Mapping Pm2.5 Concentration At High Resolution Using A Cascade Random Forest Based Downscaling Model: Evaluation And Application
JOURNAL OF CLEANER PRODUCTION(2020)
摘要
Fine particulate matter (PM2.5) mapping at high resolution (sub-kilometers level) can be important for fine-scale pollution research. However, current researches about high-resolution PM2.5 mapping mostly depend on high-resolution aerosol optical depth product and can be difficult to be applied to a large extent. In this paper, we tried to solve the problem of large-scale high-resolution PM2.5 mapping through a machine learning based downscaling approach. We developed the cascade random forest model and utilized the elevation and land cover data as auxiliary variables for downscaling. The results showed that the proposed downscaling method can improve the resolution of the current PM2.5 product by more than 30 times from 0.1 degrees (similar to 10 km) to 0.003 degrees (similar to 300m), with the product accuracy well kept. The correlation between ground-based PM2.5 measurements and the original and downscaled products were 0.74 and 0.76, respectively. Using the proposed downscaling method, we completed 3 aspects of work. Firstly, we selected seven typical cities in China to analyze the spatial variations of PM2.5 at 0.003 degrees. Secondly, the PM2.5 annual variations from 2000 to 2015 in Wuhan were analyzed at a fine scale. The impact of the nature reserve and urbanization on PM2.5 pollution was detected. And finally, we applied this method to the Korean Peninsula and North Indian to see whether it has the potential to be applied to a global extent. The proposed downscaling algorithm is an effective method with low requirements for input data and a significant improvement in product resolution, it has a large potential to be applied to the generation of global high-resolution PM2.5 product. (C) 2020 Elsevier Ltd. All rights reserved.
更多查看译文
关键词
PM2.5, Downscale, High-resolution, Cascade random forest, Urban expansion
AI 理解论文
溯源树
样例
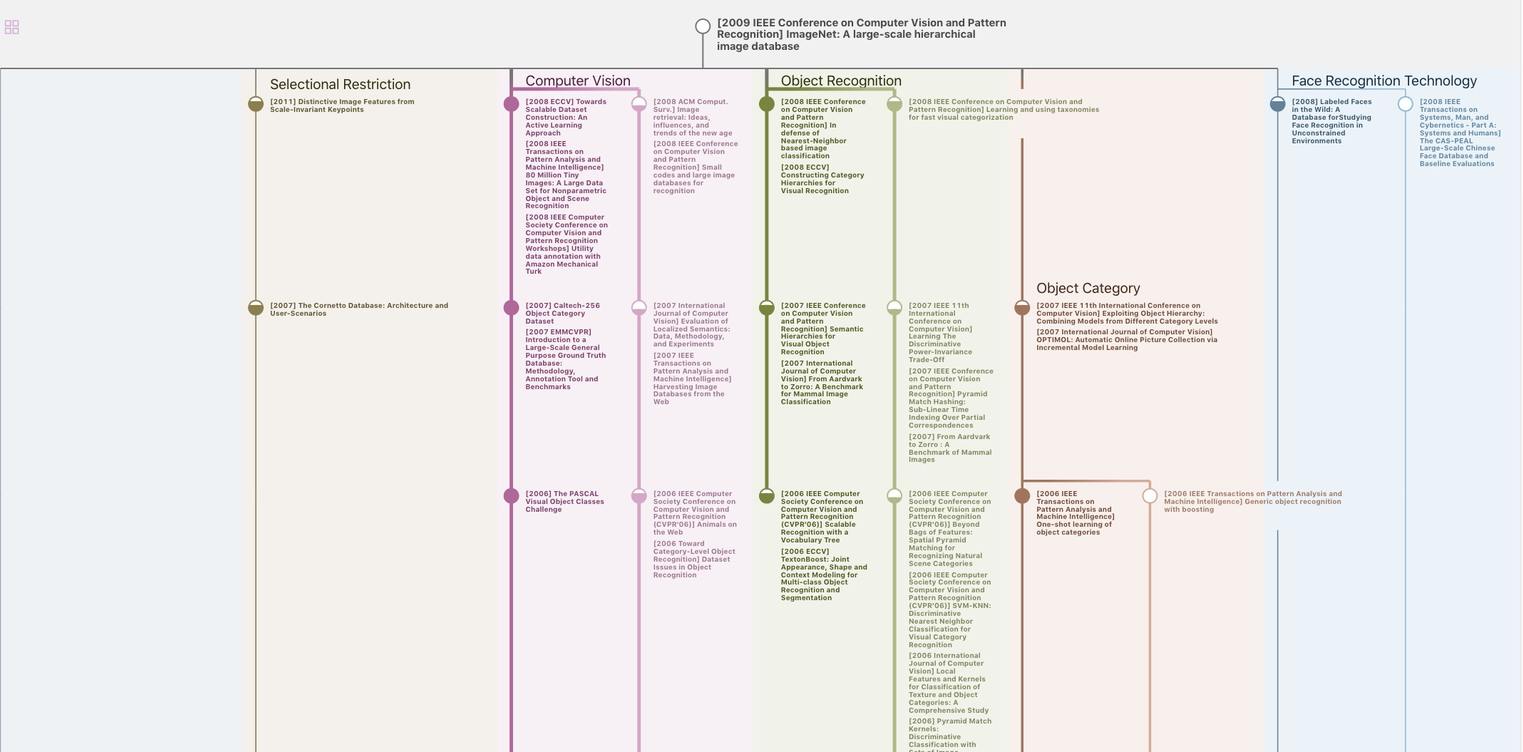
生成溯源树,研究论文发展脉络
Chat Paper
正在生成论文摘要