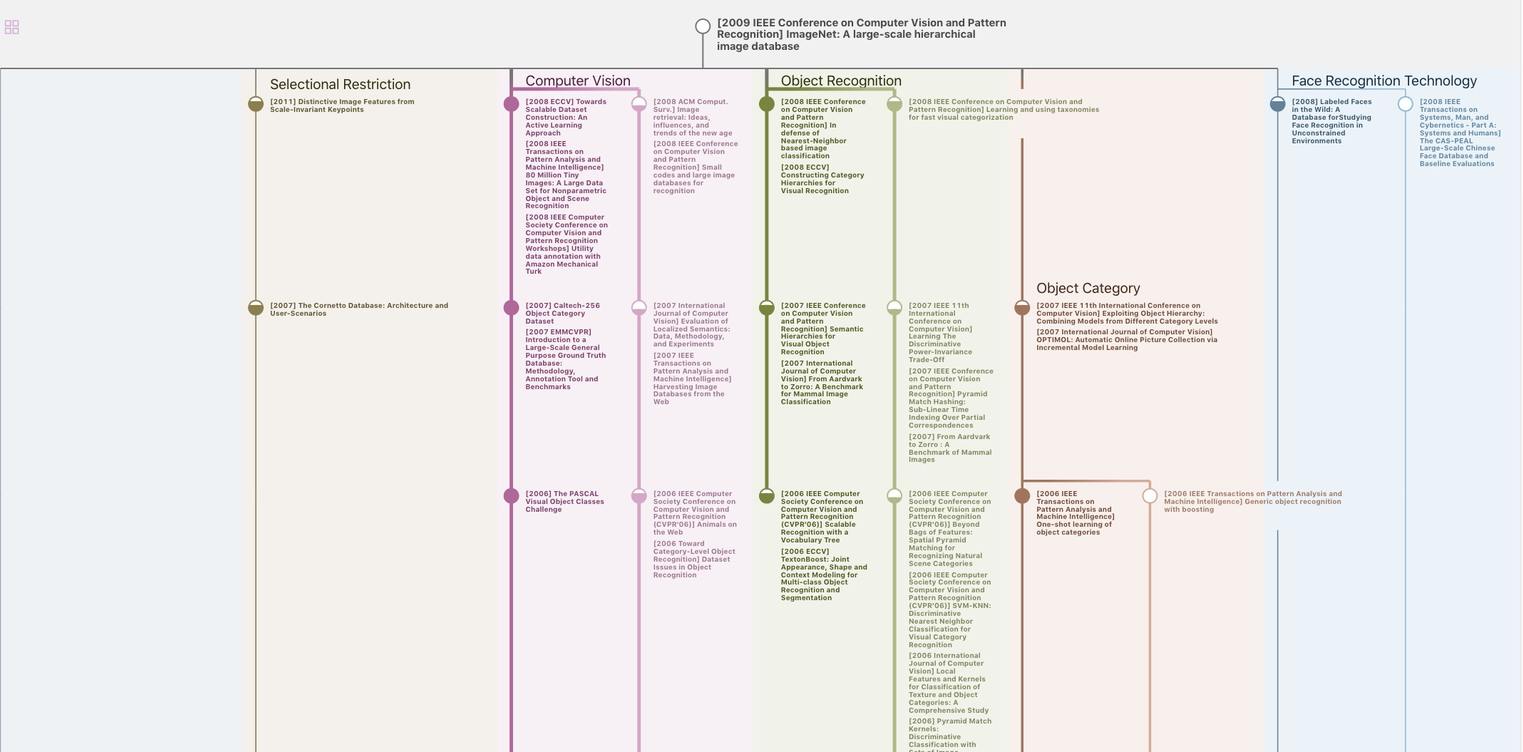
生成溯源树,研究论文发展脉络
Chat Paper
正在生成论文摘要